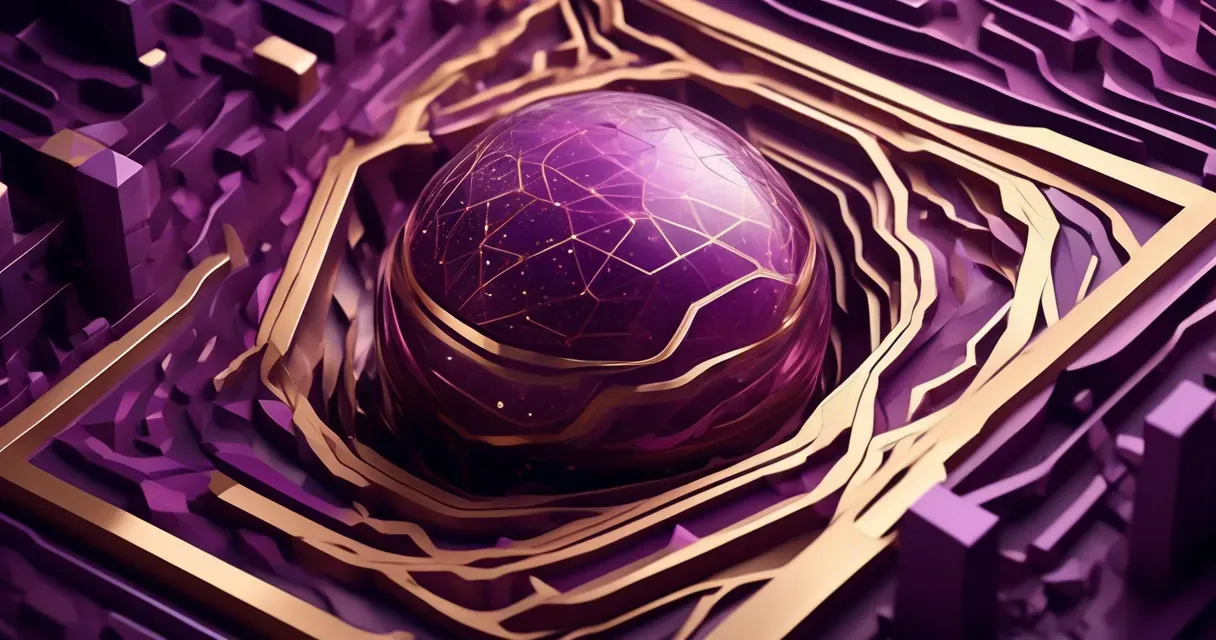
Packet Vision Method Enhances Network Traffic Classification
/ 3 min read
Quick take - Researchers have introduced the Packet Vision method, a novel approach using convolutional neural networks for enhanced network traffic classification, which improves security monitoring, preserves user privacy, and demonstrates scalability for diverse network environments.
Fast Facts
-
Introduction of Packet Vision Method: A new approach using convolutional neural networks (CNNs) for enhanced network traffic classification, particularly relevant for 5G architectures.
-
Multi-Step Process: The method involves data collection, image generation from network packets, CNN training, and statistical analysis to improve traffic analysis and classification.
-
Key Benefits: Enhances security monitoring, preserves user privacy, and demonstrates scalability for efficient network management.
-
Foundation for Future Research: The findings support further innovations in network traffic classification methodologies and technologies.
-
Practical Applications: Includes real-time traffic monitoring, integration with intrusion detection systems, IoT security, and cross-domain traffic classification.
Advancements in Network Traffic Classification with the Packet Vision Method
In a notable stride for network security and management, researchers have introduced the Packet Vision method, a pioneering approach employing convolutional neural networks (CNNs) for network traffic classification. This technique is particularly pertinent amid the rise of advanced network architectures, such as 5G, aiming to refine the efficiency and accuracy of network traffic analysis.
Overview of the Packet Vision Method
The Packet Vision method follows a multi-step process that begins with data collection and preprocessing. It then progresses to image generation, CNN training and evaluation, and concludes with statistical analysis. This systematic approach converts network packets into visual formats used to train CNNs. The end result is a comprehensive set of images that encapsulate the complexities of network traffic, facilitating advanced analysis and classification.
Key Findings and Implications
Enhanced Traffic Classification for Security Monitoring
The research highlights significant improvements in classifying network traffic, which strengthens security monitoring efforts. This enhancement is vital for identifying malicious activities within network environments.
Privacy Preservation in Traffic Analysis
A standout feature of the Packet Vision method is its ability to maintain user privacy while conducting thorough traffic analysis. This aspect is increasingly important given today’s heightened concerns over data privacy.
Scalability and Efficiency in Network Management
The method demonstrates scalability, making it suitable for various network environments, including those handling vast amounts of data. This scalability enhances overall network management efficiency.
Foundation for Future Research and Development
The findings lay a foundation for future research initiatives, potentially leading to further innovations in network traffic classification methodologies and technologies.
Strengths and Limitations
While promising, the Packet Vision method has both strengths and limitations. Its robust classification capabilities and privacy-preserving techniques are notable strengths. However, challenges may arise due to computational demands and the need for extensive training data to achieve optimal performance.
Tools and Techniques Utilized
Several essential tools and techniques underpin the effectiveness of the Packet Vision method:
- Packet Vision: The core framework transforming network packets into images for CNN training.
- Convolutional Neural Networks (CNNs): The backbone of the classification process, enabling advanced pattern recognition.
- Data Augmentation: Techniques that expand the training dataset, enhancing model generalization.
- Fine-Tuning: Adjustments made to pre-trained models to improve performance on specific tasks or datasets.
Practical Applications
The Packet Vision method opens doors to several practical applications:
- Real-Time Traffic Monitoring and Anomaly Detection: Facilitating immediate identification of irregular patterns indicative of security threats.
- Integration with Intrusion Detection Systems (IDS): Enhancing IDS capabilities by improving classification and detection of malicious traffic.
- Application in IoT Security: Addressing challenges posed by Internet of Things (IoT) devices to ensure their safety within networks.
- Privacy-Preserving Traffic Analysis: Ensuring compliance with privacy regulations while maintaining effective traffic analysis.
- Cross-Domain Traffic Classification: Allowing classification across different types of networks, enhancing versatility.
As organizations transition to more complex architectures like 5G, understanding these advancements becomes crucial. The Packet Vision method’s implications for future research and practical applications could redefine how organizations monitor and manage their network environments.