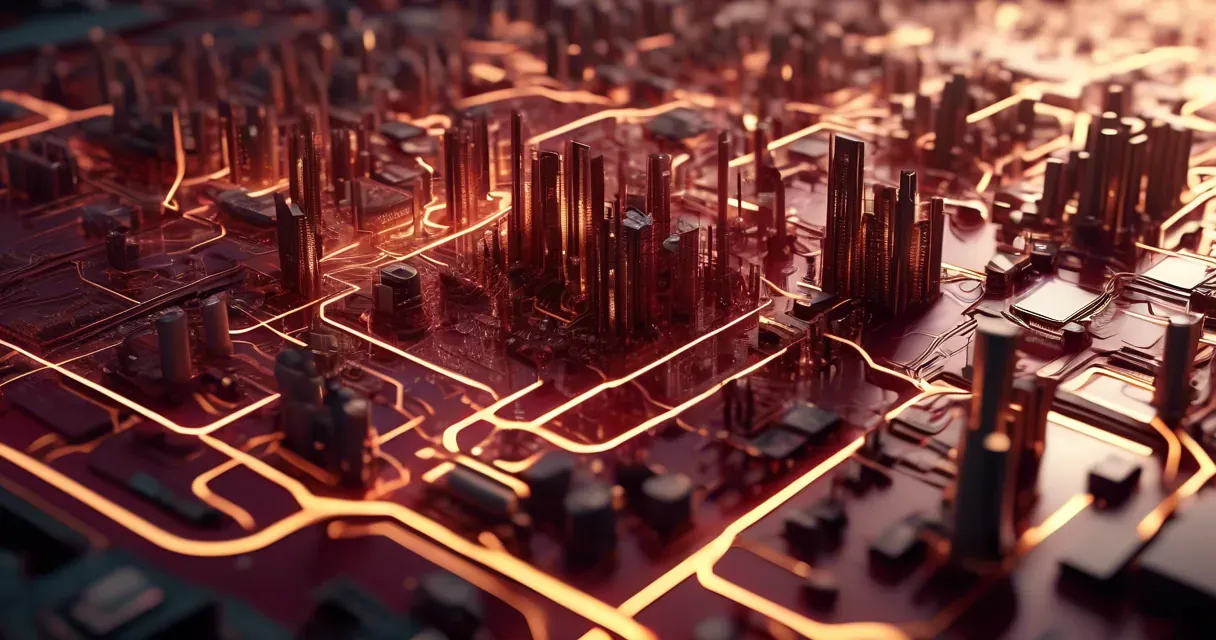
Deep Learning Enhances Security for LoRa Device Identification
/ 4 min read
Quick take - Recent research by Yalin E. Sagduyu and Tugba Erpek has developed deep learning models to enhance security in Low Power Wide Area Networks (LoRa) by effectively classifying legitimate and rogue devices, while also proposing robust defense mechanisms against potential vulnerabilities.
Fast Facts
- Research Focus: The study by Sagduyu and Erpek aims to enhance security in LoRa networks by developing deep learning models to classify legitimate and rogue devices, addressing vulnerabilities in IoT applications.
- Methodology: It involves data collection, signal processing, and the use of deep learning techniques like DNNs and CNNs, alongside adversarial attack simulations to test classifier robustness.
- Key Findings: The research emphasizes the importance of tailored security protocols for IoT devices and demonstrates that multi-task learning can improve classification efficiency and resource allocation.
- Tools Utilized: Techniques such as Fast Gradient Sign Method (FGSM), adversarial training, and Kernel Density Estimation (KDE) are employed to enhance classifier resilience and accuracy.
- Implications: The findings could influence practical cybersecurity strategies for IoT networks, promoting robust security measures and cross-platform solutions for smart city infrastructures.
Enhancing Security in LoRa Networks through Deep Learning Techniques
Recent research by Yalin E. Sagduyu and Tugba Erpek has made noteworthy progress in addressing security vulnerabilities within Low Power Wide Area Networks (LoRa), which are increasingly integral to Internet of Things (IoT) applications. The study introduces deep learning models designed to identify and classify LoRa devices, distinguishing between legitimate and rogue entities. This advancement is pivotal for safeguarding IoT ecosystems from potential security threats.
Main Objectives
The primary aim of the research is to develop deep learning frameworks that can effectively classify LoRa device signals. By ensuring a clear differentiation between legitimate and rogue devices, these frameworks are crucial for protecting IoT networks from malicious actors. Additionally, the study implements adversarial attacks to evaluate the vulnerabilities of these classifiers, providing a deeper understanding of how such systems might be compromised.
Methodology
The researchers adopted a systematic approach, starting with the collection and processing of LoRa signal data. They developed deep learning models, including Deep Neural Networks (DNNs) and Convolutional Neural Networks (CNNs), to accurately classify these signals. To test the robustness of these classifiers, adversarial attack simulations were conducted. Following this, defense mechanisms were implemented to mitigate any identified vulnerabilities.
Key Findings
The study underscores the necessity for enhanced security protocols tailored specifically for IoT devices. It emphasizes that an awareness of adversarial machine learning is critical when designing these systems. Multi-task learning techniques were found to improve classification efficiency, facilitating better resource allocation within IoT networks. Furthermore, the researchers proposed a comprehensive framework for evaluating security risks, offering a structured approach to identifying and mitigating potential threats.
