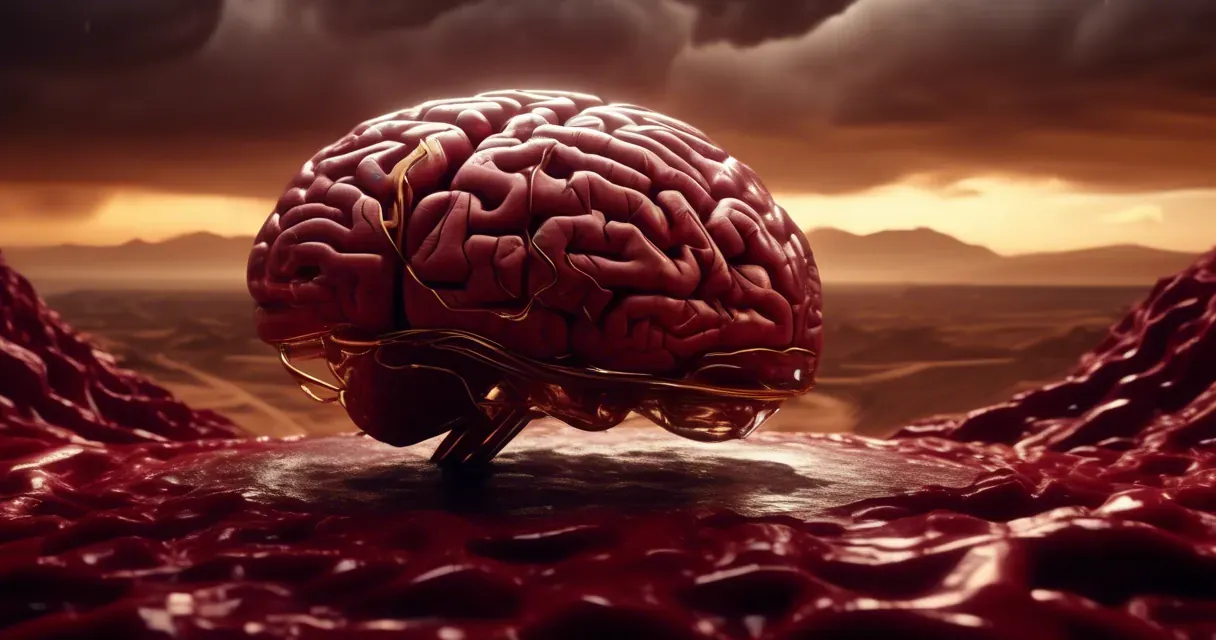
Research Highlights Vulnerabilities in Generative AI Security
/ 4 min read
Quick take - Recent research highlights the vulnerabilities of generative AI to prompt injection techniques and offers recommendations for enhancing cybersecurity through preventive measures, user education, and policy frameworks.
Fast Facts
- Recent research highlights vulnerabilities in generative AI, particularly related to prompt injection techniques, necessitating enhanced cybersecurity measures.
- Key objectives include identifying prompt injection methods, simulating attack scenarios, developing preventive strategies, and implementing monitoring tools.
- Findings emphasize a proactive cybersecurity approach that combines technical solutions with user awareness to mitigate risks effectively.
- The study discusses important frameworks and tools, such as the MITRE ATLAS Matrix and OWASP Top 10, to address security vulnerabilities in AI systems.
- Recommendations include advanced detection mechanisms, user education programs, and the establishment of policy frameworks to govern the use of generative AI in sensitive sectors.
Enhancing Cybersecurity in Generative AI: Key Findings and Recommendations
In an era where generative AI is becoming increasingly integrated into various sectors, recent research has highlighted critical vulnerabilities that could compromise these systems. The study focuses on prompt injection techniques, a method by which attackers can manipulate AI outputs, posing significant risks to both users and organizations. As the use of generative AI expands, understanding and mitigating these vulnerabilities is crucial.
Objectives of the Research
The primary goals of the research were to identify and analyze prompt injection techniques, simulate attack scenarios, develop preventive measures, and implement monitoring tools. By doing so, the study aimed to bolster cybersecurity measures within AI technologies.
- Identify and Analyze Prompt Injection Techniques: The research sought to uncover various methods attackers might use to influence AI-generated content.
- Simulate Attack Scenarios: Hypothetical scenarios were created to evaluate the potential impact of these vulnerabilities and test existing safeguards.
- Develop Preventive Measures: Strategies were formulated to prevent prompt injections from affecting AI outputs.
- Implement Monitoring and Detection Tools: Continuous monitoring tools were established to detect anomalies in AI interactions.
Methodology
The study employed a structured approach to achieve its objectives. It began with a detailed examination of prompt injection techniques, followed by simulations of attack scenarios. This was complemented by the development of preventive strategies and the implementation of monitoring tools designed to safeguard against potential threats.
Key Findings
The findings underscore the necessity for a proactive cybersecurity approach in dealing with generative AI vulnerabilities. Technical solutions alone are insufficient; user awareness plays a pivotal role in mitigating risks effectively. The implications highlight the need for comprehensive strategies that encompass both technological and educational components.
Strengths of the Research
The research is notable for its thorough analysis of prompt injection techniques and its practical approach to developing preventive measures. It emphasizes user education and policy frameworks, offering a holistic view of cybersecurity in generative AI.
