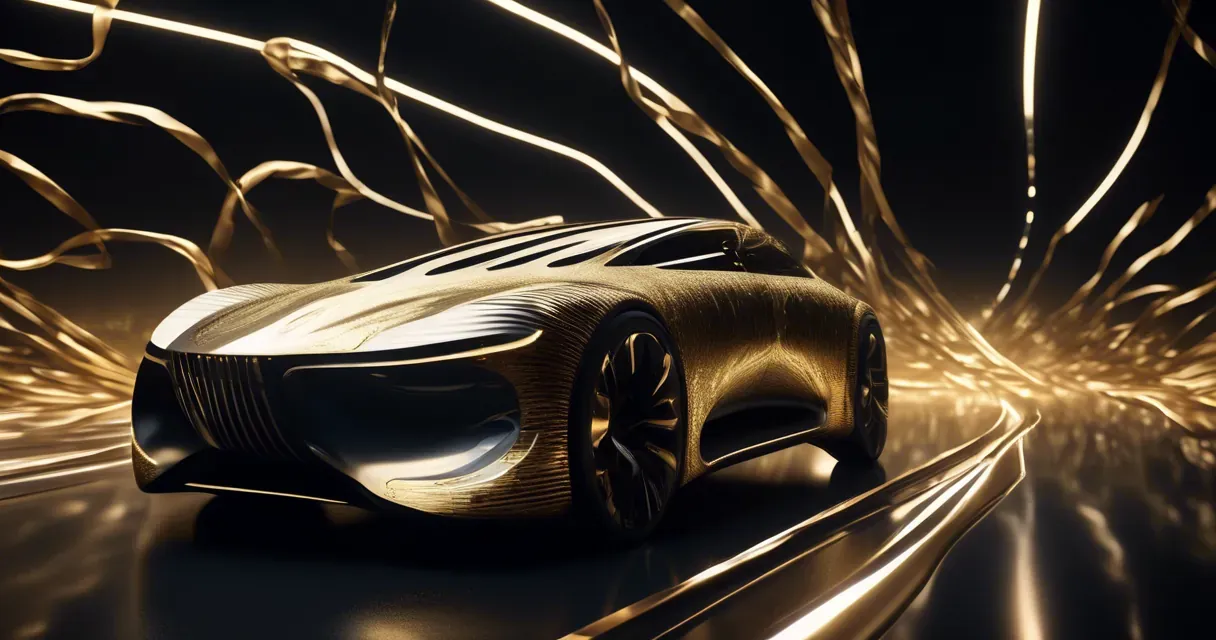
Advancements in GPS Spoofing Detection for Autonomous Vehicles
/ 4 min read
Quick take - Recent research has made significant strides in developing a GPS Intrusion Detection System (GPS-IDS) to enhance the cybersecurity of autonomous vehicles against GPS spoofing attacks, highlighting the importance of advanced detection techniques and the integration of machine learning for improved safety in modern transportation systems.
Fast Facts
- Recent advancements in GPS Intrusion Detection Systems (GPS-IDS) aim to enhance the cybersecurity of autonomous vehicles (AVs) against GPS spoofing attacks.
- Key components of the research include the development of an Autonomous Vehicle Behavior Model, an Anomaly Behavior Analysis module, and robust detection mechanisms for GPS spoofing.
- The GPS-IDS framework has shown substantial effectiveness in detecting spoofing attacks, emphasizing the importance of GPS security in AV operations.
- Future directions include integrating multi-sensor systems, real-time threat intelligence sharing, and collaboration with Vehicle-to-Everything (V2X) technologies for improved security.
- The research highlights the need for ongoing development in cybersecurity as AV technology evolves, advocating for regulatory frameworks to ensure robust protective measures.
Advancements in GPS Intrusion Detection Systems for Autonomous Vehicles
In a significant stride towards bolstering the cybersecurity of autonomous vehicles (AVs), recent research has unveiled advancements in GPS Intrusion Detection Systems (GPS-IDS). These developments aim to fortify AVs against the growing threat of GPS spoofing attacks, a pressing concern as these vehicles become integral to modern transportation networks. The study’s findings underscore the critical need for enhanced security measures to safeguard AV navigation systems.
Key Objectives and Methodology
The research focused on several pivotal objectives, beginning with the development of an Autonomous Vehicle Behavior Model. This model serves as a foundational framework for understanding how AVs operate under various conditions. A crucial component of this initiative is the Anomaly Behavior Analysis (ABA) module, designed to identify unusual patterns in vehicle navigation data that may indicate spoofing attempts.
To achieve these objectives, researchers employed a structured methodology. This included dataset preparation and stratified sampling to ensure a comprehensive analysis of diverse data sets. Machine learning techniques were then utilized to train detection models, followed by rigorous performance evaluations to assess their capabilities. Threshold optimization was also conducted to fine-tune detection accuracy.
Noteworthy Findings
The research yielded several important findings. The GPS-IDS framework demonstrated substantial effectiveness in detecting GPS spoofing attacks, showcasing its potential for real-world application. This highlights the necessity of integrating machine learning into cybersecurity practices to enhance AV safety. The study also emphasizes the importance of focusing on GPS security and risk assessment within autonomous operations.
