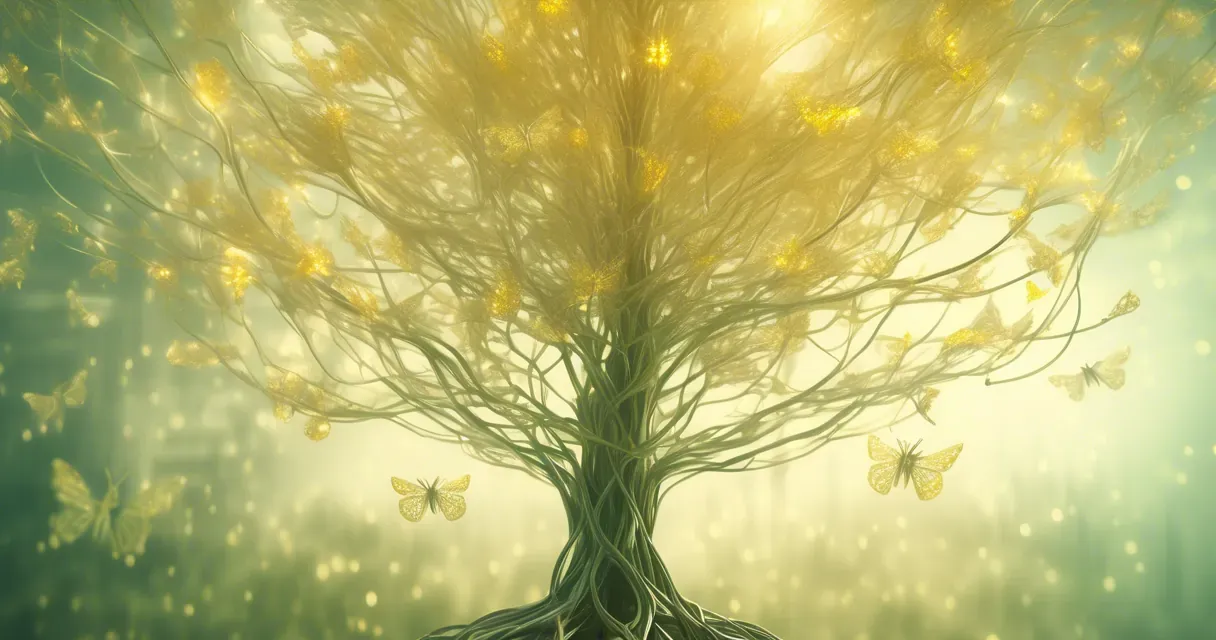
Temporal Convolutional Networks Enhance Network Intrusion Detection
/ 4 min read
Quick take - Recent research has introduced a Temporal Convolutional Network (TCN) model for network intrusion detection, demonstrating improved performance over traditional methods while highlighting the need for further investigation into its limitations and potential applications across various domains.
Fast Facts
- Novel Approach: Research introduces a Temporal Convolutional Network (TCN) model for enhanced network intrusion detection, aiming to improve cybersecurity measures.
- Methodology: The study involves model development, dataset preparation using the Edge-IIoTset, and rigorous training and evaluation to assess effectiveness.
- Key Findings: The TCN model outperforms traditional methods in detecting network intrusions, though it requires larger datasets and further testing under varied conditions.
- Practical Implications: The model can be integrated into real-time intrusion detection systems, utilize adaptive learning, and collaborate with threat intelligence platforms for improved security.
- Future Directions: Suggested avenues include expanding real-time applications, developing adaptive learning mechanisms, and exploring cross-domain applications of the TCN model.
Advancements in Network Intrusion Detection Through Temporal Convolutional Networks
In a significant stride towards bolstering cybersecurity, recent research has introduced a novel approach to network intrusion detection utilizing Temporal Convolutional Networks (TCNs). This innovative model promises to enhance the detection and response capabilities of cybersecurity systems, particularly in environments involving Internet of Things (IoT) devices and edge computing. The study meticulously details the development, evaluation, and potential applications of this advanced model, marking a pivotal moment for cybersecurity practices.
Methodology: A Structured Approach
The research employs a comprehensive methodology divided into three critical phases.
Model Development
The TCN model was crafted with specific architectural considerations aimed at optimizing its performance in identifying network intrusions. By leveraging the unique capabilities of TCNs, the model is designed to process sequential data effectively, which is crucial for timely threat detection.
Dataset Preparation
For training and testing purposes, the researchers utilized the Edge-IIoTset dataset. This choice ensures that the model is well-equipped to handle real-world scenarios typical of IoT and edge computing environments. The dataset’s relevance to these domains is instrumental in preparing the model for practical applications.
Model Training and Evaluation
Rigorous training and evaluation processes were conducted to assess the TCN model’s effectiveness. The focus was on determining its accuracy and reliability in identifying network threats, with results indicating superior performance compared to traditional methods.
Key Findings: Strengths and Limitations
The study highlights several strengths of the TCN model. It demonstrates exceptional capability in detecting a wide range of network intrusions, outperforming conventional techniques. The model’s proficiency in processing sequential data allows for rapid identification and response to potential threats.
However, the research also acknowledges certain limitations. There is a need for more extensive datasets to further validate the model’s efficacy across diverse conditions. Additionally, its performance under varied network environments remains an area for further investigation.
Practical and Theoretical Implications
The implications of these findings are profound for cybersecurity:
- Real-Time Intrusion Detection Systems (IDS): The TCN model can be integrated into real-time IDS, enhancing security measures for IoT devices and edge computing.
- Adaptive Learning Mechanisms: The study suggests implementing adaptive learning to enable the model to evolve alongside emerging threats.
- Integration with Threat Intelligence Platforms: Collaborating with threat intelligence platforms could fortify the model’s ability to detect sophisticated cyber threats.
- Cross-Domain Applications: Beyond cybersecurity, the TCN model’s architecture may find applications in other fields requiring sequence-based data analysis.
Recommended Tools and Techniques
Several tools and frameworks are pivotal to the success of the TCN model:
- Temporal Convolutional Networks (TCNs): Essential for processing sequential data, making them ideal for intrusion detection tasks.
- Residual Block Architecture: Enhances learning complex patterns through skip connections.
- Dilated Convolutions: Captures a larger context of input data without significantly increasing computational costs.
- Edge-IIoTset Dataset: A critical resource reflecting real-world scenarios in network intrusion detection.
Future Directions
The research outlines promising avenues for future exploration:
- Real-Time IDS Deployment: Expanding deployment in real-time systems could strengthen security frameworks across industries.
- Adaptive Learning Development: Focus on mechanisms allowing quick adaptation to new intrusion types.
- Threat Intelligence Integration: Collaborating with existing systems may enhance prediction and mitigation capabilities.
- Cross-Domain Exploration: Investigating applicability in other domains could broaden the impact of this technology.
As cybersecurity continues to evolve, these advancements underscore the importance of innovative approaches like TCNs in safeguarding digital infrastructures against ever-evolving threats.