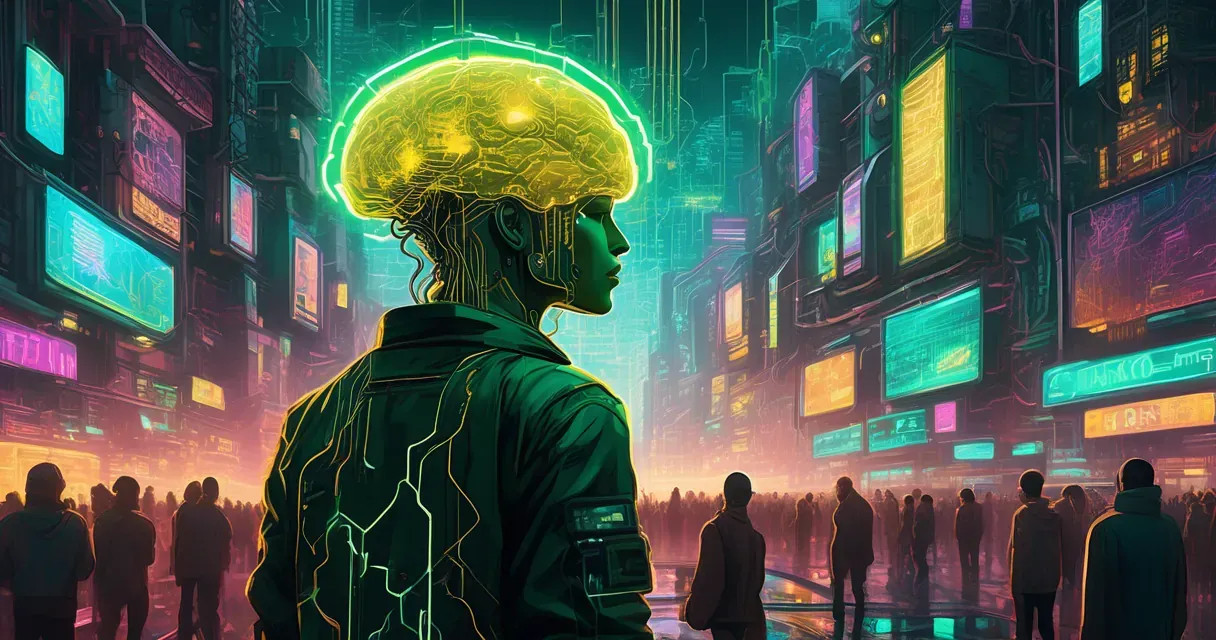
Advancements in Scam Detection Using Large Language Models
/ 3 min read
Quick take - Recent research by Tianhui Huang and Jessica Staddon highlights the potential of large language models in enhancing scam detection while addressing critical issues related to bias, reliability, and ethical considerations in consumer protection.
Fast Facts
- Recent research by Tianhui Huang and Jessica Staddon highlights the use of large language models (LLMs) for improving scam detection and consumer protection.
- The study developed a robust dataset through data collection and manual labeling, leading to enhanced detection mechanisms and user education strategies.
- Key findings emphasize the need for ethical considerations, addressing bias, reliability, and data privacy in LLM applications.
- Proposed tools include user education resources, collaboration with financial institutions for real-time alerts, and a centralized scam reporting dashboard.
- The research underscores the importance of continuous evaluation and ethical guidelines to ensure effective and responsible use of LLMs in combating scams.
Advancements in Scam Detection Through Large Language Models
In a significant stride towards enhancing consumer protection, recent research by Tianhui Huang and Jessica Staddon has unveiled promising advancements in scam detection using large language models (LLMs). This study not only highlights the potential of LLMs in identifying scams but also underscores critical areas requiring further refinement to ensure robust consumer safety.
Key Findings and Methodology
The research embarked on a comprehensive journey, beginning with meticulous data collection and manual labeling to create a robust dataset for training LLMs. This foundational step was crucial for developing sophisticated mechanisms capable of detecting scams with greater accuracy. The iterative development of prompts aimed at refining model outputs played a pivotal role in this process.
An ensemble model was subsequently crafted to enhance detection accuracy, followed by rigorous error analysis and performance evaluation. These steps were essential in assessing the effectiveness of the model, revealing significant advancements in leveraging LLM capabilities for scam detection.
Enhancing User Education and Interaction Design
A notable aspect of the study is its emphasis on user education and interaction design. By equipping consumers with the knowledge to recognize and respond to potential scams, the research advocates for a proactive approach to consumer protection. This focus on education is vital as it empowers users to be vigilant against evolving digital threats.
Addressing Bias and Ethical Considerations
Despite these advancements, the study acknowledges concerns regarding bias and reliability within LLMs. The potential for bias poses a challenge that must be addressed to build trust among users. Additionally, data privacy and ethical considerations are paramount, necessitating careful attention to ensure that these technologies are used responsibly.
Proposed Tools and Frameworks
To facilitate the practical application of their findings, Huang and Staddon propose several tools and frameworks:
-
Enhanced User Education Tools: These aim to empower consumers with insights into scam tactics and prevention strategies.
-
Integration with Financial Institutions: Collaborating with banks could enable real-time scam detection and alerts, bolstering consumer safety.
-
Scam Reporting Dashboard: A centralized platform for reporting scams could aid in analyzing patterns and developing more effective detection strategies.
-
Personalized Scam Risk Assessment: Utilizing LLMs to assess individual risk profiles can lead to tailored recommendations.
-
Ethical Guidelines Development: Establishing guidelines is crucial for ensuring privacy and fairness in LLM applications.
Implications for Consumer Protection
The implications of this research are profound, highlighting the potential of LLMs in safeguarding consumers from scams while emphasizing the importance of ethical considerations. As digital threats continue to evolve, integrating advanced models into consumer protection strategies could play a pivotal role in defending individuals against financial fraud and deception.
This research sets the stage for future developments in scam detection technology, urging continuous improvement and ethical vigilance as key components of effective consumer protection frameworks.