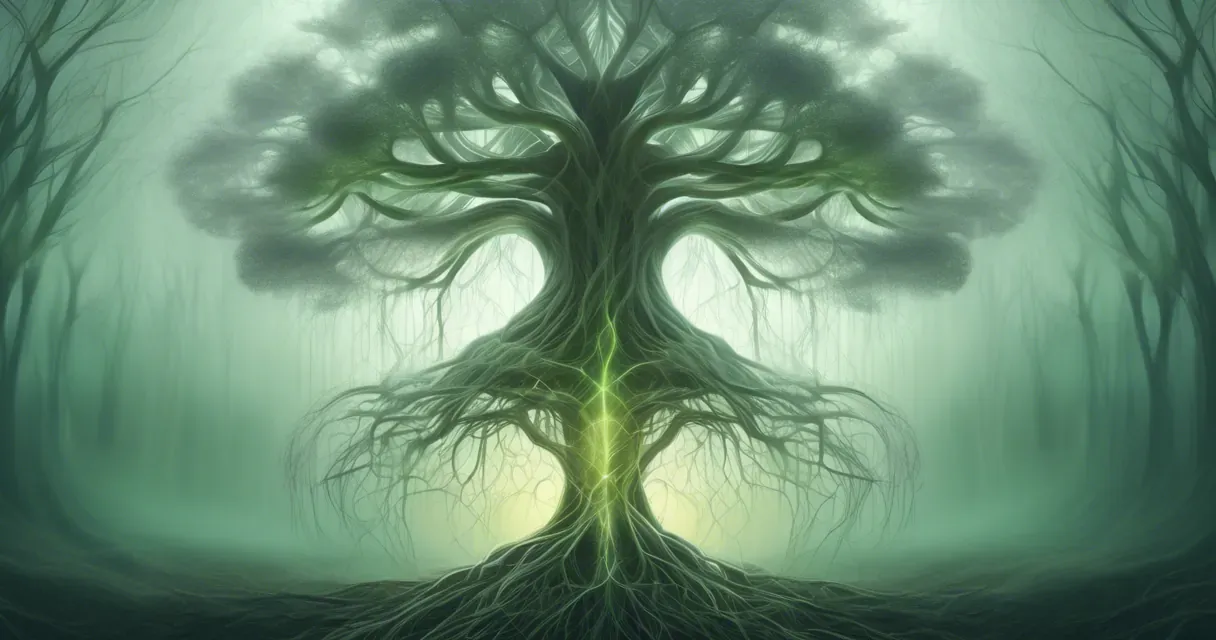
AI Integration in Cybersecurity: NIDS Rules Labeling Study
/ 3 min read
Quick take - Recent research has explored the integration of advanced artificial intelligence techniques, particularly Large Language Models and machine learning, to automate the labeling of Network Intrusion Detection System rules, aiming to enhance threat detection and response capabilities in cybersecurity.
Fast Facts
- Recent research integrates AI, specifically Large Language Models (LLMs) and machine learning (ML), to automate the labeling of Network Intrusion Detection System (NIDS) rules using MITRE ATT&CK techniques, enhancing threat detection and response.
- The methodology includes dataset preparation, prompt engineering for LLMs, ML model development, and performance evaluation against traditional models.
- Key findings indicate improved automation in threat detection, hybrid approaches for better accuracy, and the importance of high-quality training data.
- The study highlights the potential for explainable AI in cybersecurity, which could enhance understanding of model decision-making processes.
- Future directions include developing real-time threat detection systems, automating threat intelligence generation, and customizing models for specific environments.
Advancements in Cybersecurity Through AI Integration
In a notable development for cybersecurity, recent research has explored the integration of advanced artificial intelligence techniques to enhance threat detection and response mechanisms. By utilizing Large Language Models (LLMs) and machine learning (ML) models, the study aims to automate the labeling of Network Intrusion Detection System (NIDS) rules with MITRE ATT&CK techniques and tactics, offering a promising avenue for improving cybersecurity measures.
Methodology Overview
The research employed a comprehensive methodology, beginning with the collection and preparation of extensive datasets. This foundational step was crucial for training and evaluating the models effectively. Following this, prompt engineering was applied to LLMs, optimizing their performance within the cybersecurity context. The development of robust ML models tailored for threat detection applications was another key component. Finally, the study systematically evaluated and compared the performance of LLMs against traditional ML models to identify their respective strengths and weaknesses.
Key Findings and Implications
The integration of LLMs and ML models in cybersecurity has led to several significant findings:
- Enhanced Automation: Automating the labeling of NIDS rules with MITRE ATT&CK techniques can significantly accelerate threat detection processes.
- Hybrid Approaches: The complementary use of LLMs and ML models shows promise in refining threat detection accuracy.
- Training Data Quality: The success of these models is heavily reliant on the quality and relevance of training data.
- Explainable AI: There is potential for developing explainable AI systems within cybersecurity, which could improve understanding of model-derived conclusions.
These insights suggest transformative potential in cybersecurity practices, paving the way for more efficient threat detection and response mechanisms.
