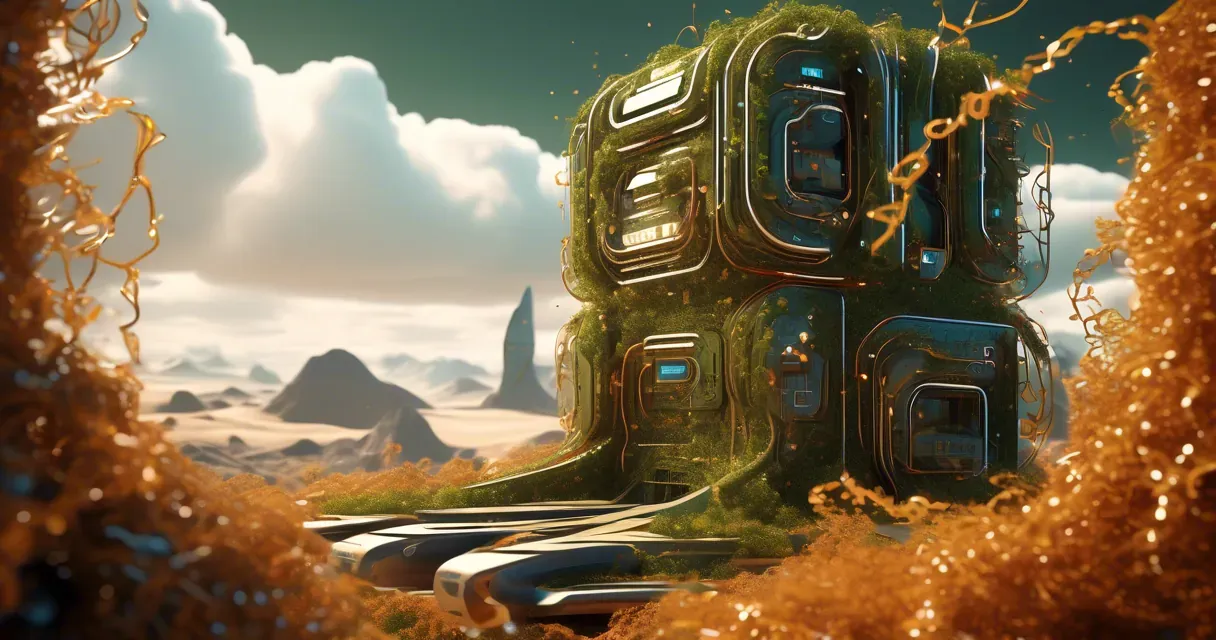
Advancements in Intrusion Detection Systems Enhance Cybersecurity
/ 3 min read
Quick take - Recent research has advanced Intrusion Detection Systems (IDS) by introducing methodologies such as Drift Detection and Strategic Sample Selection, aimed at improving their adaptability and effectiveness against evolving cyber threats.
Fast Facts
- Recent research enhances Intrusion Detection Systems (IDS) through Drift Detection and Strategic Sample Selection to better combat evolving cyber threats.
- Drift Detection identifies when an IDS model requires updates due to changes in data patterns, followed by Strategic Sample Selection for effective data management.
- The Strategic Selection and Forgetting (SSF) method improves model relevance and threat detection performance while maintaining high detection rates and minimizing false positives.
- Recommended tools for implementation include the Kolmogorov-Smirnov Test for drift detection and Kullback-Leibler Divergence for probability distribution analysis.
- The research emphasizes the need for continuous evaluation and adaptation of these methodologies to effectively address the dynamic nature of cyber threats.
Advancements in Intrusion Detection Systems: A New Era of Cybersecurity
In a significant leap forward for cybersecurity, recent research has unveiled innovative methodologies aimed at enhancing Intrusion Detection Systems (IDS). By focusing on Drift Detection and Strategic Sample Selection, these advancements promise to improve the adaptability and effectiveness of IDS amidst the ever-evolving landscape of cyber threats.
Understanding the Core Innovations
The research zeroes in on two pivotal areas: Drift Detection and Strategic Sample Selection. These methodologies are designed to ensure that IDS can dynamically respond to shifts in data patterns that signal potential security threats. This capability is crucial as cyber threats grow more sophisticated and varied.
Drift Detection: A Crucial Component
Drift Detection plays a vital role in identifying when an IDS model requires updating due to changes in the underlying data distribution. This detection is essential for maintaining the relevance and accuracy of threat detection systems. Once a drift is identified, the process moves to the next phase: Strategic Sample Selection.
Strategic Sample Selection: Enhancing Model Adaptability
Strategic Sample Selection involves two key phases:
- Memory Buffer Update: This phase focuses on efficiently managing previously encountered data, ensuring that only the most pertinent samples are retained for analysis.
- Model Fine-Tuning: After updating the memory buffer, the model undergoes fine-tuning with the newly selected samples, thereby enhancing its predictive accuracy and adaptability.
The research introduces the Strategic Selection and Forgetting (SSF) method as a robust approach for managing data within IDS. This method not only helps maintain model relevance but also boosts overall threat detection performance.
Evaluating Strengths and Limitations
The SSF method demonstrates several strengths, including high detection rates and minimized false positives. However, challenges remain, particularly concerning scalability in real-world applications. Further investigation is needed to assess its effectiveness across diverse environments.
