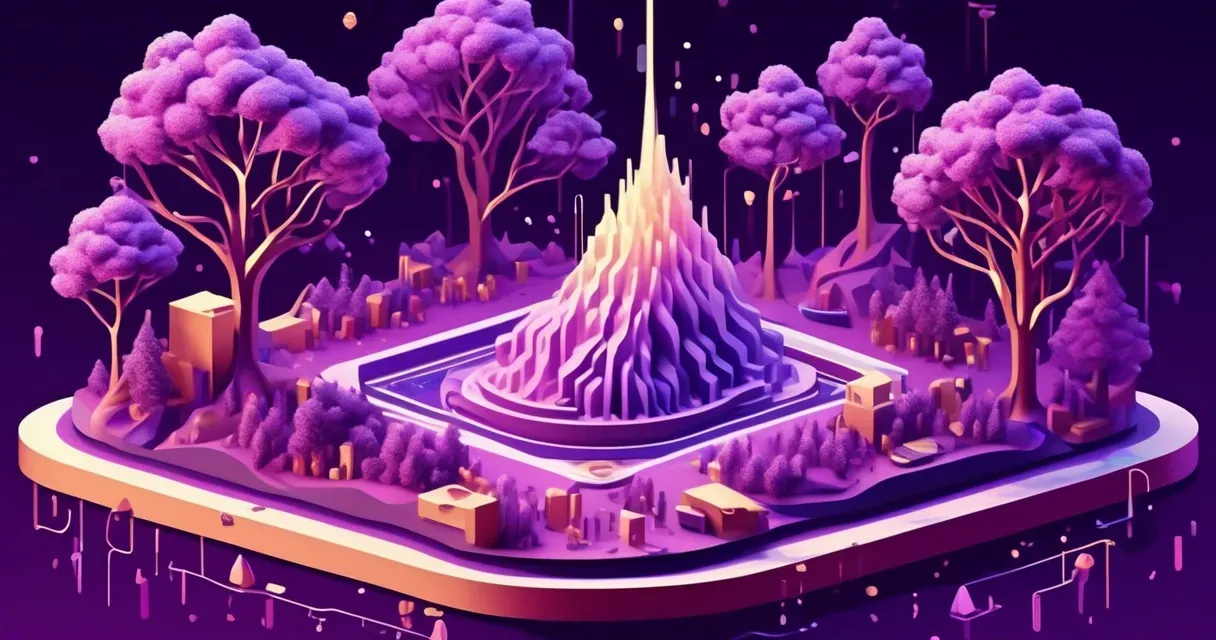
Multiparty Homomorphic Encryption Enhances Federated Survival Analysis
/ 4 min read
Quick take - Researchers have developed innovative privacy-preserving techniques for healthcare data sharing, particularly in oncology, utilizing multiparty homomorphic encryption and federated learning to enhance patient confidentiality while enabling collaborative analysis across institutions.
Fast Facts
- Researchers have developed innovative privacy-preserving techniques for healthcare data sharing, focusing on multiparty homomorphic encryption (MHE) and federated learning frameworks in oncology.
- The study demonstrated effective secure federated analytics, allowing institutions to collaborate on sensitive medical data without compromising patient confidentiality.
- Key findings include strategies to mitigate reconstruction attacks, scalability of federated Kaplan-Meier analysis, and the potential for real-time cybersecurity monitoring to enhance clinical decision support.
- The research emphasizes the importance of regulatory compliance and trust in data governance, facilitating collaboration between institutions and improving personalized medicine approaches.
- Future directions include expanding the federated framework for real-time data analysis and exploring cross-domain applications, such as fraud detection in financial services.
In an age where data is the new currency, the healthcare sector emerges as a critical frontier in the battle for privacy and security. As medical institutions increasingly collaborate to share vast amounts of sensitive patient information, the risks associated with potential data breaches and reconstruction attacks loom large. This landscape has prompted researchers to explore innovative techniques that ensure patient confidentiality while enabling essential data analytics. One such promising avenue lies in Secure Multi-Party Computation (SMPC), which facilitates secure data sharing without compromising individual privacy. Coupled with robust data encryption methodologies, like Multiparty Homomorphic Encryption (MHE), these advancements are not just theoretical musings; they are paving the way for practical applications in oncology and personalized medicine.
The integration of federated learning frameworks marks a significant leap forward in how we think about medical data analysis. By allowing multiple institutions to analyze shared datasets without ever exposing the raw data itself, researchers can derive insights while adhering to stringent regulatory guidelines like HIPAA. The Kaplan-Meier Estimator plays a crucial role here, providing a statistical method for survival analysis that works seamlessly within this federated framework. It allows for the assessment of patient outcomes without revealing identifiable patient information, thus striking a delicate balance between analytical rigor and privacy needs.
As researchers delve deeper into this realm, their investigations into vulnerabilities surrounding overlapping patient data among institutions have unveiled critical insights. The study’s findings indicate that employing advanced techniques can significantly mitigate reconstruction attacks—scenarios where adversaries attempt to extract sensitive information from aggregated datasets. The implications of this research extend beyond mere academic interest; they directly inform the development of robust cybersecurity measures necessary for safeguarding patient confidentiality across collaborative networks.
Yet, the journey does not end here. While the strengths of current methodologies are evident, several limitations beckon further exploration. For instance, implementing real-time analytical capabilities within these frameworks could greatly enhance clinical decision support systems. The ongoing evolution of privacy-preserving machine learning methods necessitates continuous refinement and adaptation to emerging threats, particularly in healthcare environments where stakes are exceptionally high.
Moreover, the scalability of secure computation techniques remains an area ripe for investigation. As healthcare data continues to grow exponentially, ensuring that these privacy-preserving technologies can handle increasing volumes without sacrificing performance is paramount. Additionally, fostering collaboration across diverse institutions requires robust policy and regulatory frameworks to build trust among stakeholders involved in data sharing initiatives.
Looking ahead, the future holds immense potential for leveraging these findings not only within healthcare but also across other domains such as financial services and fraud detection. The principles governing secure federated learning models may serve as foundational blueprints for addressing similar challenges in various sectors grappling with data privacy concerns.
The integration of anomaly detection mechanisms and machine learning models into federated frameworks could herald a new era of cybersecurity resilience. By focusing on real-world application testing within clinical settings, researchers will be able to assess the practicality and effectiveness of these methods within diverse environments.
As we stand on the brink of this exciting frontier, it becomes increasingly clear that the advancements in privacy-preserving techniques offer a beacon of hope for secure data sharing and analysis in healthcare—and perhaps beyond. As these methodologies evolve and expand, they promise not just enhanced security but also a more collaborative approach to tackling some of society’s most pressing health challenges in an increasingly interconnected world.