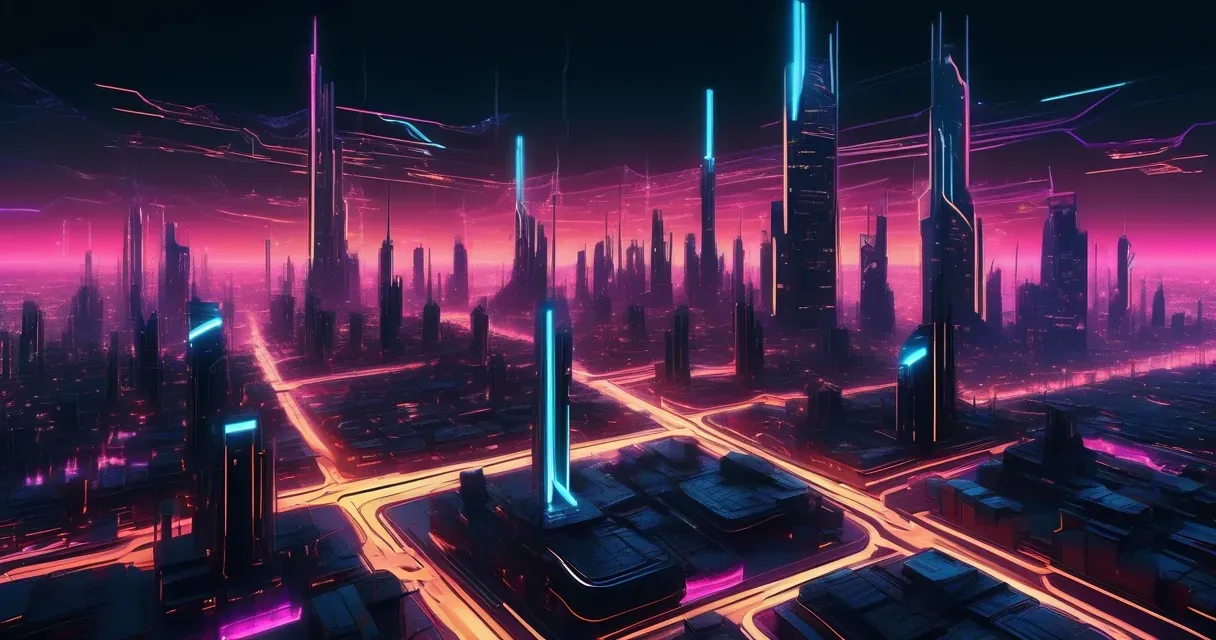
New Framework Enhances Intrusion Detection Systems
/ 4 min read
Quick take - Recent research on the Disentangled Dynamic Intrusion Detection System with Multi-scale Few-shot Learning (DIDS-MFL) has introduced new methodologies aimed at improving the accuracy and reliability of intrusion detection systems in dynamic network environments.
Fast Facts
- Innovative Framework: The Disentangled Dynamic Intrusion Detection System with Multi-scale Few-shot Learning (DIDS-MFL) enhances IDS accuracy by addressing entangled traffic features and dynamic network environments.
- Key Methodologies: The approach includes statistical disentanglement, dynamic graph diffusion, and historical memory to improve detection of attack-specific features and facilitate real-time analysis.
- Improved Detection Capabilities: DIDS-MFL shows enhanced detection of unknown attacks, reduced false positives, and dynamic feature representation, leading to greater reliability in threat identification.
- Strengths and Limitations: While the framework offers significant advancements in few-shot learning and adaptability, challenges remain in scalability and integration with existing security systems.
- Future Directions: Research suggests integrating DIDS-MFL with IoT security, real-time threat intelligence sharing, and cross-domain applications to further enhance cybersecurity measures.
Advancements in Intrusion Detection Systems: A New Era of Cybersecurity
In a significant stride towards bolstering cybersecurity, recent research has unveiled the Disentangled Dynamic Intrusion Detection System with Multi-scale Few-shot Learning (DIDS-MFL). This innovative framework aims to enhance the accuracy and reliability of intrusion detection systems (IDS), addressing the persistent challenges posed by entangled traffic features and dynamic network environments.
Key Methodologies
The DIDS-MFL framework introduces a systematic approach to intrusion detection, leveraging advanced techniques such as statistical disentanglement and dynamic graph diffusion. These methodologies are designed to refine the detection process and adapt to evolving threats in real-time.
Statistical Disentanglement
At the core of this approach is statistical disentanglement, which focuses on isolating attack-specific features. By reducing the impact of entangled distributions within traffic data, this method enhances the model’s ability to concentrate on relevant threat indicators, thereby improving detection precision.
Dynamic Graph Diffusion
Dynamic graph diffusion plays a crucial role in facilitating real-time analysis of network conditions. This technique allows for adaptive responses to threats as they emerge, ensuring that the system remains vigilant and responsive to new attack vectors.
Historical Memory and Message Generation
To support informed decision-making, the system incorporates historical memory and message generation. This ensures that crucial past data is retained and utilized effectively, providing context for current threat assessments.
Node Memory Update and Representation Generation
Enhancing the system’s capability further, node memory update and representation generation processes enable the creation of dynamic feature representations. These are vital for maintaining high detection accuracy across diverse network scenarios.
Multi-Layer Graph Diffusion
Finally, multi-layer graph diffusion promotes efficient communication between nodes within a network. This comprehensive threat analysis capability is essential for identifying complex attack patterns that may otherwise go unnoticed.
Key Findings
The implementation of the DIDS-MFL framework has yielded several noteworthy outcomes:
- Enhanced Detection of Unknown and Few-Shot Attacks: The system excels in identifying previously unseen attacks, a critical feature for adapting to new cyber threats.
