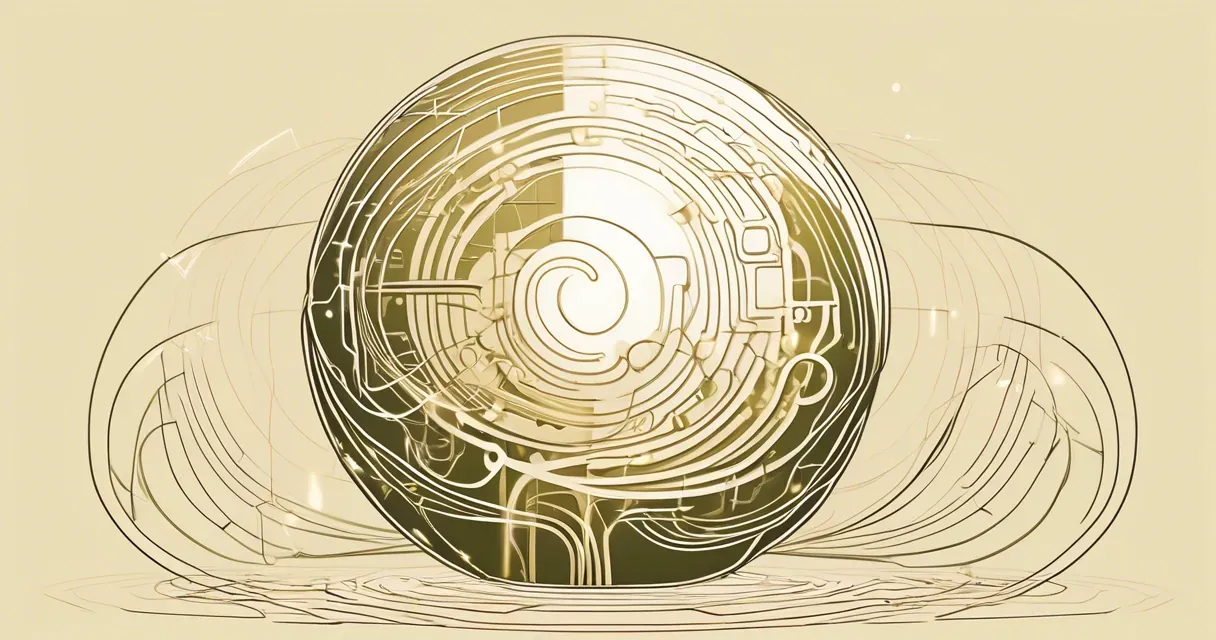
AI Integration with End-to-End Encryption: Challenges and Recommendations
/ 3 min read
Quick take - A recent research paper examines the compatibility of artificial intelligence with end-to-end encryption systems, highlighting the complexities and implications for cybersecurity, while offering recommendations for technical design, user consent, and ethical considerations.
Fast Facts
- A research paper titled “How To Think About End-To-End Encryption and AI” examines the compatibility of AI with end-to-end encryption (E2EE), highlighting cybersecurity implications.
- The study identifies four key objectives: assessing AI and E2EE compatibility, analyzing legal and ethical issues, recommending technical designs, and presenting relevant case studies.
- It emphasizes the need for careful integration of AI to enhance E2EE without compromising user privacy and data protection.
- Recommendations include implementing trusted execution environments, local data processing, opt-in consent management, and privacy-preserving technologies.
- The paper advocates for developing privacy-preserving AI models and comprehensive policies to ensure responsible AI deployment within E2EE frameworks.
Navigating the Intersection of Artificial Intelligence and End-to-End Encryption
Artificial intelligence (AI) is rapidly transforming various sectors, prompting a critical examination of its compatibility with end-to-end encryption (E2EE) systems. A recent research paper titled “How To Think About End-To-End Encryption and AI” delves into this intersection, revealing significant implications for cybersecurity. As AI continues to evolve, understanding how it can be integrated with E2EE without compromising security principles is essential.
Compatibility Challenges Between AI and E2EE
The research paper identifies several challenges in integrating AI with E2EE systems. While AI has the potential to enhance E2EE by improving efficiency and user experience, it also introduces vulnerabilities that could be exploited by malicious actors. The study emphasizes the need for a careful approach to ensure that AI does not undermine the core principles of E2EE, such as data confidentiality and integrity.
Legal and Ethical Considerations
Deploying AI within E2EE frameworks raises complex legal and ethical questions. The research scrutinizes these implications, focusing on user privacy and data protection. Ethical concerns are paramount, as the use of AI technologies can lead to potential misuse or unauthorized data access. Ensuring user consent and transparency in data processing is crucial to maintaining trust in these systems.
Recommendations for Technical Design and User Consent
To address these challenges, the paper advocates for improved technical designs that incorporate robust user consent frameworks. By ensuring that users are informed and have control over their data processing, trust in E2EE systems can be bolstered. This approach allows for the beneficial integration of AI while safeguarding user privacy.
