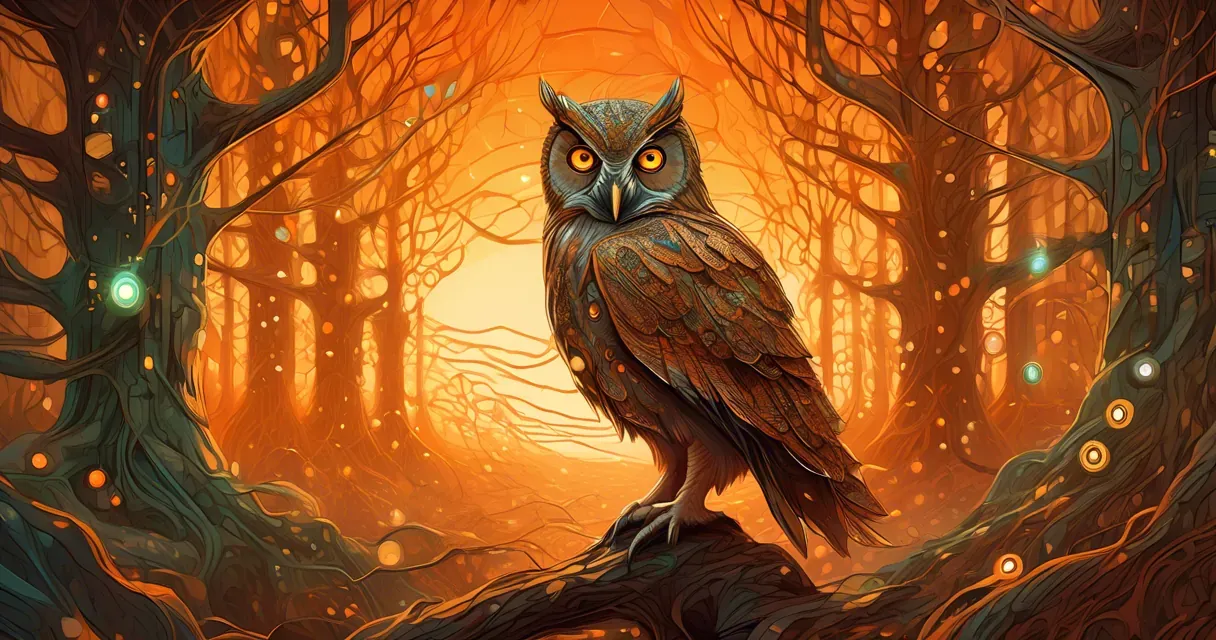
Machine Learning Framework Developed for Smishing Detection
/ 4 min read
Quick take - Recent research has advanced the detection of smishing and SMS spam through innovative filtering methodologies and machine learning techniques, highlighting the importance of real-time detection systems and user awareness in enhancing mobile communication security.
Fast Facts
- Recent research has advanced smishing detection and SMS spam filtering, focusing on innovative methodologies to combat SMS phishing attacks.
- Key objectives include developing effective filtering systems like SMSassassin and real-time detection mechanisms within cloud environments.
- The study employs machine learning algorithms and comprehensive text preprocessing to enhance classification accuracy for smishing messages.
- Findings indicate that integrating content analysis and crowdsourced data significantly improves detection rates, with effective algorithms identified for threat identification.
- Future directions emphasize expanding detection to multi-modal platforms, real-time threat intelligence sharing, and user education on smishing risks.
Advancements in Smishing Detection and SMS Spam Filtering: A New Frontier in Cybersecurity
In a significant leap forward for cybersecurity, recent research has unveiled innovative methodologies aimed at detecting smishing—SMS phishing attacks that trick users into revealing personal information. As these threats become increasingly prevalent, the study highlights the urgent need for effective detection systems to protect mobile communications.
Objectives and Research Focus
The research is driven by several key objectives. It aims to develop robust SMS spam filtering systems like SMSassassin, which leverage crowdsourcing to effectively filter out spam and smishing messages. Another critical focus is the investigation of real-time detection mechanisms within cloud computing environments, designed to enhance both response times and accuracy. Additionally, the study seeks to improve detection models by incorporating new content-based features and text normalization techniques.
Methodology and Approach
The study employs a comprehensive methodology that includes several pivotal steps:
-
Text Preprocessing and Normalization: This foundational step involves tokenization, lowercasing, stop word removal, and stemming to prepare data for analysis.
-
Machine Learning Classification: Various machine learning algorithms, such as the Naïve Bayesian Classifier, are applied to classify smishing and spam messages effectively.
-
Experimental Validation: The proposed models undergo rigorous experimental validation to assess their performance in real-world scenarios.
-
Comparative Analysis: A thorough comparison of different techniques is conducted to identify the most effective methods for smishing detection.
Key Findings
The research presents several noteworthy findings. The implementation of SMS spam filtering frameworks significantly boosts the accuracy of smishing message detection. Integrating content and URL behavior analysis with crowdsourced data further enhances detection rates. Notably, the Dendritic Cell Algorithm and rule-based frameworks prove effective in identifying smishing threats.
Implications for Cybersecurity
These findings carry profound implications for the cybersecurity landscape. They underscore the necessity for continuous innovation to counteract the evolving tactics of cybercriminals. Real-time detection mechanisms are crucial for swiftly responding to emerging threats. Moreover, user-centric awareness programs are vital in educating individuals about the dangers of smishing.
Strengths and Limitations
The research stands out for its robust approach, combining machine learning techniques with community engagement. It offers a comprehensive framework adaptable across various mobile communication platforms. However, it also identifies limitations, such as the need for broader datasets to enhance model training and validation. Further exploration of additional machine learning algorithms and hybrid approaches could improve accuracy.
Future Directions
Looking ahead, several potential future directions emerge:
-
Integration with Multi-Modal Communication Platforms: Expanding detection capabilities beyond SMS to include other forms of communication.
-
Real-Time Threat Intelligence Sharing: Enhancing information sharing among users and organizations for better threat response.
-
User-Centric Awareness Programs: Developing educational initiatives to help users recognize and respond to smishing attempts.
-
Adaptive Machine Learning Models: Creating personalized security measures that adapt to user behavior and evolving threat landscapes.
As advancements in smishing detection and SMS spam filtering continue to unfold, they play a crucial role in bolstering cybersecurity measures. The proposed frameworks and methodologies offer a promising path towards a more secure mobile communication environment, emphasizing the need for ongoing evolution to stay ahead of cyber threats.