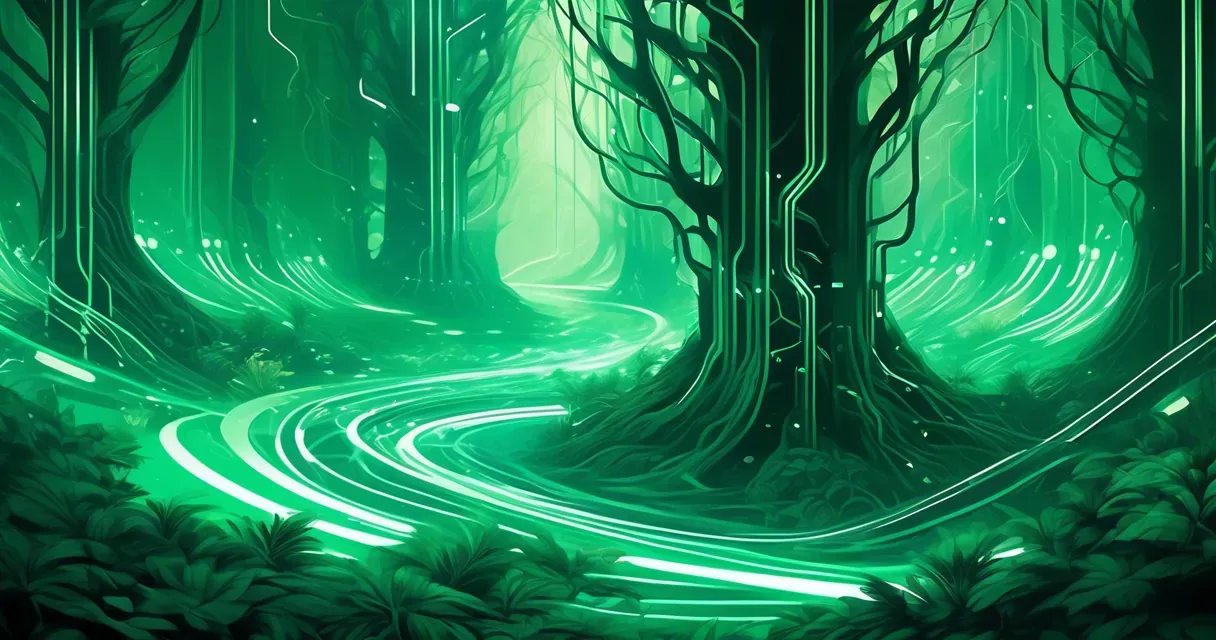
SLIFER Architecture Enhances Malware Detection Performance and Robustness
/ 4 min read
Quick take - Recent research on the SLIFER architecture has demonstrated significant improvements in malware detection accuracy and robustness against adversarial attacks, emphasizing the importance of model calibration and setting a foundation for future advancements in cybersecurity methodologies.
Fast Facts
- SLIFER Architecture: Introduces a sequential malware detection pipeline that enhances detection accuracy and robustness against adversarial attacks through intelligent feature extraction and recognition.
- Calibration Impact: The study emphasizes the importance of calibrating decision thresholds, significantly affecting detection metrics like True Positive Rate (TPR) and False Positive Rate (FPR).
- Robust Methodology: Incorporates error handling and rigorous testing against adversarial attacks, validating SLIFER’s effectiveness compared to existing malware detection models.
- Future Directions: Suggests integration with threat intelligence platforms, adaptive learning mechanisms, and cross-platform detection to improve real-time threat response and adaptability.
- Research Limitations: Identifies the need for improved temporal analysis and deeper exploration of adversarial attack defenses to strengthen the SLIFER architecture.
Advancements in Malware Detection with SLIFER Architecture
In the rapidly evolving field of cybersecurity, the development of innovative methodologies to combat malware threats is crucial. Recent research on SLIFER (Sequential Learning for Intelligent Feature Extraction and Recognition) has highlighted significant advancements in detection accuracy and robustness against adversarial attacks. This study not only underscores the importance of model calibration but also lays the groundwork for future innovations in malware detection systems.
Overview of SLIFER Architecture
The SLIFER research primarily aimed to explore how calibration impacts decision thresholds for pre-trained models, enhancing detection performance and robustness against sophisticated malware threats. The study employed a comprehensive methodology that included developing a sequential analysis pipeline, threshold calibration, and performance evaluation against established malware detection models.
Methodology and Approach
Development of SLIFER Architecture
The research began with creating an end-to-end model focusing on intelligent feature extraction and recognition. This approach aimed to improve the model’s ability to identify and classify malware accurately.
Error Handling and Sample Classification
A robust error handling strategy was integrated into the architecture, enhancing its capability to classify samples accurately even in challenging scenarios. This feature ensures that the model remains effective under various conditions.
Robustness Evaluation Against Adversarial Attacks
The study rigorously tested SLIFER’s resilience by simulating adversarial attacks, ensuring its effectiveness in real-world applications. This evaluation is crucial for understanding how well the model can withstand attempts to deceive it.
Extensive Experimental Analysis
A thorough evaluation of SLIFER’s performance was conducted, comparing it with state-of-the-art approaches and hybrid models. This analysis validated its superiority in terms of detection accuracy and robustness.
Key Findings and Implications
The findings revealed that calibrating decision thresholds significantly influences detection metrics such as True Positive Rate (TPR), False Positive Rate (FPR), and F1-score across various datasets. Additionally, the research highlighted the nuanced role of dynamic analysis and the effectiveness of hybrid detection strategies. These insights emphasize the necessity for continuous innovation in malware detection methodologies, focusing on balancing detection accuracy, resource efficiency, and robustness against evolving threats.
Practical and Theoretical Implications
- Adaptive Detection Systems: There’s a need for systems that can evolve with the changing landscape of cybersecurity threats.
- Robust Error Handling: Integrating robust error handling and calibration strategies is essential in malware detection frameworks.
Strengths of the Research
The research successfully demonstrates SLIFER’s potential through its comprehensive approach, innovative architecture, and rigorous evaluation against existing models. The integration of dynamic analysis and error handling further enhances its applicability in real-world scenarios.
Limitations and Areas for Further Investigation
Despite its strengths, the research identifies areas for further exploration, including improved temporal analysis and concept drift mitigation techniques. Additionally, deeper investigations into adversarial attack development and defense mechanisms are necessary to fortify the SLIFER architecture.
Recommended Tools and Techniques
The study discusses several tools and frameworks integral to the SLIFER architecture:
- SLIFER: The primary model focusing on intelligent feature extraction and recognition.
- Quo.Vadis: A framework for evaluating malware variants.
- MalConv: A convolutional neural network model tailored for malware detection.
- YARA Rules: A tool for identifying and classifying malware samples based on specific patterns.
Future Directions and Applications
The research outlines several promising directions for the SLIFER architecture:
- Integration with Threat Intelligence Platforms: Enhancing real-time threat detection capabilities.
- Adaptive Learning Mechanisms: Allowing SLIFER to learn from new data and adapt to emerging threats.
- Cross-Platform Malware Detection: Extending SLIFER’s applicability across various operating systems and environments.
- User Behavior Analytics (UBA) Integration: Incorporating UBA could provide additional context for detecting anomalous activities related to malware threats.
As cybersecurity threats continue to evolve, these advancements in malware detection highlight the critical need for adaptive technologies like SLIFER that can respond effectively to new challenges.