Case Study Insights
The research includes case studies that provide valuable insights into the real-world application of AI in E2EE settings. These examples highlight both the potential pitfalls and successes of such integrations, offering lessons for future implementations. Understanding these case studies is essential for stakeholders aiming to navigate the complexities of AI and E2EE.
Implications for Cybersecurity
The integration of AI into E2EE systems poses significant challenges that require robust solutions to protect user privacy and data integrity. The research outlines several tools and frameworks that can aid in this endeavor:
-
Trusted Execution Environments (TEEs): Secure areas within processors that protect sensitive data during processing.
-
Local Processing: Keeping data processing local rather than cloud-based reduces transmission risks.
-
Opt-in Consent Management: Frameworks requiring explicit user consent before data use foster trust.
-
Privacy-Preserving Technologies: Innovations allowing data analysis without compromising privacy.
Moreover, the paper recommends developing privacy-preserving AI models, enhancing user consent frameworks, implementing AI-driven threat detection mechanisms within E2EE systems, and formulating comprehensive policies to guide the integration process.
In summary, integrating AI into end-to-end encryption systems presents both opportunities and challenges. By following the recommendations from this research, stakeholders can work towards a cybersecurity landscape that respects privacy while embracing technological advancements.
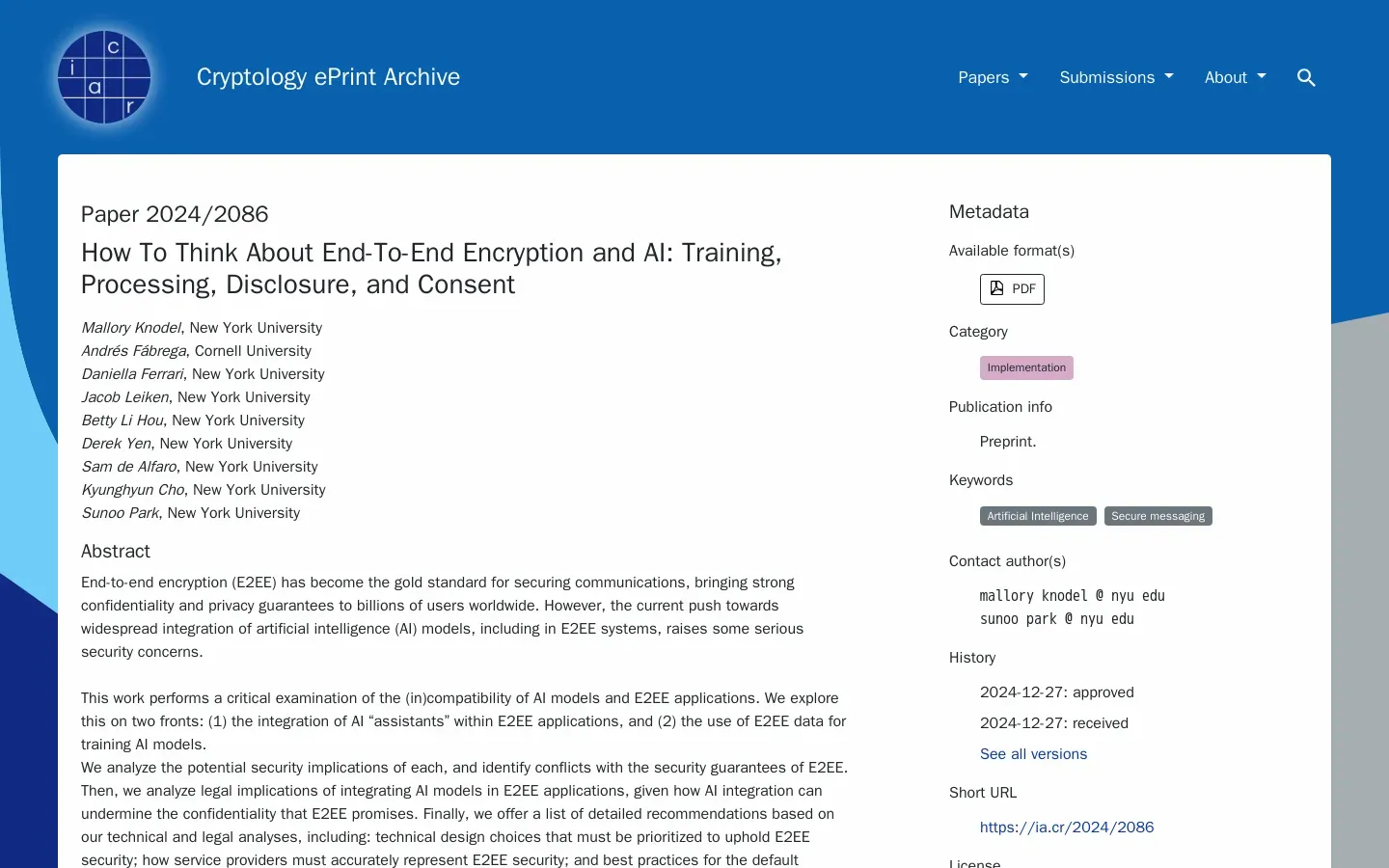