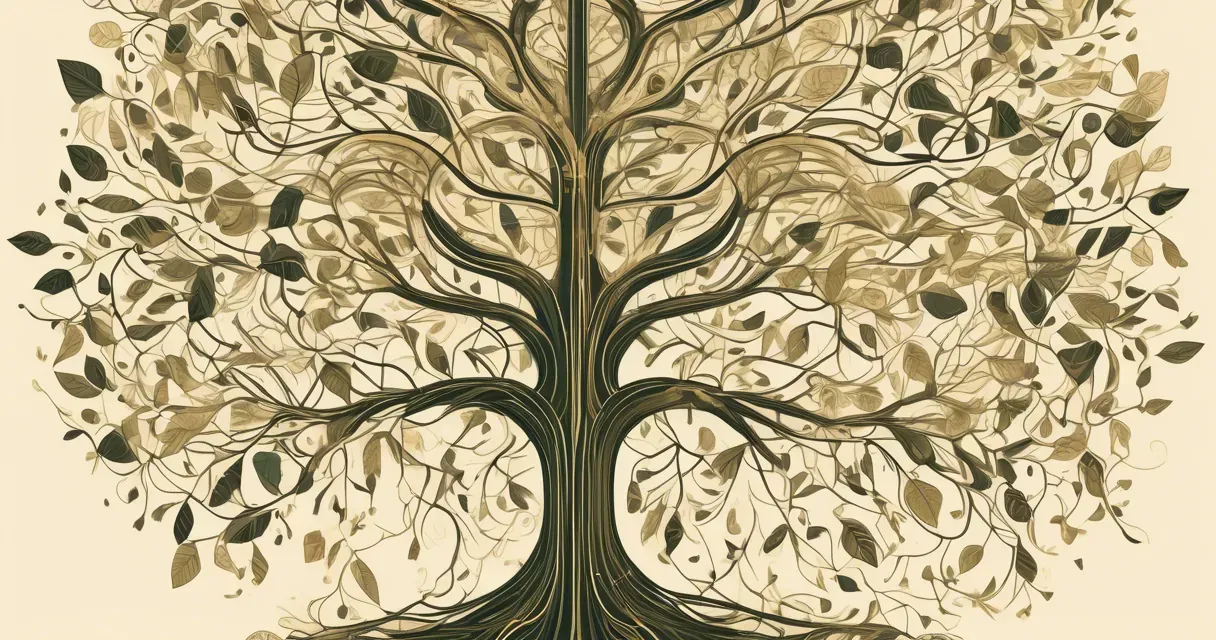
Taxonomy Developed for Reporting AI Security Incidents
/ 4 min read
Quick take - Recent research has produced a position paper that outlines the vulnerabilities and security incidents associated with artificial intelligence, proposing new frameworks and guidelines to enhance cybersecurity practices in response to the unique challenges posed by AI technologies.
Fast Facts
- Recent research has produced a position paper addressing security incidents related to AI, highlighting vulnerabilities and the need for adaptive cybersecurity strategies.
- A multi-faceted methodology was used, including expert consultations, taxonomy development, and testing against existing incident databases.
- Key findings emphasize the identification of AI vulnerabilities, the creation of tailored incident management frameworks, and insights from simulated attacks.
- The study calls for evolving cybersecurity practices, establishing standardized incident reporting frameworks, and utilizing tools like the CVE Program and MITRE ATT&CK Framework.
- Future directions include enhancing security for autonomous systems, creating comprehensive reporting frameworks, and developing prevention mechanisms for AI model theft.
Advancements in AI Security Incident Research: A Comprehensive Overview
In a rapidly evolving technological landscape, the intersection of artificial intelligence (AI) and cybersecurity has become a focal point for researchers and industry experts. A recent position paper sheds light on the security incidents related to AI, offering a structured approach to understanding and mitigating potential risks. This research is pivotal as it addresses growing concerns about AI vulnerabilities, emphasizing the need for adaptive strategies tailored to the unique challenges posed by these systems.
Methodology: A Multi-Faceted Approach
The research employed a robust methodology designed to ensure comprehensive and relevant findings. It began with expert consultation and feedback collection, engaging industry leaders to gather insights and validate preliminary findings. This was followed by an iterative refinement of the taxonomy, where a classification system for AI incidents was developed through continuous feedback loops.
To test the applicability of this taxonomy, researchers utilized existing incident databases for tagging and testing. The final step involved a broader expert review, ensuring that the research framework and findings were thoroughly vetted by a wider pool of experts before finalization.
Key Findings: Vulnerabilities and Frameworks
The study identified several critical outcomes regarding AI security incidents. One major finding was the identification of vulnerabilities within AI systems, highlighting areas particularly susceptible to attacks. Additionally, the research proposed new guidelines and frameworks specifically tailored to managing AI incidents.
Insights from simulation and testing provided a deeper understanding of potential threats, underscoring the urgent need to address these vulnerabilities. Such measures are crucial for protecting against malicious attacks and ensuring the safe deployment of AI across various applications.
Implications for Cybersecurity Practices
The implications of this research extend into both practical and theoretical realms within cybersecurity. Existing practices must evolve to address the specific risks associated with AI technologies. The study advocates for adapting current methodologies to better suit these emerging challenges.
A significant recommendation is the establishment of standardized incident reporting frameworks. These frameworks are essential for effectively tracking and managing AI-related incidents, facilitating improved response strategies across industries.
Tools and Frameworks: Enhancing AI Security
Several tools and frameworks were explored in the study, each playing a critical role in enhancing AI security. The Common Vulnerability and Exposure (CVE) Program offers a standardized method for identifying software vulnerabilities. Meanwhile, the MITRE ATT&CK Framework provides a knowledge base of adversary tactics based on real-world observations.
Additionally, Model Cards—tools designed to provide transparency and accountability for AI model performance—were discussed alongside the AI Incident Database (AIID), which serves as a repository for documenting AI-related security incidents.
Future Directions: Bolstering AI Security
Looking ahead, the research outlines several future directions aimed at strengthening AI security. These include developing enhanced security protocols for autonomous systems and creating comprehensive frameworks for reporting and analyzing AI incidents.
Furthermore, there is an emphasis on implementing prevention mechanisms to protect against AI model theft, safeguarding intellectual property from unauthorized access. Addressing security challenges specific to reinforcement learning environments is also highlighted as a priority area.
Strengths and Limitations: A Balanced Perspective
The strengths of this research lie in its thorough methodology and collaborative development process, providing a well-rounded perspective on AI security incidents. However, it acknowledges limitations such as the need for ongoing investigation into specific vulnerabilities as AI technologies continue to advance.
As AI technologies increasingly integrate into various sectors, understanding their security vulnerabilities becomes paramount. This research serves as a foundational step towards establishing safer AI systems, prompting further exploration and collaboration in the field.