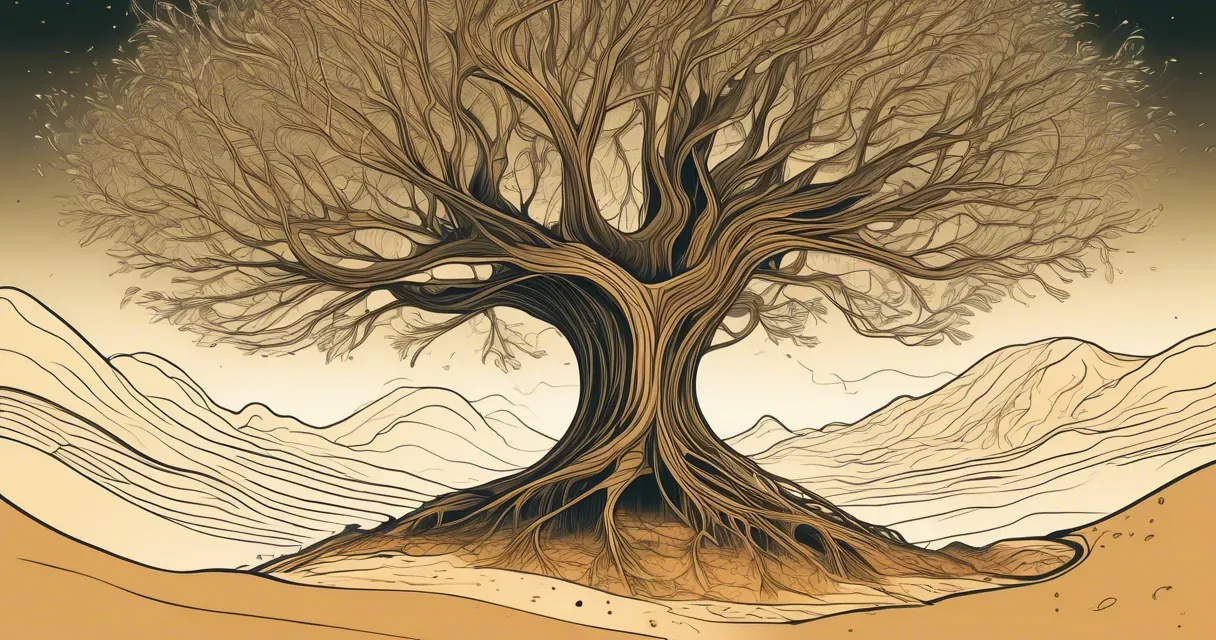
Post-Training Defense Method Enhances Malware Classifiers
/ 4 min read
Quick take - Recent research by Dung Thuy Nguyen and colleagues has introduced the Post-Backdoor Purification method, which aims to improve the security and reliability of machine learning models against backdoor attacks, particularly in malware detection, with potential applications across various cybersecurity domains.
Fast Facts
- Introduction of PBP Method: The Post-Backdoor Purification (PBP) method enhances the security of malware classifiers against backdoor attacks, improving machine learning model reliability.
- Key Objectives: The research focuses on neuron mask generation, activation-shift fine-tuning, and statistical alignment of batch normalization layers to mitigate backdoor influences.
- Promising Outcomes: PBP significantly strengthens defenses against backdoor attacks, providing insights into model behavior and neuron activity, with potential applications beyond malware detection.
- Cybersecurity Implications: The findings suggest improved malware detection techniques, new mitigation strategies for backdoor attacks, and the need for collaborative data-sharing initiatives.
- Future Directions: Future work includes integrating PBP into real-time systems, developing adaptive defenses, exploring synthetic data generation, and establishing benchmarks for effectiveness evaluation.
Advancements in Cybersecurity: Post-Training Defense Mechanisms Against Backdoor Attacks
In a significant development for cybersecurity, recent research spearheaded by Dung Thuy Nguyen and colleagues has introduced innovative post-training defenses against backdoor attacks in malware classifiers. This study unveils the Post-Backdoor Purification (PBP) method, which promises to bolster the security and reliability of machine learning models, particularly those used in malware detection. These advancements could potentially reshape cybersecurity practices, providing more robust tools to counteract malicious interventions in machine learning systems.
Key Objectives and Methodology
The research is anchored on several pivotal objectives aimed at fortifying machine learning models:
-
Neuron Mask Generation: This process involves identifying and isolating neurons that contribute to compromised model behavior, thereby mitigating their influence.
-
Activation-Shift Fine-Tuning: The focus here is on adjusting the model to minimize the impact of backdoor neurons while maintaining effectiveness on legitimate data.
-
Statistical Alignment of Batch Normalization Layers: Ensuring consistency and reliability in model performance across diverse data inputs is a critical goal.
The methodology employed includes:
-
Layer-wise Activation Alignment: This strategy aligns neuron activations across layers, enhancing model robustness against backdoor attacks.
-
Backdoor Model Purification: A crucial process that evaluates and mitigates the impact of backdoor attacks on model performance.
-
Hyperparameter Tuning: Future work will explore automated methods for optimizing model parameters to improve usability and effectiveness across various applications.
Key Findings
The study’s findings highlight the efficacy of the PBP method:
-
Enhanced Defense Mechanisms: PBP establishes a reliable post-training defense against backdoor attacks, significantly boosting the resilience of malware classifiers.
-
Insights into Model Behavior: The research offers valuable insights into neuron activity and model behavior, deepening understanding of how backdoor attacks exploit vulnerabilities.
-
Broader Applicability: The methodologies developed extend beyond malware detection, with potential applications across various domains.
Implications for Cybersecurity
The implications of this research are far-reaching for cybersecurity:
-
Improved Malware Detection Techniques: The advancement of PBP could lead to more effective malware detection systems that are less prone to manipulation.
-
Backdoor Attack Mitigation Strategies: New strategies derived from this research can enhance existing defenses against sophisticated cyber threats.
-
Collaboration and Data Sharing Initiatives: Collaborative frameworks for sharing data and insights will be crucial to strengthening defenses against evolving threats.
-
Cross-Domain Applications: The methodologies developed can be adapted for use in various cybersecurity domains, including Internet of Things (IoT) security and autonomous systems.
Strengths and Limitations
While the research showcases significant strengths, such as the innovative PBP methodology and its practical implications, it also identifies limitations. There is a need for further investigation into hyperparameter optimization and the practicality of data collection for real-world applications.
Future Directions
The research outlines several future directions to bolster cybersecurity efforts:
-
Integration into Real-Time Malware Detection Systems: Implementing PBP in real-time systems can enhance their responsiveness to threats.
-
Development of Adaptive Defense Mechanisms: Creating systems that can dynamically adapt to new threats will improve overall security.
-
Exploration of Synthetic Data Generation Techniques: This could facilitate the construction of comprehensive fine-tuning datasets necessary for training robust models.
-
Benchmarking and Standardization: Establishing benchmarks for evaluating the effectiveness of PBP will be crucial as the field evolves.
As cybersecurity continues to face complex challenges, these advancements represent a critical step forward in enhancing the resilience of machine learning models against backdoor attacks.