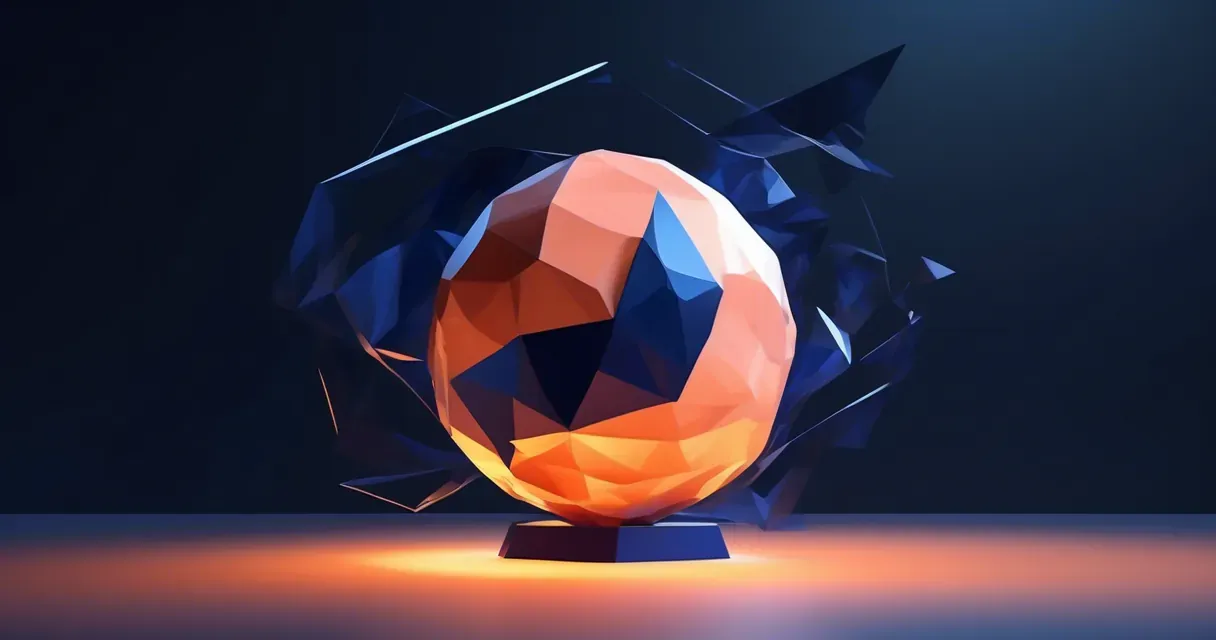
New SPIDEr Framework Aims to Enhance Data Privacy
/ 4 min read
Quick take - Recent research has introduced the Secure Pipeline for Information De-Identification with End-to-End Encryption (SPIDEr), a framework designed to enhance data privacy through the use of Trusted Execution Environments, formal privacy guarantees, and advanced processing techniques, with implications for sectors such as healthcare and IoT.
Fast Facts
-
Introduction of SPIDEr Framework: The Secure Pipeline for Information De-Identification with End-to-End Encryption (SPIDEr) enhances data privacy through Trusted Execution Environments (TEEs) and advanced processing techniques.
-
Key Findings: SPIDEr ensures data confidentiality, formalizes privacy guarantees for regulatory compliance, and optimizes performance for large-scale data handling.
-
Robust Attestation Mechanism: The framework emphasizes the importance of verifying cloud service integrity to maintain secure data processing environments.
-
Applications in Critical Sectors: SPIDEr is particularly beneficial for healthcare and IoT, enabling real-time analytics while safeguarding sensitive information.
-
Future Research Directions: Further investigation is needed for real-time analytics capabilities and compliance with international data protection regulations.
Advancements in Data Privacy: Unveiling the SPIDEr Framework
In a world where data breaches and privacy concerns are increasingly prevalent, recent research has introduced a promising advancement in data protection methodologies. The Secure Pipeline for Information De-Identification with End-to-End Encryption (SPIDEr) framework emerges as a significant development aimed at bolstering data privacy through cutting-edge techniques. This innovative approach leverages Trusted Execution Environments (TEEs), formalizes privacy guarantees, and implements advanced data processing methods to address the growing need for robust security measures.
Key Findings and Objectives
The SPIDEr research focused on several critical objectives to enhance data privacy. At the forefront is the implementation of TEEs, which provide a secure environment for processing sensitive information. These environments ensure that data remains confidential and tamper-proof, a crucial aspect in sectors like healthcare and IoT where data sensitivity is paramount.
Another cornerstone of the SPIDEr framework is its formal privacy guarantees. By aligning with regulatory standards, the framework enhances trust among users and organizations, offering assurances that their data is handled with utmost care. Additionally, SPIDEr is designed for scalability and performance optimization, making it adept at handling large volumes of data efficiently. This capability is particularly beneficial for real-time analytics and operational scalability.
A notable aspect of the research is the emphasis on attestation and trust in cloud environments. The study underscores the necessity of a robust attestation mechanism to verify the integrity of cloud services, ensuring that data processing occurs within secure parameters.
Strengths of the SPIDEr Framework
The SPIDEr framework’s comprehensive approach to privacy sets it apart from existing methodologies. It integrates state-of-the-art encryption techniques and demonstrates adaptability across various applications, including IoT and healthcare. A key strength lies in its ability to combine different privacy methodologies such as K-Anonymisation and Differential Privacy, enhancing its effectiveness in protecting individual identities within datasets.
