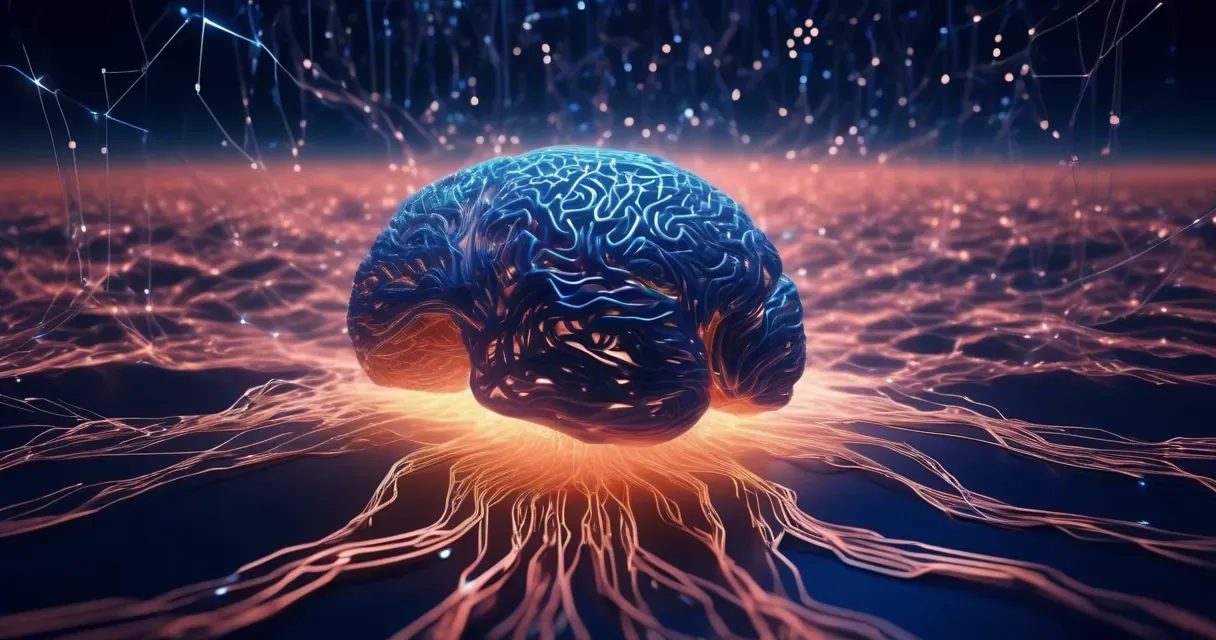
Large Language Models in Software Supply Chain Security
/ 3 min read
Quick take - Recent research highlights the potential of Large Language Models to improve software supply chain security through enhanced vulnerability detection and automated processes, while also acknowledging the challenges and limitations that must be addressed for effective implementation.
Fast Facts
-
Transformative Potential: Research highlights Large Language Models (LLMs) as powerful tools for enhancing software supply chain security, offering both opportunities and challenges.
-
Methodological Framework: A structured experimental framework was developed to evaluate LLMs, utilizing benchmarks like TruthfulQA and a balanced dataset for robust training.
-
Key Applications: LLMs can enable real-time vulnerability detection, automate security audits, enhance threat intelligence, and facilitate crowdsourced vulnerability reporting.
-
Research Strengths: The study showcases LLMs’ capabilities in improving vulnerability detection and predictive analytics, positioning them as critical tools in cybersecurity.
-
Challenges Ahead: Limitations include the risk of biased outputs, the need for comprehensive training datasets, and integration into existing security frameworks, necessitating further research.
Advancements in Software Supply Chain Security Through Large Language Models
In a rapidly evolving digital landscape, the security of software supply chains is paramount. Recent research has illuminated the potential of Large Language Models (LLMs) to transform this critical area of cybersecurity. While these models offer promising advancements, they also introduce challenges that must be carefully managed for successful implementation.
Transformative Potential of LLMs
The study delves into the capabilities of LLMs in bolstering software supply chain security, showcasing methodologies that could redefine industry practices. At the core of this research is an experimental framework designed to scrutinize how LLMs can address vulnerabilities effectively. This structured approach provides a comprehensive analysis of LLM performance across various security contexts.
Methodology and Framework
A pivotal aspect of the research was the utilization of the TruthfulQA benchmark, which played a crucial role in evaluating the reliability and accuracy of LLM outputs. This benchmark ensures that models deliver truthful and contextually relevant information, a necessity in cybersecurity applications.
Dataset construction was another critical component, with researchers curating a balanced representation of vulnerabilities. This meticulous dataset enabled robust training and evaluation, ensuring that LLMs are well-equipped to handle diverse security threats. The iterative testing and performance evaluation further refined these models, focusing on their real-time detection and response capabilities.
Key Findings
The findings reveal several practical applications for LLMs in enhancing software supply chain security:
-
Real-Time Vulnerability Detection: LLMs can continuously monitor systems, identifying vulnerabilities as they emerge and enabling immediate remediation.
-
Automated Security Audits: By automating routine evaluations, LLMs streamline compliance processes and minimize human error.
