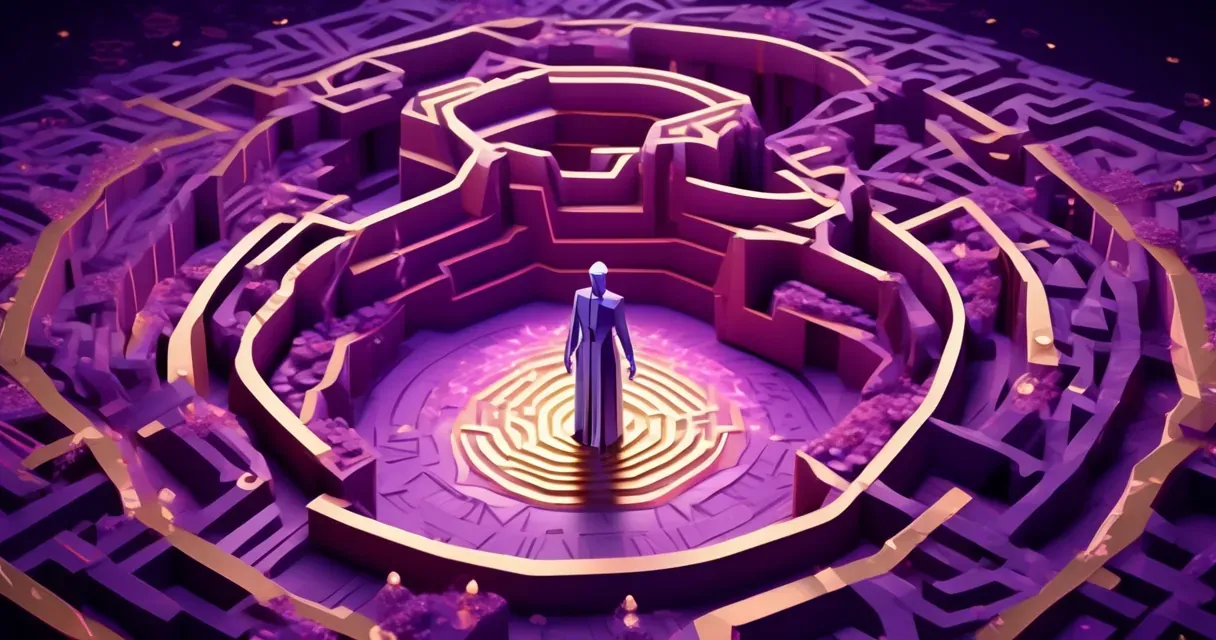
Enhancing Security Measures for AI Agents
/ 4 min read
Quick take - recent research has identified vulnerabilities in AI agents and proposed defense mechanisms to enhance their security, highlighting the importance of a proactive approach to cybersecurity as AI technologies continue to evolve.
Fast Facts
- Recent research identifies vulnerabilities in AI agents and proposes tailored defense mechanisms to enhance their security.
- Key defense strategies include session management, sandboxing, format-preserving encryption techniques, and prompt tuning.
- The study emphasizes the importance of session-aware AI models and a security-centric approach in AI development.
- Future directions include developing robust security frameworks, real-time monitoring systems, and privacy-preserving techniques.
- Practical applications of the findings aim to improve personalized cybersecurity, secure data handling, threat detection, and regulatory compliance.
Enhancing Security of AI Agents: Key Findings and Future Directions
Recent research has shed light on the vulnerabilities within artificial intelligence (AI) systems, offering new insights into enhancing their security. As AI technologies become increasingly integral to various applications, understanding these vulnerabilities and implementing effective defense mechanisms is crucial for the future of cybersecurity.
Identifying Vulnerabilities in AI Systems
The study conducted a systematic analysis to identify potential weaknesses in AI agent systems. Researchers focused on areas where security could be compromised, highlighting the need for robust protection measures. This comprehensive approach ensures that vulnerabilities are not only identified but also understood in the context of their potential impact.
Proposed Defense Mechanisms
In response to the identified vulnerabilities, the study introduced several tailored defense strategies:
-
Session Management: By ensuring that AI agents can effectively manage user sessions, security is significantly enhanced. This approach helps in maintaining the integrity of interactions between users and AI systems.
-
Sandboxing: Implementing controlled environments allows AI agents to operate without risking system integrity. Sandboxing confines the actions of AI agents, preventing them from accessing sensitive resources unless explicitly permitted.
-
Format-Preserving Encryption Techniques (FPETS): These techniques protect sensitive data while maintaining its usability, ensuring that data remains secure without hindering its functionality.
-
Prompt Tuning: Optimizing AI responses reduces the risk of exploitation by ensuring that outputs are consistent with security protocols.
Key Findings
The research highlights critical insights that bridge AI development and cybersecurity:
-
Session-Aware AI Models: The study advocates for AI models that are aware of user sessions. Such models can learn from interactions while safeguarding privacy, offering a balance between personalization and security.
-
Security-Centric Analysis: Emphasizing a security-centric approach in AI development, the findings suggest balancing performance enhancement with robust security measures.
Implications for Cybersecurity
The implications of these findings underscore the necessity for a proactive cybersecurity approach in AI contexts:
-
Reevaluation of Confidentiality Protocols: Organizations must reassess their data confidentiality measures to address AI-specific vulnerabilities.
-
Integrity Management in AI Interactions: Ensuring data integrity during exchanges between AI agents and users is paramount to maintaining trust and security.
