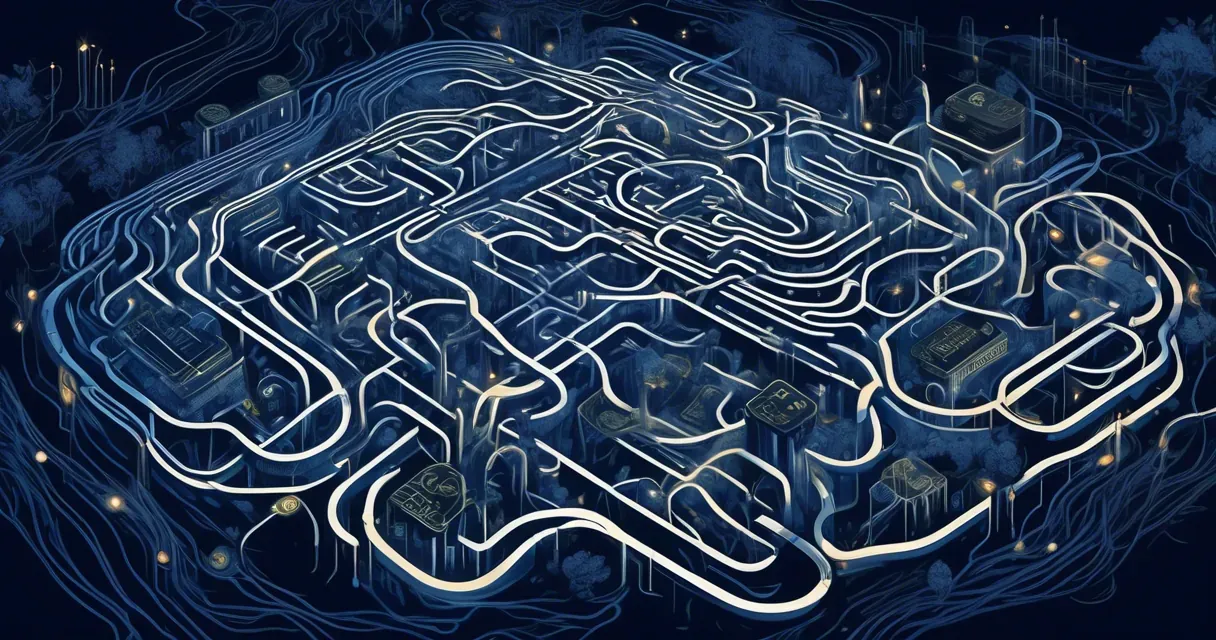
AI Techniques in Cybersecurity: Benefits and Challenges Explored
/ 4 min read
Quick take - Recent research highlights the integration of artificial intelligence and deep learning in cybersecurity frameworks, revealing both significant benefits in threat detection and challenges related to resource requirements and transparency.
Fast Facts
- Enhanced Threat Detection: AI and deep learning significantly improve real-time identification and response to cyber threats through data analysis.
- Resource and Infrastructure Challenges: Implementing advanced AI technologies requires substantial computational resources, posing challenges for many organizations.
- Need for Transparency: Greater transparency and clear evaluation metrics are essential for assessing the effectiveness of AI-driven cybersecurity solutions.
- Future Research Directions: Opportunities for further exploration include developing open-source frameworks and integrating federated learning for continuous threat detection.
- Key Tools: Notable tools discussed include NVIDIA Morpheus, Vectra AI, Check Point Quantum R81, and Long Short-term Memory (LSTM) networks for anomaly detection.
Advancements and Challenges of AI Techniques in Cybersecurity Frameworks
In the rapidly evolving landscape of cybersecurity, artificial intelligence (AI) and deep learning techniques are emerging as pivotal tools for enhancing threat detection and response. Recent research has delved into the integration of these technologies within cybersecurity frameworks, offering a comprehensive analysis of their potential benefits and inherent challenges. This study provides critical insights into how AI can transform cybersecurity measures while also highlighting areas that require further exploration.
Enhanced Threat Detection Capabilities
One of the most significant findings of the research is the enhanced threat detection capabilities offered by AI and deep learning. These technologies enable systems to analyze vast datasets in real time, facilitating quicker and more accurate identification of cyber threats. By leveraging AI, organizations can proactively manage threats, reducing the risk of breaches and minimizing potential damage. This capability is particularly crucial as cyber threats become more sophisticated and frequent.
Resource Requirements and Infrastructure Challenges
Despite the promising advancements, the implementation of AI-driven cybersecurity solutions is not without its challenges. The research underscores the substantial computational resources and robust infrastructure required to deploy these advanced technologies effectively. Many organizations may find these requirements daunting, potentially limiting their ability to fully integrate AI into their cybersecurity frameworks. Addressing these challenges is essential for broader adoption and effectiveness.
Transparency and Evaluation of Cybersecurity Solutions
The study also highlights the need for greater transparency in AI-driven cybersecurity solutions. There is a pressing demand for clear evaluation metrics to assess the effectiveness and reliability of these systems. Without standardized metrics, it becomes challenging to gauge the true impact of AI on cybersecurity measures. This lack of transparency can hinder trust and slow down the adoption of innovative solutions.
Future Research Directions
The research identifies several promising avenues for future exploration. Among them is the development of open-source cybersecurity frameworks that could democratize access to advanced security tools. Additionally, integrating federated learning—a technique that allows models to be trained across decentralized devices—could enhance continuous threat detection without compromising data privacy. These directions offer exciting possibilities for advancing cybersecurity practices.
Tools and Frameworks in Focus
Several tools and frameworks were discussed in the research, each contributing uniquely to the AI-driven cybersecurity landscape:
-
NVIDIA Morpheus: This framework streamlines building and deploying AI applications for cybersecurity, enhancing operational efficiency.
-
Vectra AI: Utilizes AI to detect and respond to cyber threats in real time, providing a proactive approach to threat management.
-
Check Point Quantum Cyber Security Platform R81: A comprehensive solution leveraging advanced threat prevention techniques, including AI.
-
Long Short-term Memory (LSTM): A recurrent neural network effective for time series prediction and anomaly detection in cybersecurity contexts.
Implications for Practice and Theory
The implications of these findings are multifaceted. Practically, they underscore the convergence of AI with cybersecurity, offering a foundation for understanding how deep learning can bolster security measures. Theoretically, they highlight limitations such as the need for robust datasets and real-time threat intelligence tools. Addressing these gaps could significantly enhance the efficacy of AI-driven solutions.
As we navigate an era where cyber threats are increasingly complex, integrating AI and deep learning into cybersecurity frameworks presents both opportunities and challenges. Organizations must consider resource requirements, transparency issues, and infrastructure needs to fully leverage these technologies’ potential. This research serves as a catalyst for ongoing discussions in the cybersecurity domain, encouraging further exploration and innovation to address these pressing challenges.