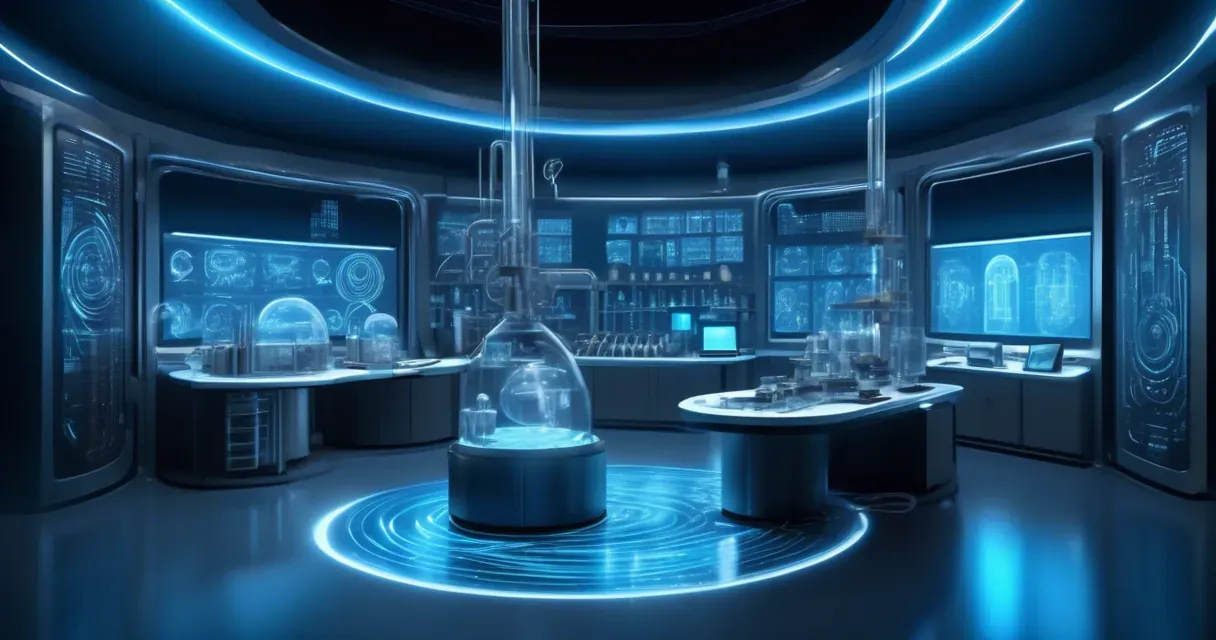
Advancements in Controllable Website Fingerprint Defense Research
/ 4 min read
Quick take - Recent research on Controllable Website Fingerprint Defense (CWFD) has provided insights into improving defenses against website fingerprinting attacks by optimizing trigger patterns, implementing server-side injections, and manipulating attackers’ models, while also highlighting the need for further exploration of data overhead and real-world application effectiveness.
Fast Facts
- Research Focus: The study on Controllable Website Fingerprint Defense (CWFD) aims to mitigate website fingerprinting attacks through trigger pattern optimization, server-side injections, and model poisoning.
- Key Objectives: It emphasizes developing undetectable static and dynamic triggers, integrating them into network traffic with minimal overhead, and manipulating attackers’ training data.
- Findings: CWFD shows adaptability against various attack strategies but faces challenges in optimizing data overhead while enhancing defense effectiveness.
- Methodology: The research utilized LSTM-based models for dynamic trigger prediction, alongside greedy optimization and performance evaluation against different attack models.
- Future Directions: Further investigation is needed to optimize overhead and evaluate CWFD in real-world scenarios, with implications for adaptive defenses and privacy-preserving protocols across various domains.
Advancements in Controllable Website Fingerprint Defense: A New Frontier in Cybersecurity
Recent advancements in Controllable Website Fingerprint Defense (CWFD) research have unveiled promising strategies to counteract website fingerprinting attacks, a growing threat to online privacy. This study sheds light on innovative methods to enhance cybersecurity defenses while maintaining minimal system overhead.
Key Objectives and Research Questions
The research is driven by three primary objectives:
-
Trigger Pattern Optimization: The development of both static and dynamic triggers aims to mislead fingerprinting systems effectively. These triggers are designed to remain undetectable, ensuring they do not compromise the integrity of network traffic.
-
Server-Side Injection of Triggers: By integrating optimized trigger patterns directly into network traffic, researchers aim to maintain the natural characteristics of the data flow. This approach seeks to ensure that the defense mechanisms impose minimal additional load on the system.
-
Poisoning the Attacker’s Model: This involves manipulating the training data used by attackers, thereby degrading their model’s performance during training phases. Such a strategy could significantly impair an attacker’s ability to accurately fingerprint websites.
Research Findings
The CWFD approach demonstrates notable strengths, particularly its adaptability to various data access conditions and attack strategies. This adaptability is crucial for efficiently mitigating fingerprinting attacks. However, challenges persist in balancing data overhead with defense effectiveness, highlighting areas for further exploration.
Methodology
The research employed a structured methodology:
-
Scenario Definition and Data Access Conditions: Various scenarios were established to test the CWFD’s effectiveness under different conditions.
-
Optimization Strategy Selection: Strategies were chosen to optimize trigger patterns, ensuring they are both effective and efficient.
-
Dynamic Trigger Prediction Model: An LSTM-based model was utilized for predicting adaptive trigger patterns, enhancing the system’s responsiveness.
-
Evaluation and Performance Metrics: The developed strategies were rigorously assessed against diverse attack models to gauge their efficacy.
Tools and Techniques
Several tools and frameworks were pivotal in this research:
-
Dynamic Trigger Prediction Model (LSTM-based): This model generates adaptive trigger patterns that can evolve with changing attack landscapes.
-
Greedy Optimization Approach: Used to refine trigger patterns for maximum effectiveness.
-
Sequence Difference Loss and Constraint Loss: These techniques help fine-tune the model’s predictions, ensuring accuracy.
-
t-SNE Visualization: Employed for visual analysis of defense mechanism effectiveness, providing insights into how well the CWFD can obscure website fingerprints.
Implications for Cybersecurity
The implications of these findings are far-reaching:
-
Adaptive Defense Mechanisms: The research advocates for defenses that can dynamically adapt to new fingerprinting techniques, enhancing resilience against evolving threats.
-
Cross-Domain Applications: Potential applications extend beyond traditional web environments, including IoT devices and privacy-focused browsers.
-
Collaboration with Machine Learning: Leveraging machine learning can bolster threat intelligence capabilities, making adaptive defenses more robust.
-
Privacy-Preserving Protocols: There is a pressing need for privacy-centric tools and collaboration with industry stakeholders to standardize practices across sectors.
Areas for Further Investigation
The study identifies several avenues for future research:
-
Optimization of Overhead: Further investigation is needed to minimize data overhead without compromising defense efficacy.
-
Real-World Deployment and Evaluation: Testing CWFD in practical settings will be crucial for assessing its real-world effectiveness against contemporary attacks.
These advancements in CWFD research mark a significant step forward in protecting online privacy. By developing new strategies and tools, this research contributes to enhancing security measures in our increasingly digital world.