-
Sandboxing and Resource Access Control: Implementing sandboxing techniques is essential for controlling resource access by AI agents, thereby minimizing risk.
Strengths and Limitations
The research excels in thoroughly examining AI vulnerabilities and developing actionable defense strategies. However, it acknowledges limitations such as the need for further investigation into the scalability of proposed solutions and practical challenges in real-world applications.
Future Directions
Looking ahead, several promising avenues for future exploration in AI agent security are outlined:
-
Development of Robust Security Frameworks: Creating comprehensive frameworks to address unique challenges posed by AI agents.
-
Real-Time Monitoring and Anomaly Detection Systems: Implementing systems capable of detecting and responding to anomalies in real-time.
-
Privacy-Preserving Techniques: Exploring methods to handle sensitive data without compromising user privacy.
-
Cross-Domain Applications and Interoperability: Investigating how security measures can be applied across different AI domains to ensure interoperability and safety.
Applications
The insights from this research pave the way for practical applications:
-
Personalized Cybersecurity Assistants: Utilizing AI to create tailored security solutions for individuals and organizations.
-
Secure Data Handling in Multi-User Environments: Developing strategies to ensure data security in collaborative settings.
-
Enhanced Threat Detection and Response: Leveraging AI capabilities to bolster threat detection systems.
-
Regulatory Compliance and Auditing: Ensuring that AI systems adhere to regulatory standards and undergo regular auditing for security compliance.
As these findings continue to influence cybersecurity practices, stakeholders must remain vigilant in adapting to emerging threats while leveraging technological advancements to safeguard AI systems effectively.
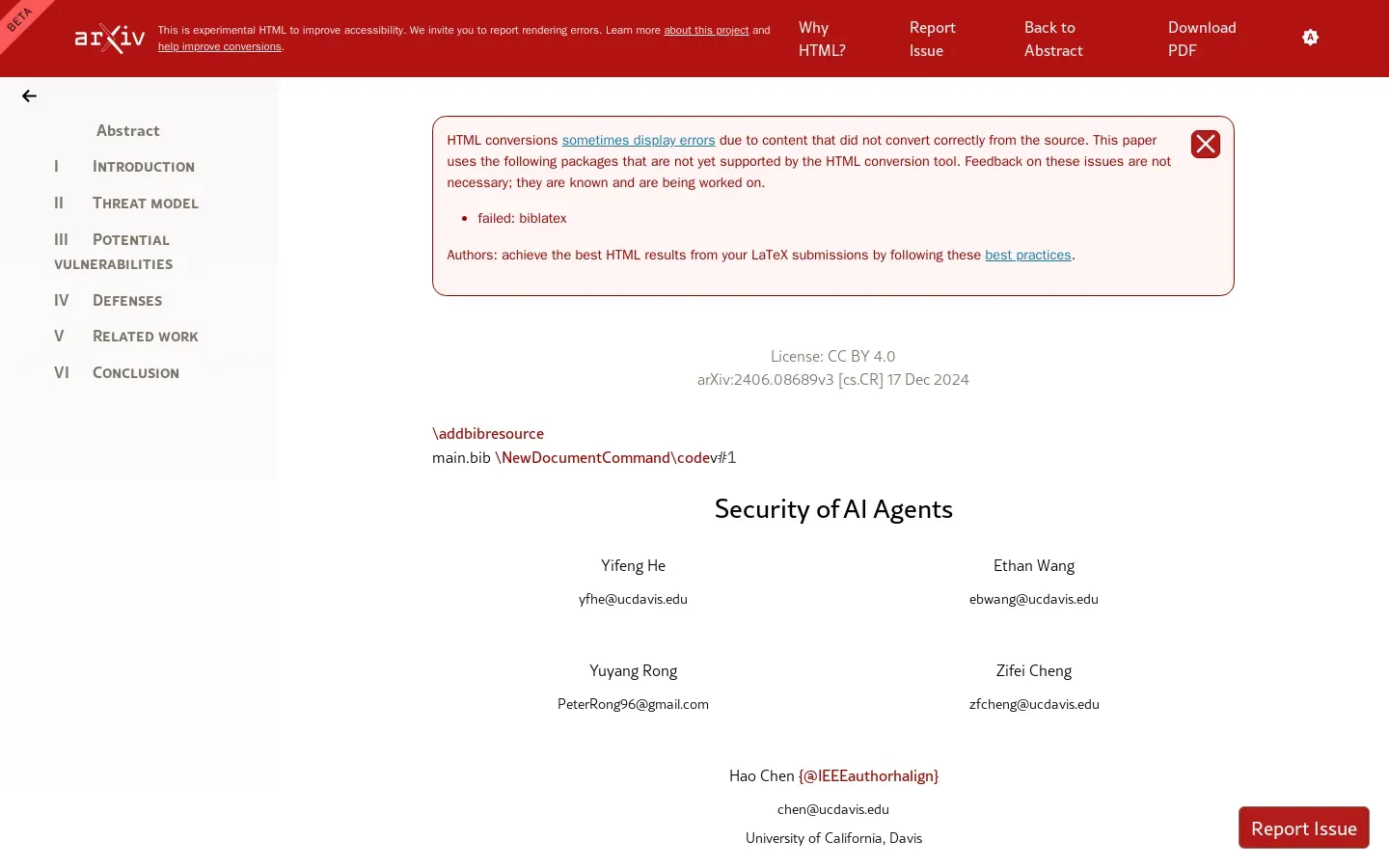