-
Predictive Analytics: These models can analyze extensive datasets to forecast potential threats, allowing for proactive rather than reactive measures.
-
Crowdsourced Reporting: LLMs facilitate collaborative vulnerability identification by harnessing community input for knowledge sharing.
Strengths and Challenges
The innovative application of LLMs in cybersecurity highlights their potential to enhance vulnerability detection, automate audits, and leverage predictive analytics. However, this research also identifies significant challenges that require further investigation.
Limitations
One major concern is the potential for LLMs to produce misleading or biased outputs. Ensuring comprehensive training datasets is essential to mitigate this risk. Additionally, integrating these advanced models into existing security frameworks poses its own set of challenges.
Implications for Cybersecurity
The implications for cybersecurity are profound. The integration of LLMs into software supply chain strategies could lead to more sophisticated and responsive systems. However, addressing the outlined challenges is crucial to ensure safe and effective deployment.
As the cybersecurity field continues to evolve, ongoing research and development will be essential in navigating the complexities associated with LLMs. Stakeholders must remain vigilant in addressing potential pitfalls while leveraging these technologies to enhance software supply chain security.
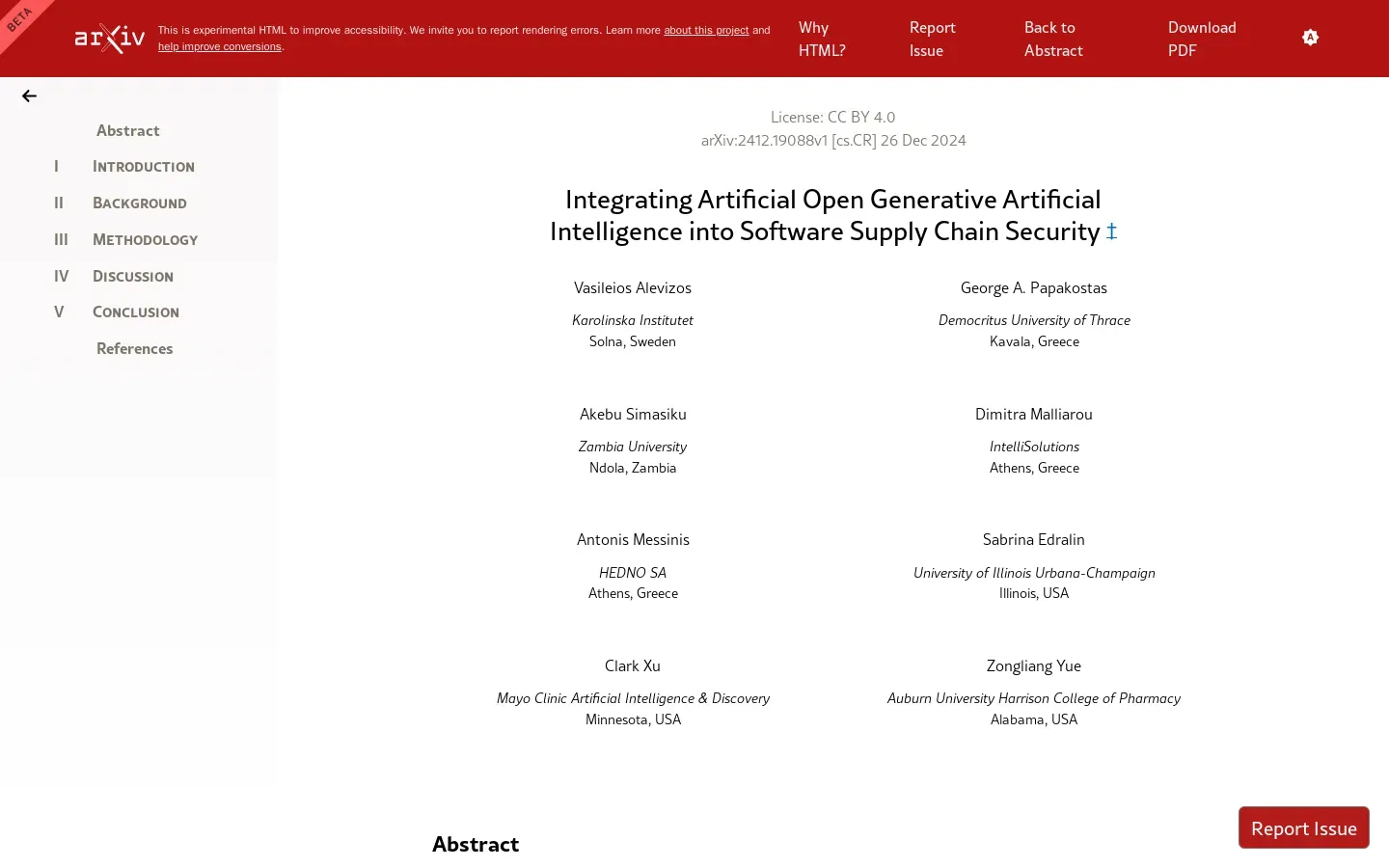