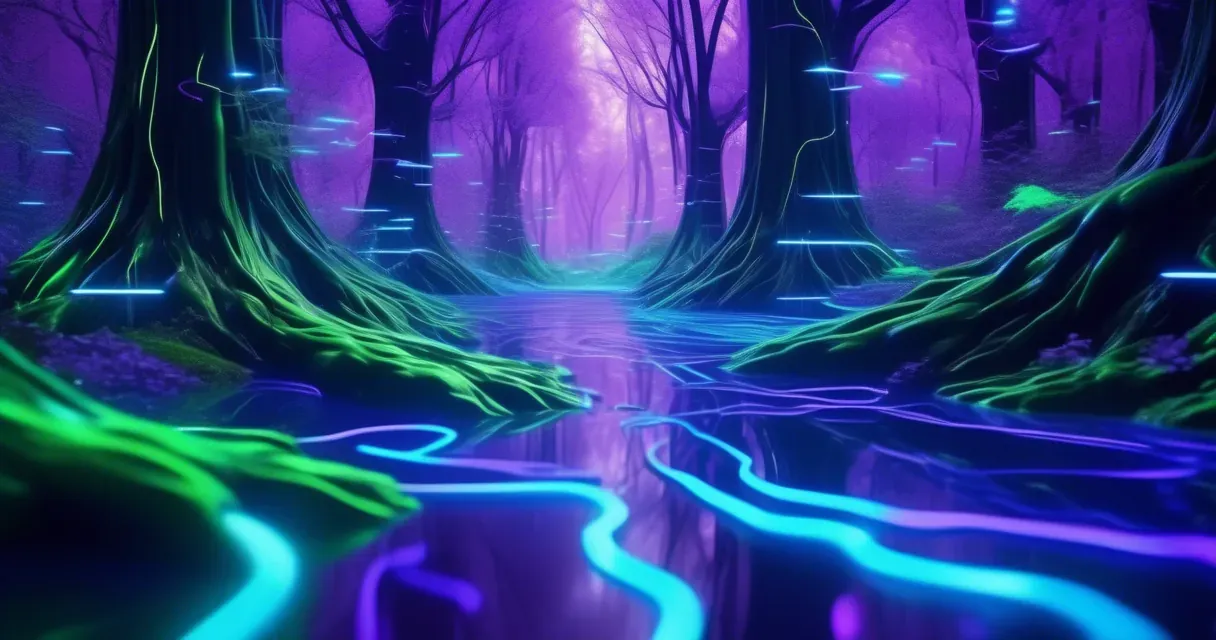
AWS Audit Manager Framework for Generative AI Adoption
/ 4 min read
Quick take - Organizations are increasingly adopting generative AI technologies, necessitating a structured approach to risk management and implementation, with the AWS Audit Manager framework providing essential strategies for effective integration and mitigation of associated risks.
Fast Facts
-
Structured Risk Management: Organizations must adopt a structured approach to risk management when integrating generative AI, focusing on data quality, model explainability, and data privacy to mitigate potential risks.
-
AWS Audit Manager Framework: The AWS Audit Manager provides a four-pillar framework (Data Governance, Model Development, Model Deployment, Monitoring & Oversight) to guide responsible adoption and implementation of generative AI technologies.
-
Integration with Amazon Services: Organizations are encouraged to combine the AWS framework with tools like Amazon Bedrock or Amazon SageMaker to customize controls that meet their specific operational needs.
-
Data Privacy and Security: Ensuring that training data is free from confidential or sensitive information is crucial for protecting data privacy and security during the implementation of generative AI.
-
Importance of Planning: Successful generative AI adoption requires meticulous planning and execution, with a focus on governance, risk management, and continuous monitoring to avoid common pitfalls and vulnerabilities.
Navigating the Integration of Generative AI in Organizations
In the rapidly evolving landscape of technology, generative AI stands out as a transformative force for businesses. However, its integration into organizational frameworks is not without challenges. As companies increasingly consider adopting these advanced systems, a structured approach to risk management and implementation becomes crucial.
The Dual Nature of Generative AI
Generative AI offers significant opportunities for innovation and efficiency but also presents potential risks that must be carefully managed. Organizations are urged to align their risk management strategies with robust mitigation measures to navigate these challenges effectively. A key resource in this endeavor is the AWS Audit Manager generative AI best practices framework, which provides a comprehensive tool for evaluating and adopting these technologies responsibly.
Essential Risk Assessment
A thorough risk assessment is foundational to the successful integration of generative AI. Key areas of concern include data quality, model explainability, and data privacy. Ensuring high data quality is critical to avoid biased outputs, which can lead to severe financial and regulatory repercussions. Organizations must be vigilant in maintaining the integrity and accuracy of their data inputs.
The AWS Audit Manager Framework
The AWS Audit Manager framework serves as a structured guide for organizations aiming to adopt generative AI responsibly. It comprises four essential pillars:
-
Data Governance: This pillar emphasizes sourcing, maintaining, and ensuring data quality while addressing privacy and bias concerns. Effective data governance is crucial for building trust in AI outputs.
-
Model Development: Organizations are guided in selecting responsible model architectures and conducting rigorous evaluations during development. This ensures that models are both effective and ethical.
-
Model Deployment: The framework outlines strategies for deploying models and infrastructure considerations necessary for effective implementation in production environments.
-
Monitoring & Oversight: Continuous monitoring is vital to identify and address potential risks and performance issues over time, ensuring long-term reliability and safety.
Integrating with Amazon Services
To tailor controls that cater to unique operational needs, organizations are encouraged to integrate the AWS framework with Amazon Bedrock or Amazon SageMaker. This integration ensures that generative AI systems remain both effective and secure, aligning with specific business objectives while safeguarding against potential threats.
Safeguarding Data Privacy and Security
Protecting sensitive information is paramount when dealing with generative AI. Organizations must ensure that training data does not inadvertently include confidential or sensitive information, thereby safeguarding data privacy and security. This proactive approach helps prevent unauthorized access or misuse of critical data assets.
Implications for Successful Adoption
The path to successful generative AI adoption hinges on meticulous planning and execution. Common pitfalls such as neglecting data quality, inadequate risk management alignment, and insufficient access controls can expose organizations to significant vulnerabilities. By leveraging the AWS Audit Manager framework, companies can establish a comprehensive strategy encompassing effective governance, responsible development, and rigorous monitoring.
As the landscape of generative AI continues to evolve, organizations prioritizing these best practices will not only mitigate risks but also unlock the full potential of generative AI technologies. This strategic alignment is essential for enhancing operations and driving innovation in an increasingly competitive market.