Limitations and Areas for Further Exploration
Despite its strengths, the research acknowledges certain limitations that require further investigation. One area identified is the need for more robust real-time data analytics capabilities. Additionally, cross-border data sharing compliance poses challenges due to varying international data protection regulations. Addressing these limitations will be crucial for maximizing the framework’s potential.
Recommended Tools and Techniques
To improve data privacy, the research advocates for adopting several tools and frameworks:
- SPIDEr: The core framework integrating various privacy methodologies and encryption techniques.
- Trusted Execution Environments (TEEs): Essential for securing data during processing.
- K-Anonymisation: A technique to protect individual identities within datasets.
- Differential Privacy: Ensures aggregate data analysis without compromising individual privacy.
Applications and Implications
The implications of this research are profound, particularly in sectors where data breaches can have severe consequences. By integrating SPIDEr with IoT devices, organizations can significantly enhance the protection of sensitive information. In healthcare, this means safeguarding patient data while supporting real-time analytics for timely insights.
Moreover, by addressing cross-border data sharing compliance, SPIDEr facilitates international collaborations without compromising security. This capability is vital as organizations increasingly operate on a global scale.
As digital landscapes continue to evolve, frameworks like SPIDEr represent a significant step forward in enhancing data privacy and security. The ongoing development and implementation of such methodologies underscore the critical need for innovative solutions to safeguard sensitive information in an interconnected world.
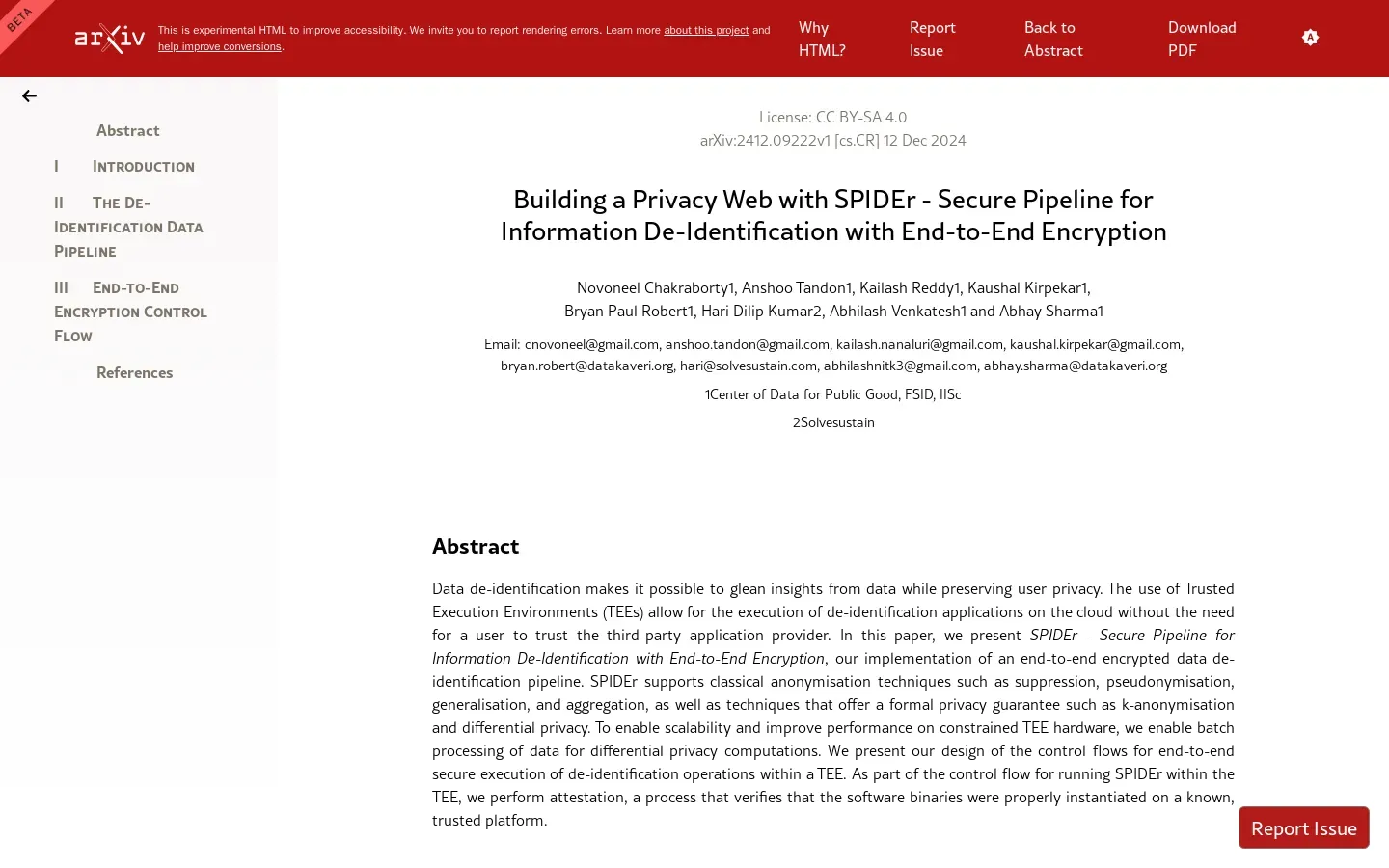