- Dynamic Feature Representation: Real-time updates of feature representations through dynamic graph techniques significantly improve detection accuracy.
- Reduction of False Positives: A marked decrease in false alarms enhances the system’s reliability, addressing a common issue in traditional IDS.
- Foundation for Future Research: The findings lay groundwork for further exploration into advanced cybersecurity methodologies.
Strengths and Limitations
While DIDS-MFL presents substantial advancements, it also highlights areas requiring further investigation. Its strengths lie in its innovative approach to few-shot learning and adaptability to dynamic environments. However, challenges remain in terms of scalability and integration with existing security frameworks.
Recommended Tools and Techniques
The research underscores several tools and frameworks poised to elevate IDS capabilities:
- Disentangled Intrusion Detection System (DIDS): Focuses on disentangling traffic features for improved detection.
- Multi-scale Few-shot Learning (MFL): Effective in data-limited environments.
- Graph Diffusion Methods: Facilitate real-time communication and analysis within networks.
- Non-parametric Optimization: Enhances system efficiency and adaptability.
Future Directions
The implications of this research extend beyond immediate applications in intrusion detection. Potential future directions include:
- Integration with IoT Security Frameworks: Enhancing security measures for Internet of Things (IoT) devices.
- Real-time Threat Intelligence Sharing: Promoting collaborative security efforts across networks.
- Cross-Domain Applications: Applying findings to various cybersecurity challenges such as malware detection and user behavior analytics.
As cybersecurity continues to evolve, the methodologies introduced by DIDS-MFL offer promising avenues for developing more resilient defenses against sophisticated cyber threats. The ongoing exploration of these techniques will be crucial in fortifying digital infrastructures against potential intrusions.
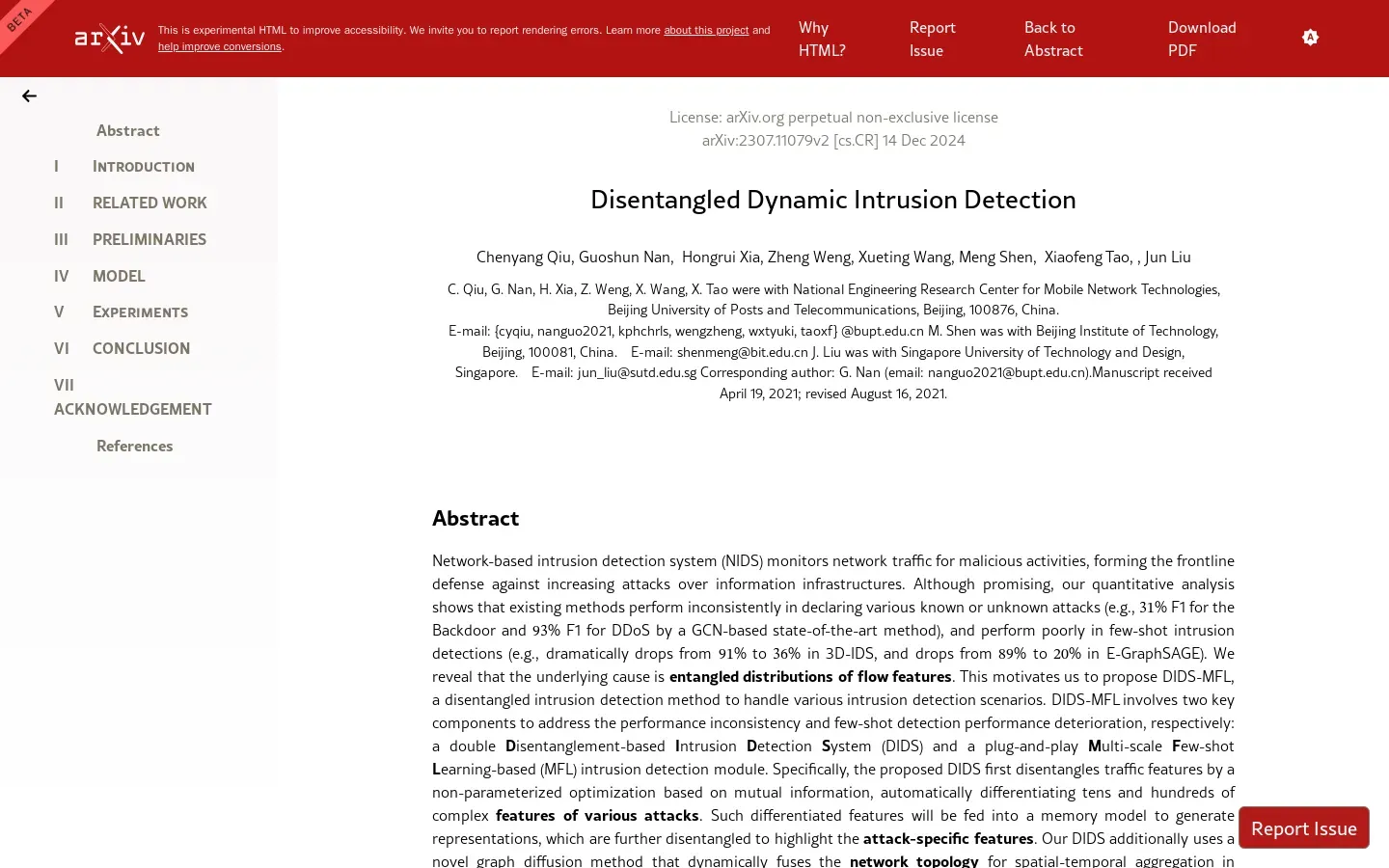