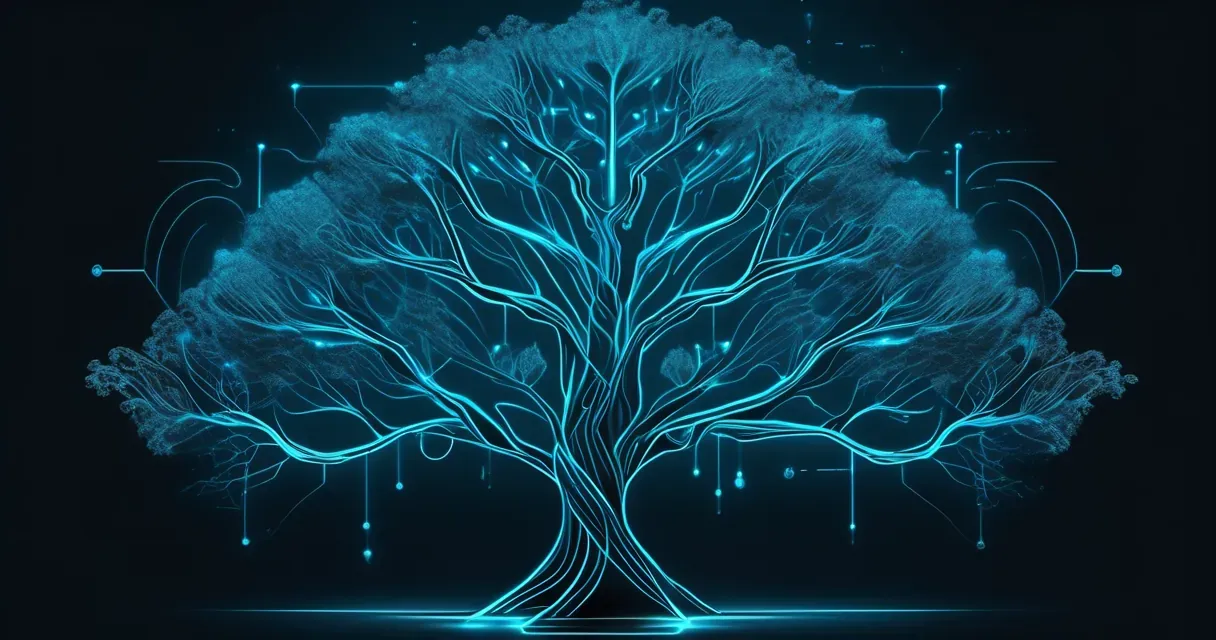
Blockchain and Federated Learning Enhance Cybersecurity Solutions
/ 4 min read
Quick take - Recent research highlights advancements in integrating federated learning and blockchain technology to improve cybersecurity in distributed systems by addressing data privacy, security threats, and resource management challenges.
Fast Facts
- Recent research integrates federated learning (FL) and blockchain technology to enhance cybersecurity in distributed systems, addressing data privacy and security threats.
- Key methodologies include secure registration, resource-aware mechanisms, outlier detection, and decentralized consensus for model validation.
- Findings highlight improved trustworthiness, mitigation of data poisoning attacks, and enhanced accountability through blockchain’s traceability.
- The study identifies limitations such as scalability and computational overhead, suggesting further investigation into these areas.
- Future directions include exploring privacy-preserving techniques, analyzing security threats, and developing efficient consensus mechanisms for federated learning.
Advancements in Federated Learning and Blockchain: A New Frontier in Cybersecurity
Recent research has unveiled significant advancements in the integration of federated learning (FL) and blockchain technology, marking a pivotal step forward in cybersecurity for distributed systems. This innovative approach addresses pressing challenges such as data privacy, security threats, and resource management. The study provides a robust framework that enhances trustworthiness and mitigates various forms of cyberattacks, offering a promising solution to the complexities of modern digital environments.
Key Objectives and Methodology
The primary aim of this research was to develop a secure, efficient, and trustworthy system for federated learning. Several methodologies were employed to achieve this:
-
Registration and Token Generation: A secure registration process was established for participants, enabling them to generate tokens for system access. This ensures that only authorized users can participate in the network.
-
Activity and Resource-Aware Mechanism: Mechanisms were implemented to monitor and optimize resource use, ensuring efficient operation within the federated learning framework. This is particularly crucial for environments with limited resources.
-
Outlier Detection and Reputation-Based Trust Model: The study developed a model that detects outlier behavior among participants and assesses their trustworthiness based on historical activity. This helps maintain data integrity by identifying potentially malicious actions.
-
Consensus Mechanism and Model Update Validation: Decentralized consensus mechanisms were introduced to validate model updates, ensuring data integrity and trust among participants. This enhances the reliability of the federated learning environment.
Key Findings
The research yielded several significant findings that reinforce the effectiveness of the proposed framework:
-
Enhanced Trustworthiness in Distributed Systems: The integration of blockchain technology boosts the reliability of federated learning environments by providing a transparent and immutable record of all transactions.
-
Mitigation of Poisoning Attacks: Strategies were developed to counteract data poisoning attacks, which are common threats in federated learning systems. These strategies enhance the resilience of the network against malicious actors.
-
Blockchain for Authenticity and Accountability: Blockchain ensures that all actions within the network are traceable and verifiable, enhancing accountability among participants.
-
Resource-Aware Security Mechanisms: The proposed mechanisms optimize resource allocation, which is particularly crucial for resource-constrained environments like Internet of Things (IoT) devices.
Strengths of the Research
This research showcases innovative approaches to combining federated learning and blockchain, demonstrating significant potential for enhancing cybersecurity practices. The methodologies employed are robust, addressing both theoretical and practical challenges in the field.
Limitations and Areas for Further Investigation
Despite its strengths, the research acknowledges certain limitations:
-
Scalability: There is a need for further investigation into the scalability of the proposed systems in large-scale applications.
-
Computational Overhead: The long-term implications of integrating blockchain with federated learning concerning computational overhead require further exploration.
-
Effectiveness of Outlier Detection Techniques: The effectiveness of these techniques in varied real-world scenarios remains an area for future study.
Tools and Frameworks
Several crucial tools and frameworks were integral to this research:
-
Federated Learning (FL): A distributed machine learning technique that allows devices to collaboratively learn while keeping data localized.
-
Blockchain Technology: A decentralized ledger that enhances security, accountability, and transparency in federated learning.
-
Reputation-Based Trust Model: A framework for assessing participant trustworthiness based on past behavior and contributions.
-
Outlier Detection Techniques: Methods designed to identify abnormal behavior among participants, ensuring data integrity.
Future Directions and Applications
The research outlines several promising future directions:
-
Investigating Privacy-Preserving Techniques: Exploring advanced methods for ensuring user confidentiality within federated learning systems.
-
Analyzing Security Threats: Conducting comprehensive studies on security threats such as data poisoning and developing countermeasures.
-
Evaluating Resource Management: Assessing blockchain-enabled frameworks’ effectiveness in optimizing resource management in federated learning, particularly in IoT devices.
-
Developing Consensus Mechanisms: Proposing decentralized consensus mechanisms that enhance efficiency and security in federated learning processes.
In summary, integrating federated learning with blockchain technology presents transformative opportunities for cybersecurity. As digital landscapes continue to evolve, ongoing research in this area is essential for addressing emerging challenges and enhancing distributed systems’ resilience against cyber threats.