Strengths and Limitations
The research highlights several strengths, such as the innovative application of AI technologies and potential enhancements in cybersecurity operations. However, it also points out limitations, particularly regarding the need for higher quality training data and refinement in automated labeling systems. Addressing these areas will be crucial for future research and practical applications.
Recommended Tools and Techniques
The study identifies several tools and techniques that could advance cybersecurity efforts:
- Large Language Models (LLMs): These are valuable for processing vast amounts of textual data relevant to cybersecurity.
- Prompt Engineering: Essential for optimizing interactions between LLMs and specific cybersecurity tasks.
- Machine Learning Models: When integrated with LLMs, they can enhance overall accuracy.
- MITRE ATT&CK Framework: A vital reference for categorizing various attack techniques.
Future Directions
Looking forward, the research outlines promising directions for applying these findings in real-world scenarios:
- Real-Time Threat Detection Systems: Developing systems capable of providing immediate alerts and responses to detected threats.
- Automated Threat Intelligence Generation: Streamlining the creation of actionable threat intelligence.
- Domain-Specific Adaptation: Customizing models for specific contexts like industrial control systems or cloud environments to boost performance.
These advancements represent a significant leap towards scalable cyber threat intelligence solutions, ultimately enhancing organizational cybersecurity worldwide. As the field evolves, ongoing research will be essential to fully harness AI’s potential in cybersecurity.
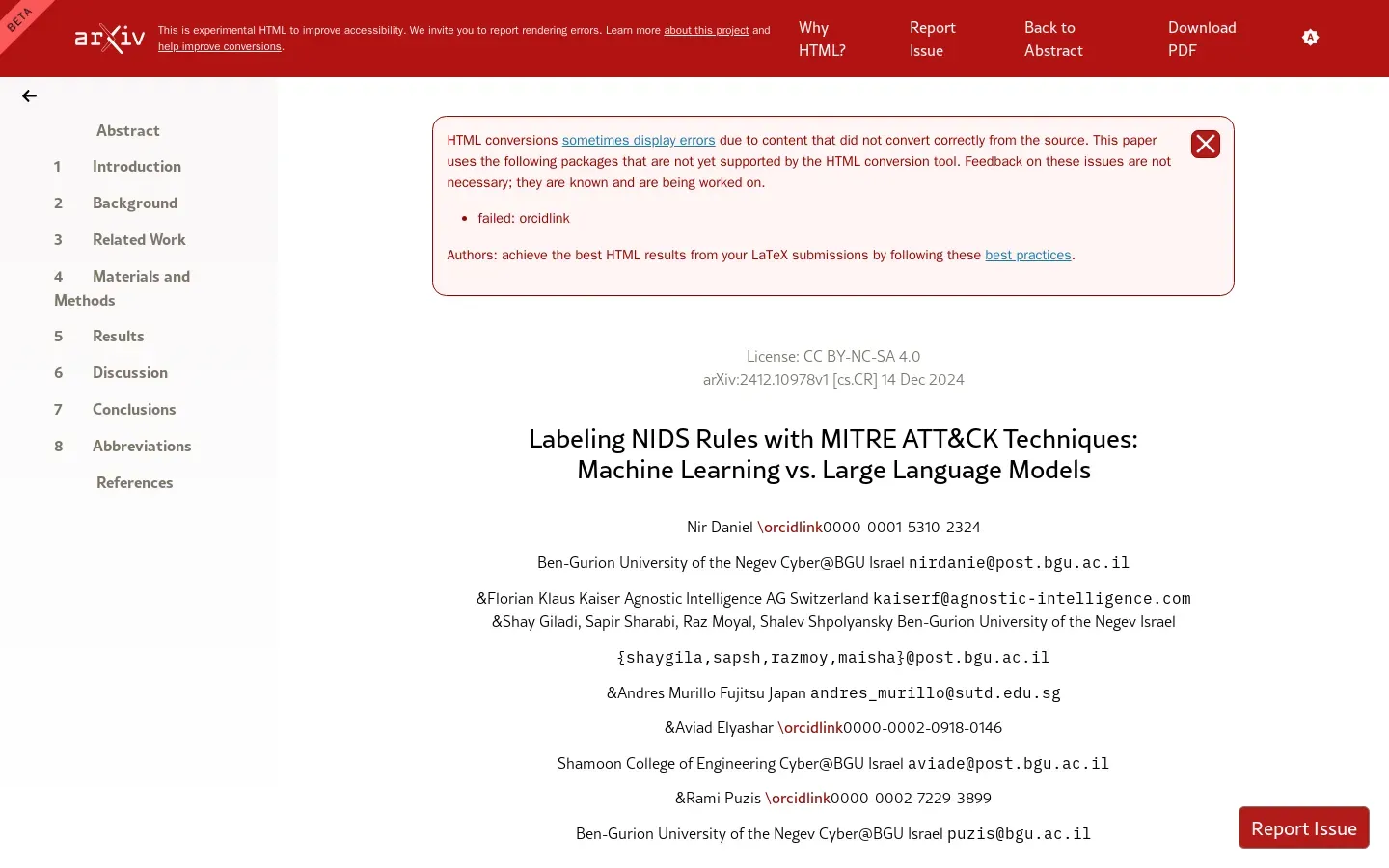