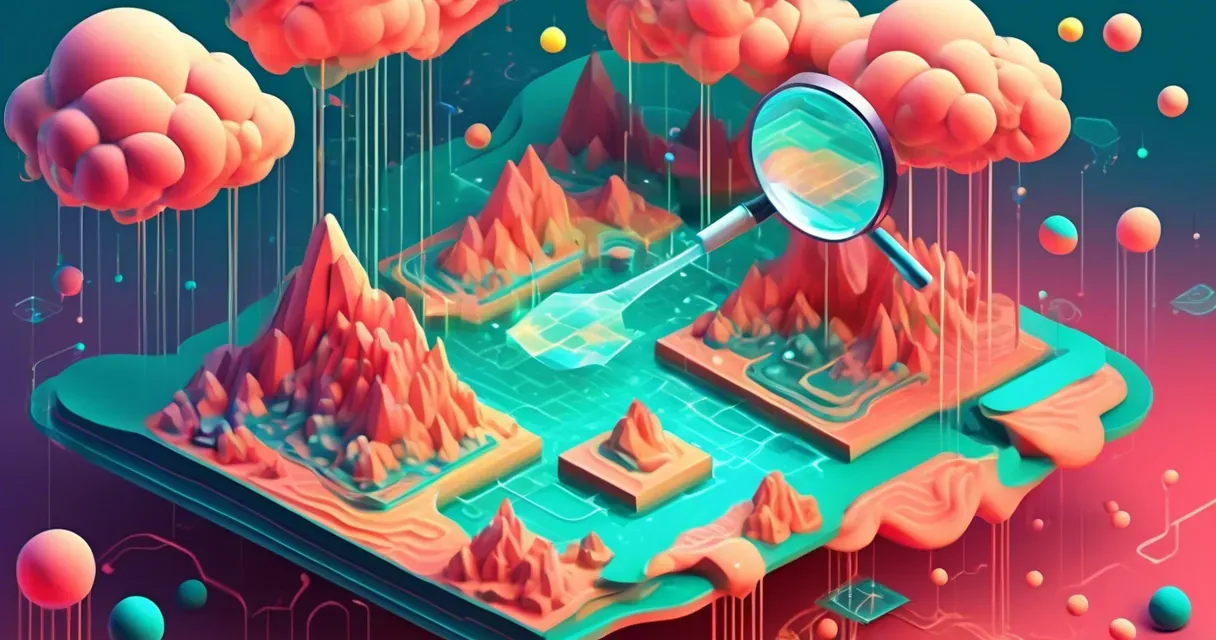
CloudLens Enhances Detection of Cloud Security Vulnerabilities
/ 4 min read
Quick take - Recent research has made notable advancements in cloud security by improving vulnerability detection and threat mitigation strategies through formal modeling, empirical testing, and automated tools, emphasizing the importance of continuous monitoring and proactive policy management to address evolving cyber threats.
Fast Facts
- Recent research has advanced cloud security through improved vulnerability detection and threat mitigation strategies, emphasizing continuous monitoring and proactive policy management.
- A multi-faceted methodology was employed, including formal modeling with CloudLens, innovative planning techniques for vulnerability detection, empirical testing on real-world configurations, and evaluation of attack path diversity.
- Key findings showed significant improvements in vulnerability detection capabilities and highlighted prevalent vulnerabilities in cloud systems, as well as the need for enhanced security measures.
- The research suggests a shift towards automated and comprehensive cloud security, with recommendations for tools like CloudLens and CloudExploit to aid in vulnerability management.
- Future directions include integrating continuous monitoring systems, developing automated remediation strategies, and establishing cross-cloud security frameworks to enhance overall security effectiveness.
Advancements in Cloud Security Research: A New Era of Vulnerability Detection
Recent advancements in cloud security research have unveiled significant progress in vulnerability detection and threat mitigation strategies. This study, which combines formal modeling, empirical testing, and automated tools, underscores the critical need for continuous monitoring and proactive policy management to safeguard against evolving cyber threats.
Methodology and Approach
The research employed a comprehensive approach with four main methodologies. First, Formal Modeling with CloudLens was used to create structured models of cloud configurations, offering a clearer understanding of potential vulnerabilities. This framework allows researchers to simulate various scenarios and identify weaknesses that could be exploited by attackers.
Next, the study introduced Vulnerability Detection through Planning, employing innovative planning techniques to systematically identify vulnerabilities. This method enhances the ability to foresee potential security breaches before they occur.
Empirical Testing on Real-World Configurations was another cornerstone of the research. By analyzing actual cloud environments, the study validated the effectiveness of its proposed methodologies. This real-world application ensures that the findings are not only theoretical but also practical and applicable in current cloud infrastructures.
Lastly, the Evaluation of Attack Path Diversity provided insights into how different configurations could be exploited. By assessing a variety of attack paths, researchers identified areas where existing security measures may fall short.
Key Findings and Results
The research yielded several significant findings. A benchmark evaluation against the IAM Vulnerable Benchmark indicated a marked improvement in vulnerability detection capabilities. This suggests that the new methodologies are more effective than traditional approaches.
Analysis of real-world datasets revealed critical insights into prevalent vulnerabilities within cloud systems. The generation of diverse attack paths highlighted specific areas where current security measures might be insufficient.
Moreover, a comparison with existing tools like PMapper demonstrated the superior capabilities of the proposed solutions. These findings suggest a paradigm shift towards more automated, proactive, and comprehensive cloud security measures.
Implications for Cybersecurity
The implications of these findings are profound for cybersecurity. There is an increasing emphasis on continuous monitoring and integrating advanced detection tools to enhance incident response efforts. As cyber threats become more sophisticated, these proactive measures will be essential in maintaining robust cloud security.
Strengths and Limitations
The strengths of this research lie in its robust methodology and practical applicability. However, limitations were also noted, including the need for further investigation into scalability and real-time applicability across diverse cloud environments. These areas require additional exploration to ensure that the proposed solutions can be effectively implemented on a larger scale.
Recommended Tools and Techniques
Several tools and frameworks were highlighted for their roles in improving cloud security:
- CloudLens: A modeling tool aiding in identifying and mitigating vulnerabilities.
- CloudExploit: An automated tool assisting in vulnerability detection and remediation.
- Planning Domain Definition Language (PDDL): Used for planning in security assessment contexts.
- IAM Vulnerable Benchmark: A benchmarking tool for evaluating identity and access management vulnerabilities.
Future Directions and Applications
The research suggests several promising directions for future work:
- Integration with Continuous Monitoring Systems: Enhancing real-time threat detection capabilities.
- Automated Remediation Strategies: Developing systems that autonomously address vulnerabilities as they are identified.
- Cross-Cloud Security Frameworks: Establishing frameworks providing security assessments across multiple cloud environments.
- User Behavior Analytics (UBA) Integration: Incorporating UBA to improve anomaly detection and response strategies.
This research marks a significant step forward in cloud security, emphasizing innovative approaches to vulnerability detection and management. As cyber threats continue to evolve, integrating advanced tools and methodologies will be crucial in maintaining the integrity and security of cloud systems.