Tools and Techniques
Several tools and techniques were pivotal in achieving the study’s objectives:
- Fast Gradient Sign Method (FGSM): This technique was used to generate adversarial examples, testing the robustness of the classifiers.
- Adversarial Training: Employed to enhance classifier resilience against adversarial attacks by training on perturbed data.
- Kernel Density Estimation (KDE): Utilized for analyzing signal data distributions to improve classification accuracy.
- Hybrid Attack Strategy: Combined multiple attack vectors to thoroughly test the classifiers.
Implications
The implications of this research extend beyond theoretical advancements, potentially influencing practical cybersecurity strategies for IoT networks. By providing insights into enhanced adversarial training techniques and real-time anomaly detection systems, the findings could contribute significantly to establishing robust security measures. Moreover, recommendations for cross-platform security solutions might lead to more secure smart city infrastructures.
Strengths and Limitations
While presenting a comprehensive approach to enhancing LoRa network security, the research acknowledges certain limitations. These include the need for further investigation into the scalability of the proposed models and their applicability across diverse IoT environments. Future directions may involve exploring collaborative defense mechanisms and cross-domain transferability studies, offering a more holistic view of security in interconnected systems.
In summary, the work by Sagduyu and Erpek represents an important step forward in cybersecurity for IoT networks. Their research offers both theoretical insights and practical applications aimed at strengthening the integrity of LoRa communications. As these findings continue to evolve, they hold promise for shaping future strategies in securing IoT ecosystems against emerging threats.
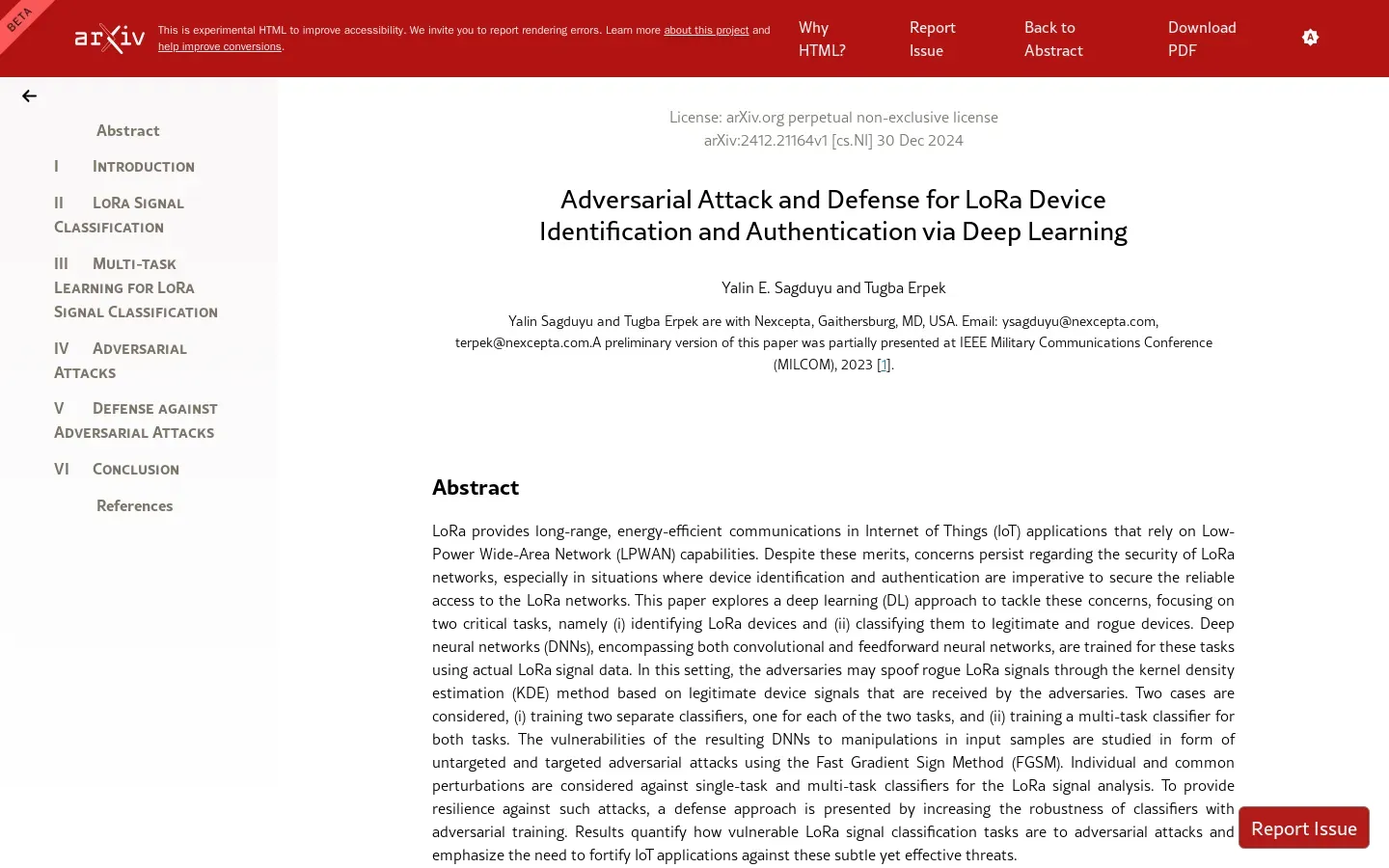