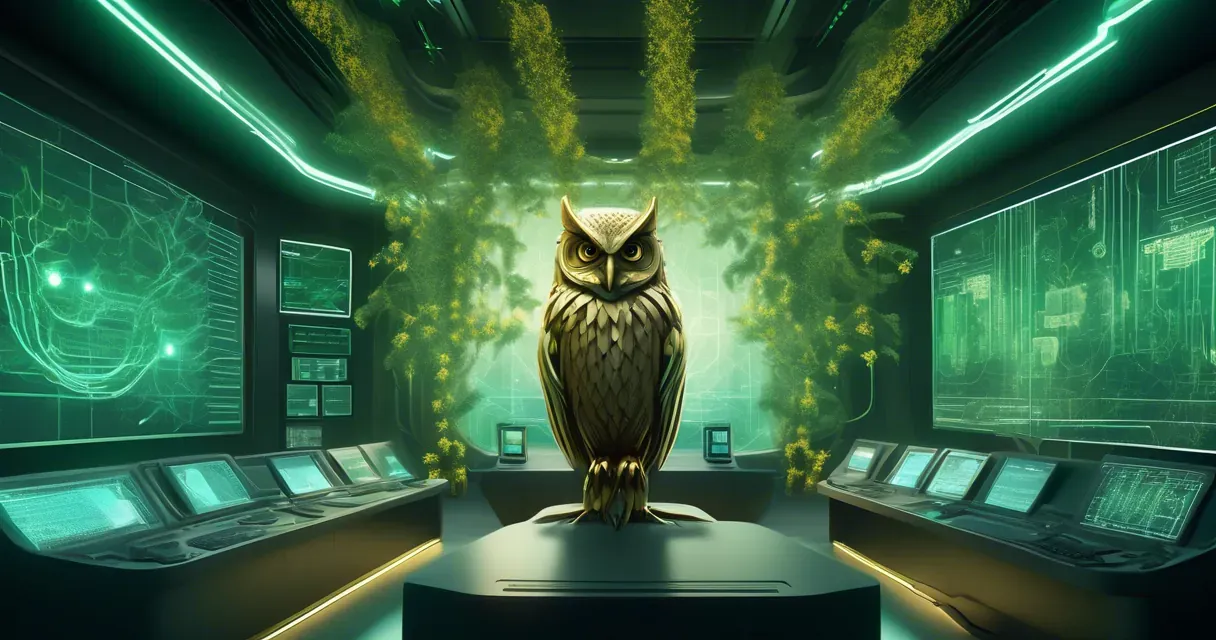
Large Language Models Enhance Cybersecurity Incident Response
/ 4 min read
Quick take - Recent research highlights the potential of large language models to significantly improve collaboration, efficiency, and effectiveness in incident response scenarios within cybersecurity.
Fast Facts
- Recent research highlights the use of large language models (LLMs) to improve multi-agent collaboration in cybersecurity incident response (IR) scenarios.
- LLMs enhance decision-making and adaptability, providing real-time insights that improve team performance across various structural configurations.
- The study emphasizes the importance of training simulations incorporating LLMs to boost preparedness and responsiveness in incident response.
- Practical applications include the need for robust network monitoring and adaptive response strategies to effectively detect and respond to threats.
- Future directions suggest developing advanced user behavior analytics, integrated monitoring systems, and decentralized threat intelligence sharing to strengthen cybersecurity resilience.
Advancements in Incident Response Through Large Language Models
Recent research has unveiled promising advancements in the use of large language models (LLMs) to enhance multi-agent collaboration in incident response (IR) scenarios within cybersecurity. The integration of LLMs into existing frameworks could significantly improve collaboration, efficiency, and overall effectiveness in addressing cybersecurity challenges.
Key Objectives and Methodology
The study aimed to explore how LLMs can optimize incident response processes through improved communication, decision-making, and adaptability among cybersecurity teams. Researchers established a controlled environment to simulate various incident response scenarios. This setup allowed for the evaluation of team interactions and decision-making processes under different conditions.
Study Design and Simulation Setup
To understand the impact of LLMs, researchers simulated diverse incident response scenarios. These simulations provided a platform to assess how teams interact and make decisions when augmented with LLMs.
Team Structure Variations
The study analyzed centralized, decentralized, and hybrid team structures to determine how these configurations affect response effectiveness when supported by LLMs. This analysis was crucial in understanding the flexibility and adaptability of different team setups.
Execution of Simulations
A series of simulations were conducted where teams used LLMs to address various cybersecurity incidents. The focus was on assessing how these models enhanced real-time communication and collaboration among team members.
Performance Evaluation and Analysis
Teams’ performance was rigorously evaluated based on their speed and accuracy in responding to incidents. Special attention was given to the role of LLMs in facilitating these processes, highlighting their potential to transform incident response strategies.
Key Findings
Several critical findings emerged from the research regarding the integration of LLMs in incident response:
-
Enhanced Decision-Making and Adaptability: LLMs provided teams with real-time insights and recommendations, improving their ability to adapt to dynamic threats.
-
Optimized Team Structures: Teams leveraging LLMs showed improved performance across different structural configurations, indicating that these models can effectively support both centralized and decentralized approaches.
-
Training and Simulation: The study underscored the importance of incorporating LLMs into training exercises, suggesting that simulations can enhance preparedness and responsiveness.
-
Scalability and Resource Allocation: LLMs can assist in scaling response efforts and optimizing resource allocation, ensuring that cybersecurity teams can respond more effectively to high-volume incidents.
Practical and Theoretical Implications
The implications of this research extend beyond theoretical frameworks, presenting practical applications for cybersecurity teams:
-
Comprehensive Network-Level Anomaly Detection: Robust network monitoring is essential for identifying anomalies that could indicate malicious activity. Implementing network intrusion detection systems (NIDS) with machine learning capabilities is recommended.
-
Adaptive Response Strategies: Enhancing incident response frameworks to be more dynamic and user-focused is crucial for effective threat detection and response.
Strengths and Limitations of the Research
The research showcases significant strengths, including innovative methodologies and practical applications of LLMs. However, it also has limitations. Areas requiring further investigation include the long-term effects of LLM integration in real-world scenarios and the potential for biases in model outputs.
Tools and Techniques Discussed
Several tools and frameworks were explored in the study, essential for improving cybersecurity defense:
-
Large Language Models (LLMs): These models serve as the backbone for enhancing communication and decision-making in incident response.
-
Backdoors & Breaches (B&B): A framework used to analyze vulnerabilities and attack vectors.
-
AutoGen Framework: This tool aids in automating the generation of incident response playbooks.
-
User Behavior Analytics (UBA): Critical for understanding normal user behavior and identifying deviations indicative of potential security threats.
Future Directions
Based on the research findings, several areas for expansion are proposed to enhance real-world cybersecurity scenarios:
-
Enhanced User Behavior Analytics (UBA) Systems: Developing more sophisticated systems for monitoring user behavior to detect anomalies.
-
Integrated Network and Endpoint Monitoring: Combining network and endpoint monitoring for a holistic view of security threats.
-
Adaptive Incident Response Frameworks: Creating frameworks that adapt to evolving threats while incorporating LLMs for real-time decision support.
-
Decentralized Threat Intelligence Sharing: Encouraging collaboration across organizations to share threat intelligence, thereby improving overall cybersecurity resilience.
The integration of large language models into incident response frameworks represents a transformative step forward for cybersecurity teams, enabling enhanced collaboration and response capabilities in an increasingly complex threat landscape.