Recommended Tools and Techniques
To facilitate the implementation of these methodologies, researchers recommend various tools and techniques:
- Kolmogorov-Smirnov (K-S) Test: A statistical tool for drift detection.
- Kullback-Leibler (KL) Divergence: Measures divergence between probability distributions.
- Memory Buffer Update Mechanism: Ensures data relevance management in IDS.
- Integration with IoT Security Frameworks: Strengthens IoT devices against intrusions.
- Real-Time Threat Intelligence Sharing: Enables rapid dissemination of threat data.
- Adaptive Security Policies in Cloud Environments: Allows dynamic threat responses in cloud systems.
- Enhanced User Behavior Analytics (UBA): Detects anomalies in user behavior patterns.
- Cross-Domain Application in Cybersecurity: Broadens applicability across sectors and domains.
Implications for Cybersecurity
The implications of this research are profound. As organizations adopt these methodologies, they will be better equipped to address the dynamic nature of cyber threats. By leveraging advanced techniques like SSF and integrating them into existing security frameworks, entities can significantly enhance their defensive capabilities. This advancement promises a more secure digital landscape.
However, continuous evaluation and adaptation of these methods will be essential to keep pace with ever-evolving cyber threats. As cybersecurity continues to advance, organizations must remain vigilant and proactive in adopting new technologies and strategies to safeguard their digital assets effectively.
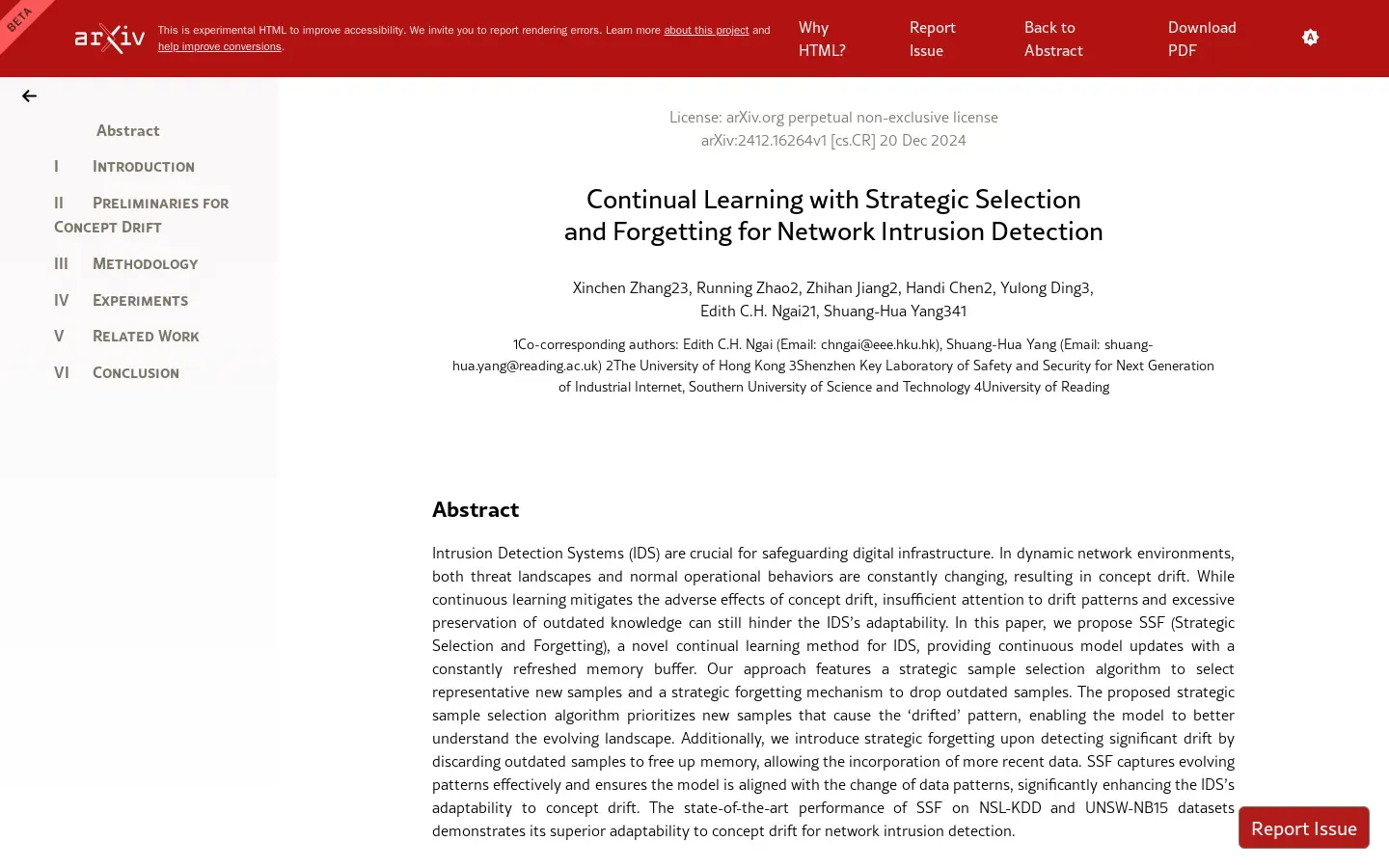