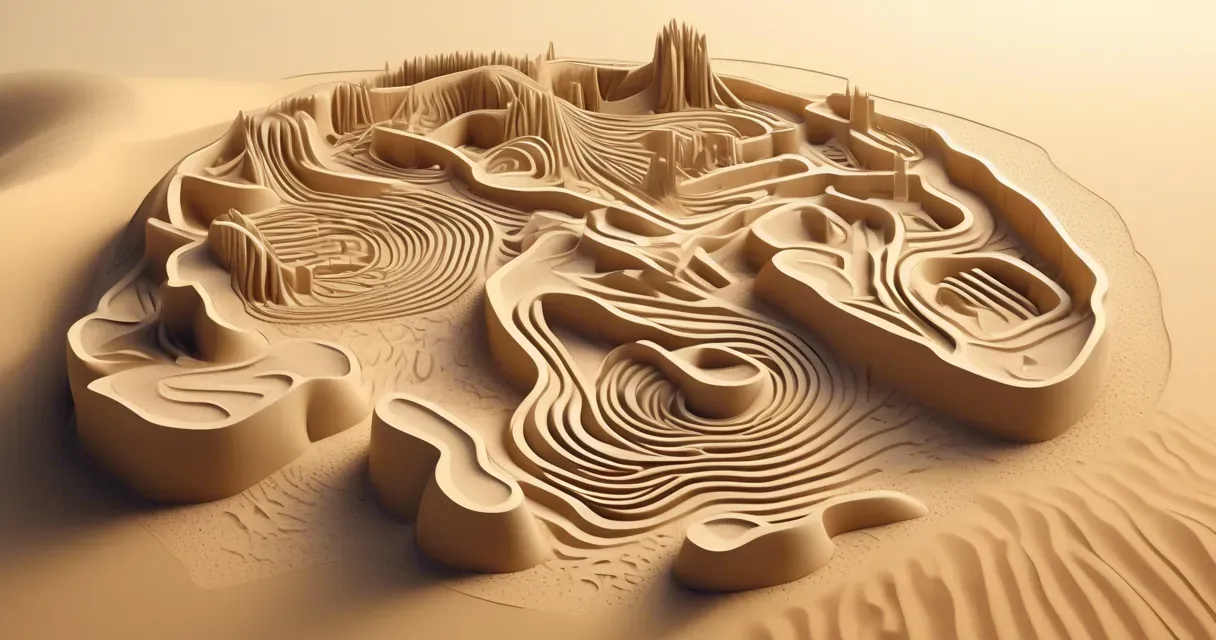
TRIGS Methodology Enhances Trojan Detection in AI Models
/ 4 min read
Quick take - Recent research has demonstrated the effectiveness of the TRIGS methodology in enhancing cybersecurity for machine learning models by improving the detection of Trojan attacks and addressing model integrity risks.
Fast Facts
-
TRIGS Methodology: The TRojan Identification from Gradient-based Signatures (TRIGS) method effectively detects Trojan attacks in machine learning models, enhancing security and model integrity.
-
Key Techniques: The research emphasizes activation optimization for signature creation, feature extraction, and training a CNN classifier to identify malicious patterns.
-
Diverse Evaluation: TRIGS was evaluated across various datasets, demonstrating its robustness and effectiveness in identifying threats.
-
Implications for Cybersecurity: The findings highlight the need for improved detection mechanisms, awareness of model integrity risks, and the evolution of AI governance and compliance frameworks.
-
Future Applications: Promising applications of TRIGS include integration into deployment pipelines, real-time monitoring, and enhancing model resilience against adversarial attacks.
Enhancing Cybersecurity in Machine Learning Models with TRIGS Methodology
In the rapidly evolving landscape of artificial intelligence, ensuring the security and integrity of machine learning models has become paramount. Recent research has introduced the TRIGS (TRojan Identification from Gradient-based Signatures) methodology, a promising approach to detecting Trojan attacks that threaten these systems. This study not only highlights the vulnerabilities inherent in current AI models but also underscores the pressing need for improved detection mechanisms and their implications on AI governance and compliance.
Key Findings and Methodology
The research delves into several critical areas to bolster the security of machine learning models against Trojan attacks. Central to this is the activation optimization for signature creation, which refines how signatures are generated to effectively identify compromised models. This process is complemented by feature extraction, which enhances detection accuracy by isolating relevant data characteristics.
A convolutional neural network (CNN) classifier plays a pivotal role in this methodology. It is trained to recognize patterns indicative of Trojan attacks, thereby providing a robust defense mechanism. The TRIGS method’s effectiveness was rigorously evaluated across diverse datasets, ensuring its applicability in various scenarios and reinforcing its robustness.
The findings reveal that TRIGS excels in identifying malicious models, understanding threat models, and developing strategies to counteract data poisoning and integrity breaches. This positions TRIGS as a vital tool in the cybersecurity arsenal for machine learning systems.
Implications for Cybersecurity
The implications of this research are far-reaching:
-
Increased Awareness of Model Integrity Risks: As Trojan attacks grow more sophisticated, there is heightened awareness among developers and organizations about the vulnerabilities of machine learning models.
-
Need for Enhanced Detection Mechanisms: The study emphasizes an urgent need for more robust detection systems to safeguard against malicious interventions.
-
Implications for Model Training Practices: The findings suggest revisiting current model training practices to incorporate security measures that can preemptively address potential threats.
-
Broader Impact on AI Governance and Compliance: The research indicates that compliance frameworks must evolve to tackle new security challenges posed by advanced AI technologies.
Strengths and Limitations
The TRIGS methodology showcases several strengths, notably its ability to enhance detection mechanisms suitable for diverse architectures and real-time monitoring systems. However, it also has limitations, such as the need for further exploration into its adaptability across different domains and potential unforeseen vulnerabilities in model designs.
Recommended Tools and Techniques
Several tools and techniques are highlighted as essential in understanding and mitigating backdoor attacks:
- TRIGS Methodology: Central to the research, offering a structured framework for Trojan identification.
- Activation Optimization and Feature Extraction: Key processes that improve detection efficacy.
- CNN Classifier: A crucial component in training processes aimed at identifying malicious patterns.
Future Directions and Applications of TRIGS
Looking forward, there are numerous promising applications for TRIGS within cybersecurity:
-
Integration into Model Deployment Pipelines: Ensuring security measures are embedded throughout development and deployment phases.
-
Real-time Monitoring and Anomaly Detection: Utilizing TRIGS for continuous oversight of model behavior in live environments.
-
Training Robustness and Adversarial Defense: Enhancing model resilience against adversarial attacks through refined training techniques.
-
Cross-Domain Applications: Expanding TRIGS’ application across various fields to standardize security practices in machine learning.
As the field continues to evolve, ongoing research will be crucial in refining these methodologies and ensuring that AI applications remain secure against emerging threats.