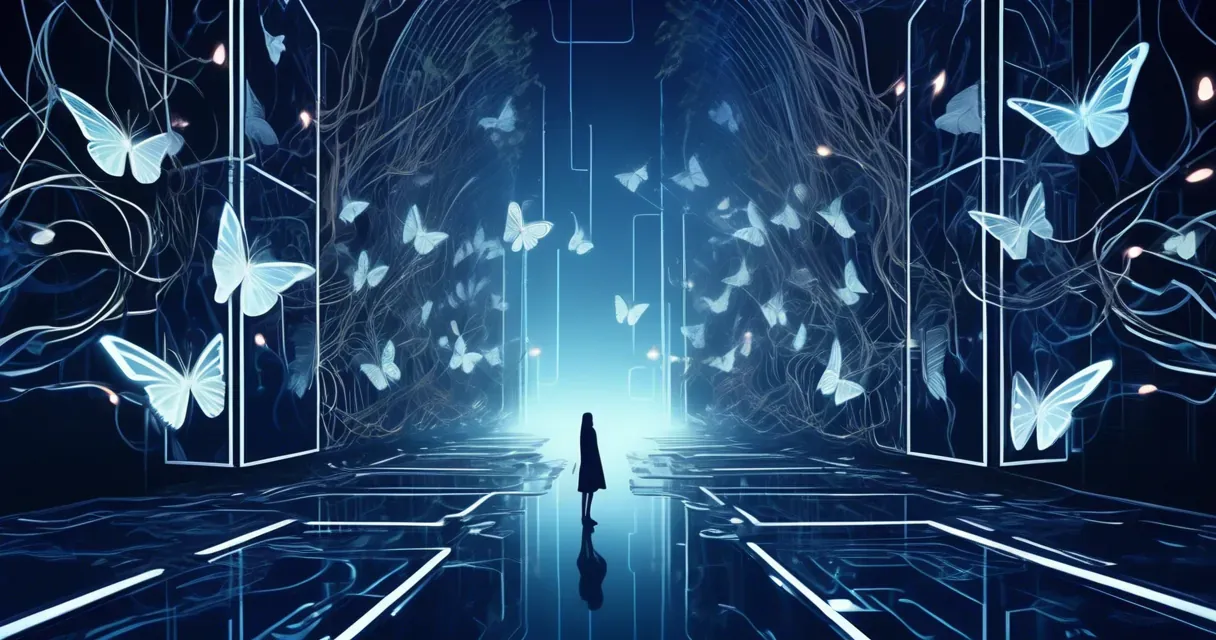
Advancements in Adversarial Attacks on Malware Detection
/ 3 min read
Quick take - Recent research has advanced adversarial attack strategies for malware detection, focusing on the secml-malware library to enhance cybersecurity measures through improved integration and evaluation of detection models in real-time environments.
Fast Facts
- Recent research has advanced adversarial attack strategies for Windows malware detection, focusing on the secml-malware library’s modular architecture.
- The study emphasizes integration with real-time threat detection systems, enhancing the practical application of developed tools in live environments.
- Automated penetration testing frameworks are highlighted as essential for simulating attacks and evaluating the resilience of malware detectors.
- The research calls for robust, cross-platform malware detection capabilities to address the diverse environments where malware can spread.
- Continuous adaptation and innovation are necessary to keep pace with evolving malware threats, underscoring the importance of ongoing research in cybersecurity.
Advancements in Adversarial Attack Strategies for Malware Detection
Recent research into adversarial attacks on Windows malware detectors has unveiled significant developments aimed at bolstering cybersecurity measures against increasingly sophisticated threats. This study, centered around the secml-malware library, employs a modular library architecture to enhance the understanding of adversarial attacks and improve defenses against them.
Overview of Findings
At the heart of this research is the systematic development of adversarial attack strategies that can be integrated with existing malware detection tools. By leveraging a modular architecture, the secml-malware library allows for flexibility and scalability in evaluating the robustness of various malware detection models. This approach not only enhances the understanding of how adversarial attacks operate but also provides a comprehensive framework for assessing the efficacy of defenses in real-world scenarios.
Supporting Details
A key strength of the research is its focus on integration with real-time threat detection systems. This capability ensures that the developed tools are not merely theoretical but also applicable in live environments where timely responses to threats are critical. The study underscores the necessity for robust malware detection models, which are essential for maintaining the integrity of cybersecurity ecosystems.
Additionally, the research highlights the importance of automated penetration testing frameworks. These frameworks can simulate adversarial attacks on malware detectors to evaluate their resilience. The study also advocates for cross-platform malware detection capabilities, recognizing the diverse environments in which malware can propagate and the need for adaptable detection mechanisms.
