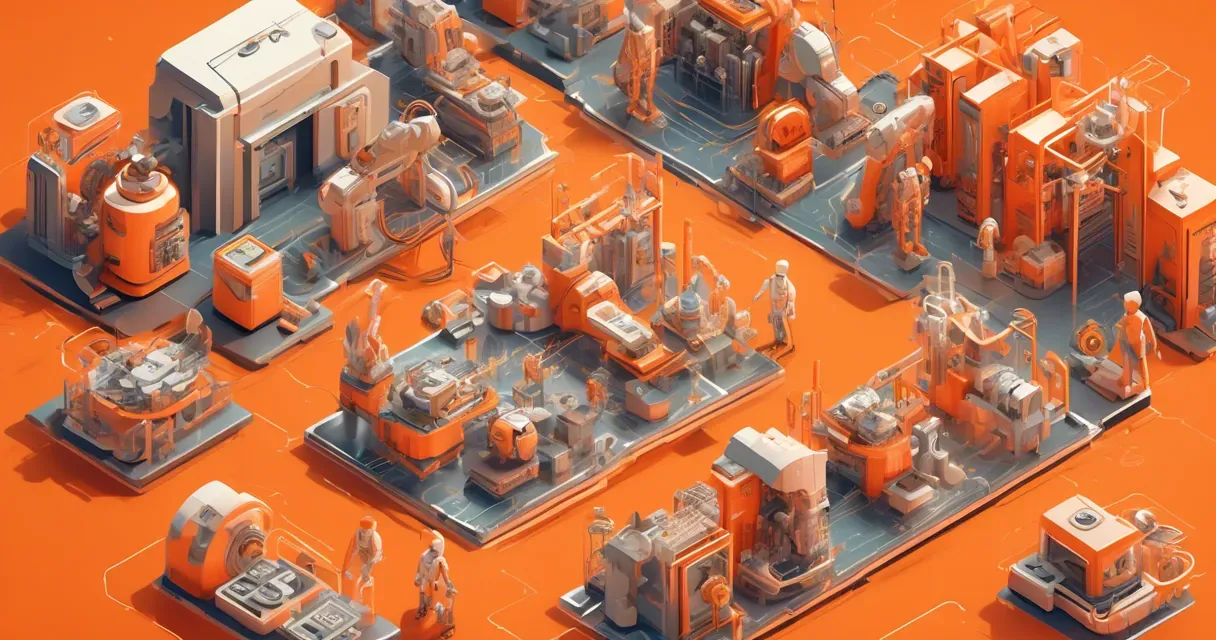
Advancements in Cybersecurity Through Automated Red Teaming
/ 3 min read
Quick take - Recent research on Automated Progressive Red Teaming (APRT) presents new frameworks and methodologies designed to improve cybersecurity practices, particularly for large language models, by integrating adaptive learning and innovative evaluation metrics to address evolving threats.
Fast Facts
- Automated Progressive Red Teaming (APRT): Introduces a framework for enhancing cybersecurity through adaptive learning and evaluation metrics for large language models (LLMs).
- Three-Core Module Framework: Establishes a systematic approach for automated red teaming, focusing on identifying and addressing vulnerabilities.
- Active Learning and Iterative Testing: Employs progressive multi-round interactions to continuously refine security measures and improve model defenses.
- Novel Evaluation Metric: The Attack Effectiveness Rate (AER) quantifies the success of attacks on vulnerabilities, aiding in security strategy assessments.
- Future Directions: Emphasizes the need for real-time threat detection integration, cross-domain assessments, and ethical compliance in cybersecurity practices.
Enhancing Cybersecurity Through Automated Progressive Red Teaming
In a rapidly evolving digital landscape, the need for robust cybersecurity measures has never been more pressing. Recent research into Automated Progressive Red Teaming (APRT) offers promising advancements in this field, focusing on strengthening cybersecurity practices through innovative frameworks and methodologies. This study underscores the importance of integrating adaptive learning and novel evaluation metrics to bolster the safety and reliability of large language models (LLMs) against emerging threats.
Key Findings and Methodologies
The research introduces a comprehensive approach to APRT, highlighting several critical components:
Three-Core Module Framework
At the heart of this research is a three-core module framework designed to systematically engage with potential vulnerabilities. This foundational structure is pivotal in developing automated red teaming strategies that can adapt to an ever-changing threat landscape.
Progressive Multi-Round Interaction
A significant aspect of the study is the progressive multi-round interaction methodology. This approach facilitates iterative testing and adaptation, allowing security measures to be continuously refined. By engaging in multiple rounds of testing, organizations can better prepare for and respond to potential threats.
Active Learning Strategy
Incorporating active learning into the framework fosters a dynamic environment where models can learn from interactions. This strategy enhances the defensive capabilities of LLMs over time, ensuring they remain resilient against adversarial attacks.
Novel Evaluation Metric - Attack Effectiveness Rate (AER)
The introduction of the Attack Effectiveness Rate (AER) provides a quantitative measure of how effectively an attack can exploit vulnerabilities. This metric is crucial for assessing the robustness of security strategies and identifying areas for improvement.
Strengths and Limitations
The APRT research stands out for its comprehensive integration of various methodologies aimed at enhancing LLM safety and reliability. However, it also acknowledges certain limitations. The effectiveness of implemented strategies requires further investigation, particularly concerning their scalability in real-world applications.
Recommended Tools and Frameworks
The study outlines several tools and techniques that can be employed in automated security testing:
- Automated Progressive Red Teaming (APRT): The primary model for conducting automated security assessments.
- Intention Expanding LLM: Enhances contextual understanding, improving responses to adversarial prompts.
- Evil Maker: Generates adversarial scenarios to test LLM robustness.
- UltraFeedback: Incorporates user feedback into LLM training for alignment with safety standards.
- MART (Multi-Round Automatic Red-Teaming): Allows multiple iterations of testing for thorough security assessments.
- Curiosity-Driven Red-Teaming: Encourages exploration through novel testing scenarios.
- AutoDAN (Automatic Jailbreak Prompt Generation): Identifies and exploits weaknesses in LLM defenses.
Future Directions
The research identifies several promising areas for future exploration:
- Integration with Real-Time Threat Detection Systems: Enhancing responsiveness to emerging threats.
- Cross-Domain Vulnerability Assessment: Expanding red teaming applicability across various domains.
- Development of Robust Reward Mechanisms: Creating incentives for models to improve defensive capabilities.
- Ethical and Regulatory Compliance Testing: Ensuring alignment with ethical standards and regulatory requirements.
As cybersecurity challenges continue to evolve, the findings from APRT research highlight the necessity for advanced methodologies in safeguarding digital environments. By leveraging innovative frameworks and continuous learning strategies, researchers and practitioners can collaborate to create a more secure digital future.