Implications for the Future
These findings have significant implications for the future of AV technology and cybersecurity. One practical application is the integration of GPS-IDS with other sensor systems, enhancing detection capabilities through multi-sensor fusion. Additionally, establishing networks for real-time threat intelligence sharing among AVs could prove invaluable in mitigating risks.
Adaptive learning mechanisms are another promising avenue, allowing detection systems to continually improve based on new data. Furthermore, there is a pressing need for regulatory and standardization frameworks that ensure robust cybersecurity measures across the industry.
Strengths and Limitations
The research boasts several strengths, including its innovative use of machine learning and comprehensive approach to anomaly detection. However, it also faces limitations. There is a need for further exploration of advanced attack detection techniques and strategies for real-time deployment in diverse environments.
Future Directions
Looking ahead, researchers recommend pursuing several avenues to enhance AV security and reliability further:
- Integration with Multi-Sensor Fusion Systems: This would improve detection accuracy by combining data from various sources.
- Real-Time Deployment Capabilities: Implementing these systems in edge computing environments could facilitate quicker responses.
- Development of Digital Twins: These virtual models would allow for extensive simulation and testing.
- Collaboration with V2X Communication Technologies: Such partnerships could enable seamless threat response across connected vehicle networks.
By addressing vulnerabilities related to GPS spoofing, this initiative not only enhances AV safety but also contributes to creating secure transportation systems in an increasingly complex technological landscape. The findings are expected to guide future research and development efforts within this critical field.
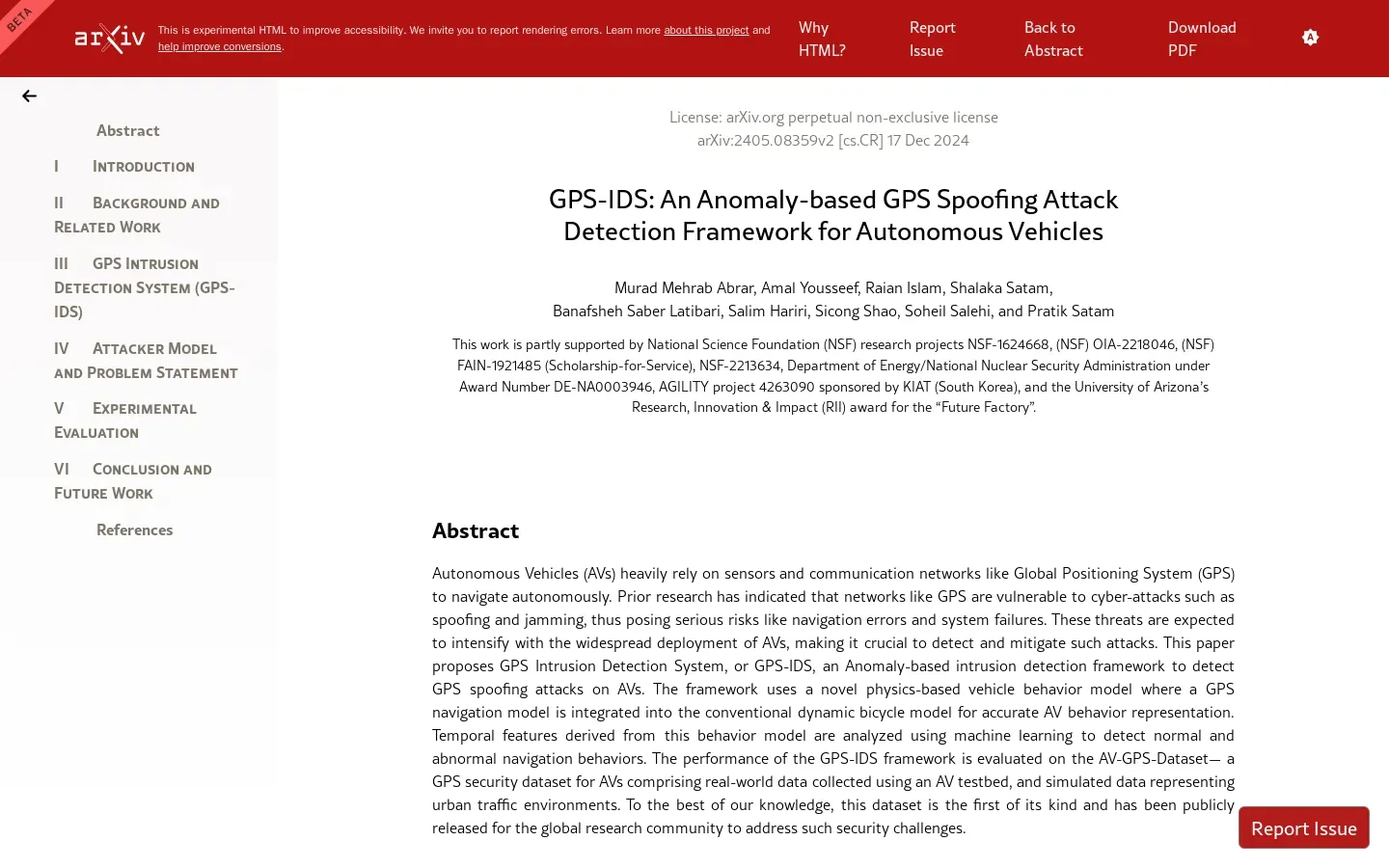