Limitations and Areas for Further Investigation
Despite its strengths, the study has limitations, particularly in the range of attack scenarios examined. Further investigation is needed to explore additional attack vectors and assess the effectiveness of proposed measures in real-world applications.
Tools and Frameworks Discussed
Several tools and frameworks were highlighted as essential in addressing security vulnerabilities:
- MITRE ATLAS Matrix: A framework for understanding adversarial techniques targeting AI systems.
- OWASP Top 10: Guidelines outlining critical security risks to web applications, adaptable for AI interfaces.
- Trend Vision One™ ZTSA (Zero Trust Secure Access): A model enforcing strict access controls with continuous verification.
- Prompt Inspection Techniques: Methods developed to scrutinize inputs before processing by AI systems.
Recommendations for Implementation
- Development of Advanced Detection Mechanisms: Organizations should invest in sophisticated systems capable of identifying prompt injection attacks.
- User Education and Awareness Programs: Training initiatives should be implemented to educate users about prompt injection risks and encourage critical evaluation of AI-generated content.
- Policy and Regulatory Frameworks: Collaboration with policymakers is essential to establish guidelines governing generative AI use, especially in sensitive sectors like finance, healthcare, and government.
As generative AI technologies continue to evolve, so too must our approaches to cybersecurity. By integrating technical solutions with user education and robust policy frameworks, stakeholders can better protect against prompt injection risks and ensure the safe deployment of AI systems.
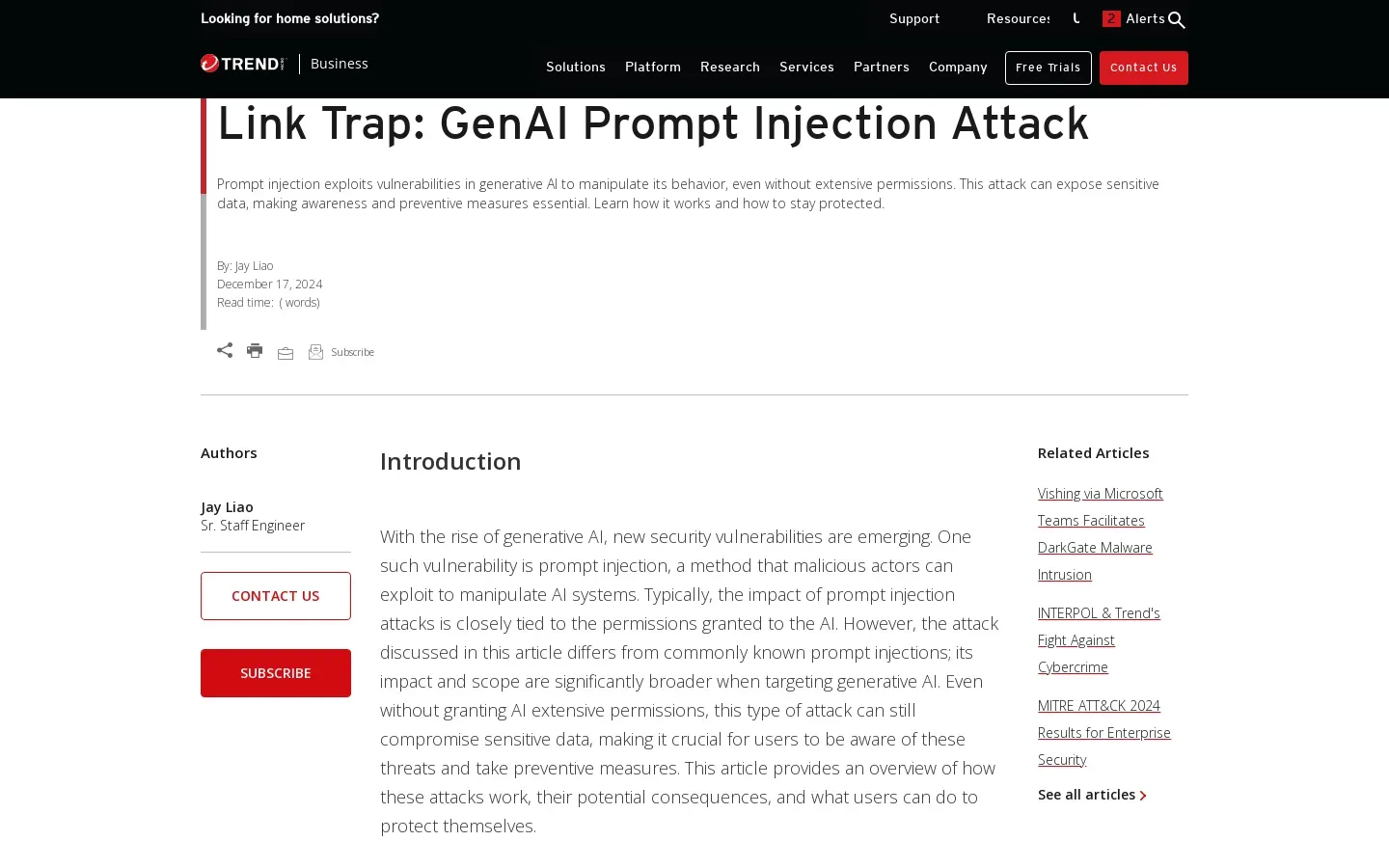