Limitations and Areas for Further Investigation
Despite its promising findings, the research acknowledges certain limitations and areas requiring further investigation. The evolving nature of malware and adversarial techniques necessitates continuous adaptation and innovation to keep pace with new threats. Ongoing research into advanced methodologies and tools is crucial for enhancing the effectiveness of malware detection systems.
Implications for Cybersecurity
The implications of this research are significant for cybersecurity. By pursuing recommended directions such as integrating advanced detection systems and developing automated frameworks, this research can substantially enhance the resilience of malware detection tools. These advancements empower cybersecurity professionals to better anticipate and mitigate risks posed by adversarial attacks, ultimately contributing to a safer digital environment.
In light of these developments, organizations should consider investing in adaptive security measures that incorporate findings from this research. Emphasizing cross-platform capabilities and real-time threat response will be crucial steps in fortifying defenses against future adversarial threats.
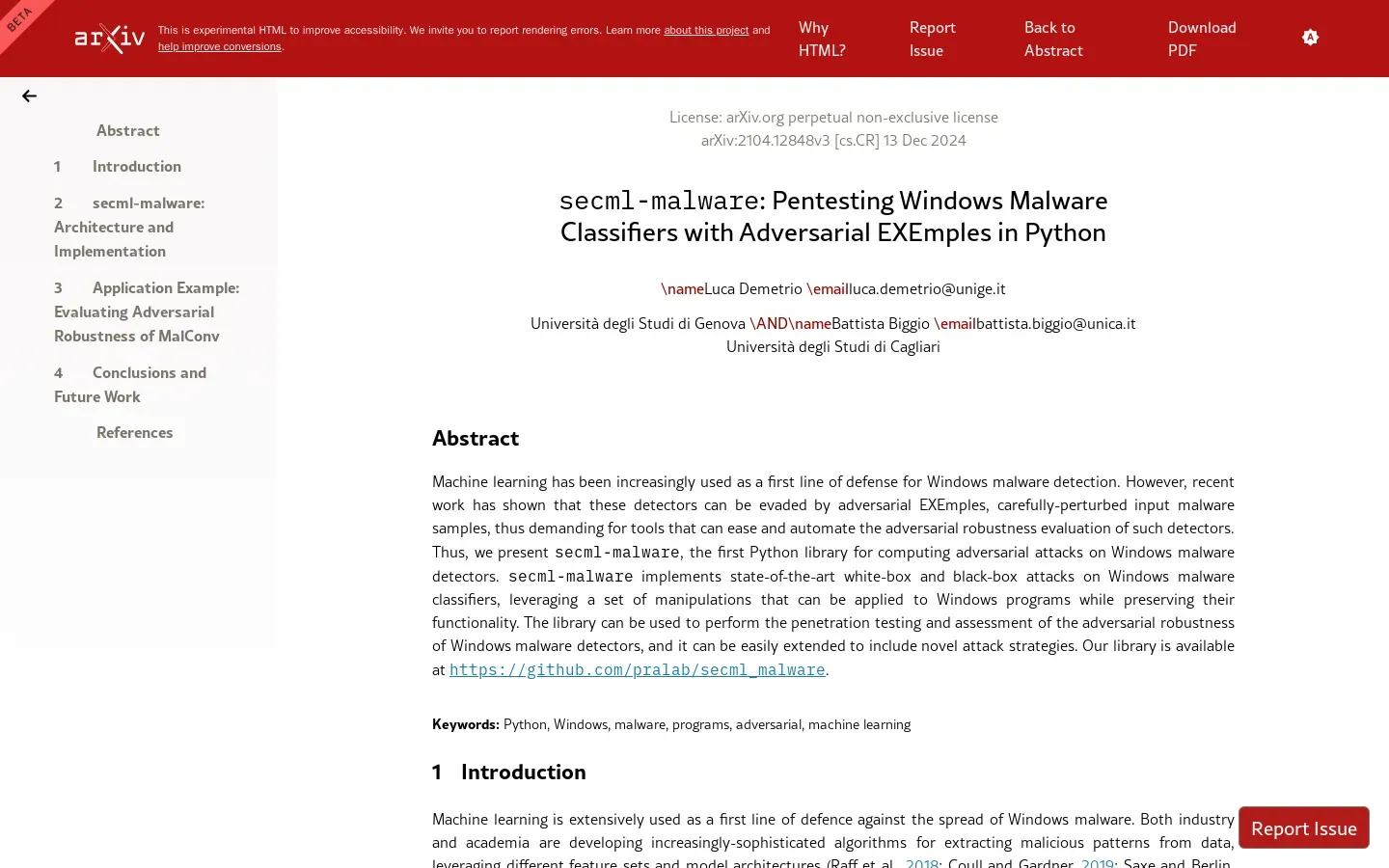