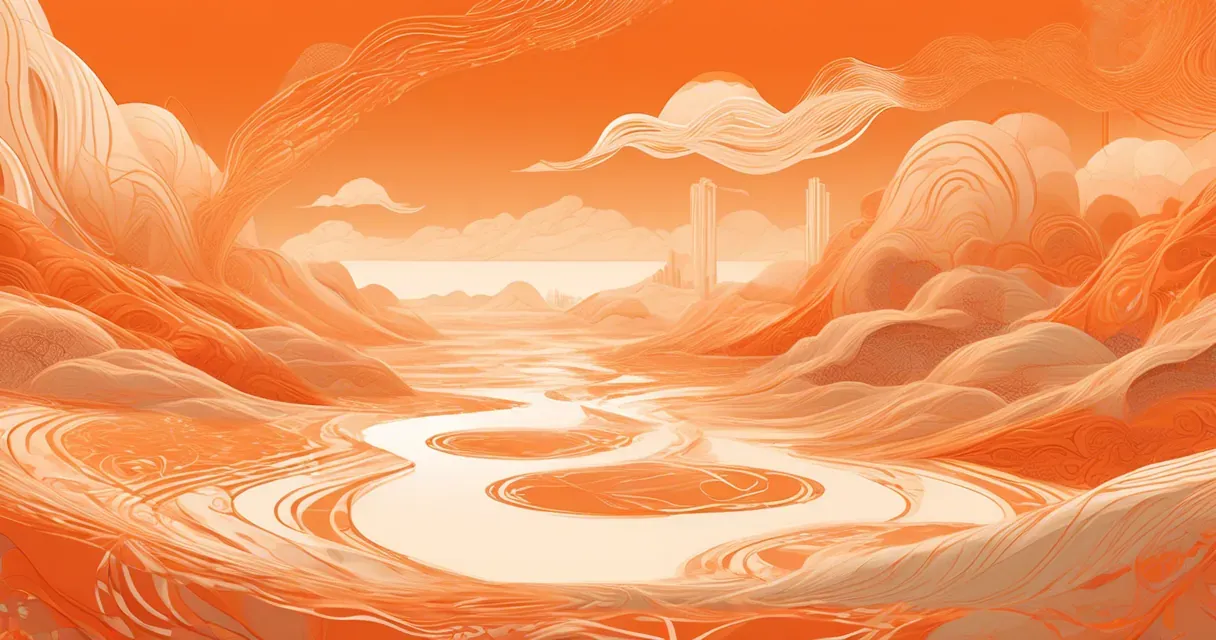
Impact of Flow Exporters on Intrusion Detection Systems
/ 4 min read
Quick take - Wu et al. (2022) highlight advancements in intrusion detection techniques that improve the accuracy and efficiency of network security systems, focusing on data quality, feature extraction, model training, and performance analysis in cloud environments.
Fast Facts
- Focus on Data Quality: The study emphasizes the critical importance of high-quality datasets for effective machine learning in intrusion detection systems (IDS).
- Utilization of HERA: The Holistic nEtwork featuRes Aggregator (HERA) is employed for efficient feature extraction from network flow data, aiding in anomaly detection.
- Machine Learning Models: Various models, including Random Forest and XGBoost, are tested to enhance detection capabilities, with flow exporters significantly impacting performance.
- Need for Standardization: The research highlights the necessity for standardized feature sets to improve benchmarking and comparability across different IDS.
- Real-World Applicability: The findings suggest that the advancements can enhance real-time IDS effectiveness in cloud environments and advocate for integration with threat intelligence platforms.
Advancements in Intrusion Detection: A Closer Look at Wu et al. (2022)
In the rapidly evolving landscape of cybersecurity, the study by Wu et al. (2022) marks a significant step forward in enhancing intrusion detection techniques. This research focuses on refining the accuracy and efficiency of network security systems, particularly within cloud environments. By systematically addressing data collection, feature extraction, model training, and performance analysis, the study aims to bolster real-time intrusion detection systems (IDS).
Key Research Objectives
The study sets out with clear objectives to improve IDS capabilities:
Data Collection and Preparation
A cornerstone of the research is the emphasis on high-quality data. The study argues that the effectiveness of machine learning models in detecting intrusions hinges on robust datasets. Poor data quality can lead to unreliable detection outcomes, underscoring the need for meticulous data preparation.
Flow Generation and Feature Extraction Using HERA
The researchers employed the Holistic nEtwork featuRes Aggregator (HERA) to streamline the process of extracting and aggregating network flow data. This tool is pivotal in identifying anomalies within network traffic, serving as a foundation for effective intrusion detection.
Model Training and Evaluation
A variety of machine learning models were tested, including Random Forest, XGBoost, LightGBM, and Explainable Boosting Machine. These models were used to analyze extracted features, aiming to enhance detection capabilities through rigorous training and evaluation processes.
Performance Analysis and Results Interpretation
The study’s performance analysis revealed that flow exporters like Argus and BRO-IDS/Zeek significantly impact model efficiency. This finding highlights the importance of selecting optimal flow exporters for real-world applications.
Key Findings
Several critical insights emerged from the research:
-
Data Quality: High-quality data is essential for effective intrusion detection. The study stresses that poor data quality can compromise model reliability.
-
Flow Exporters’ Impact: The choice of flow exporters plays a crucial role in model performance, necessitating careful selection to maximize efficiency.
-
Standardization Needs: There is a call for standardized feature sets to enable benchmarking and comparability across different IDS platforms.
-
Enhanced Detection: Improved datasets contribute to better accuracy and efficiency in identifying network anomalies, thereby strengthening security measures.
Strengths and Limitations
The study’s strengths lie in its applicability across various network security environments, making it suitable for deployment in diverse settings. However, it also acknowledges limitations such as the need for further exploration into scalability and effectiveness under varying network conditions.
Tools and Techniques
The research explores several tools integral to IDS development:
-
HERA: Essential for feature extraction, enabling comprehensive network flow analysis.
-
Flow Exporters: Argus and Zeek are highlighted for their role in collecting and exporting network flow data.
-
Machine Learning Models: The versatility of algorithms like Random Forest and XGBoost showcases their potential in optimizing detection accuracy.
-
PCAP Files: Utilized for analyzing network traffic, these files provide critical data for training and evaluating models.
Implications for Cybersecurity
The findings from Wu et al. (2022) carry significant implications:
-
Real-Time IDS in Cloud Environments: The techniques developed can enhance intrusion detection effectiveness in cloud settings, addressing unique challenges posed by cloud computing.
-
Integration with Threat Intelligence Platforms: The study advocates integrating advanced detection methods with existing threat intelligence systems to strengthen security measures.
-
Development of Standardized Datasets: Standardized datasets are crucial for benchmarking and improving comparability across various IDS platforms.
As cybersecurity threats continue to evolve, these advancements underscore the importance of ongoing research and development in intrusion detection technologies. For practitioners and organizations alike, staying informed about such innovations is vital to maintaining robust security postures in an increasingly digital world.