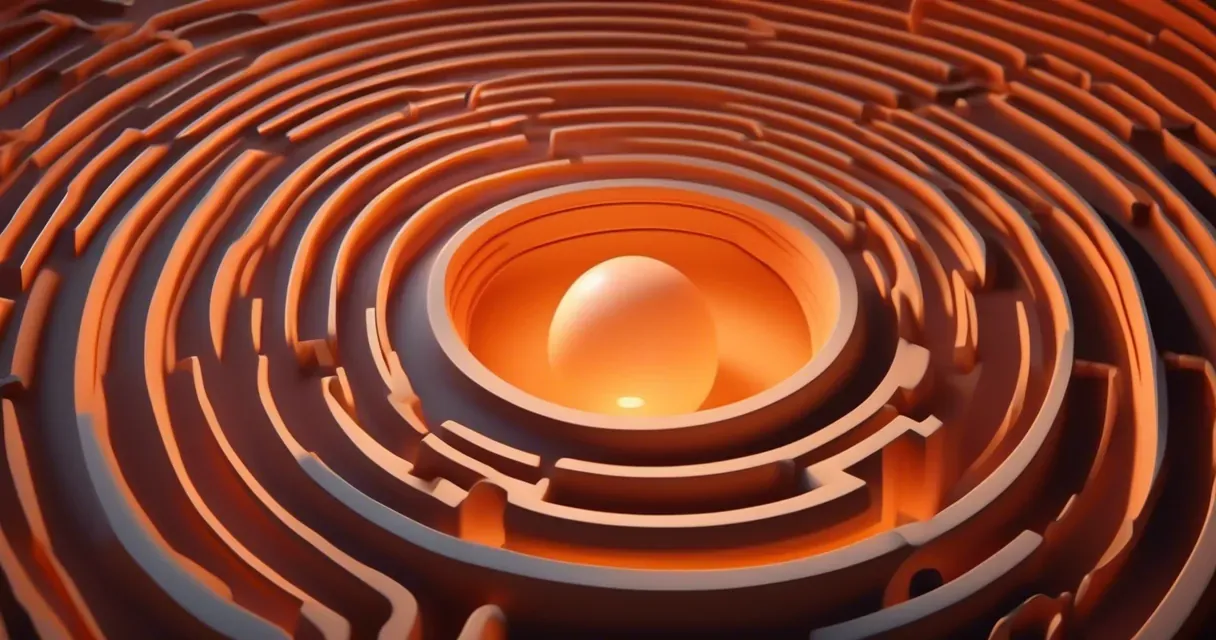
Enhancing Explainable AI for Adversarial Detection Systems
/ 4 min read
Quick take - Researchers have developed an enhanced Explainable Artificial Intelligence (XAI) framework, XReason, aimed at improving model interpretability and trust in real-time intrusion detection systems for cybersecurity, while also exploring user-centric interfaces and methodologies applicable across various industries.
Fast Facts
- Researchers enhanced Explainable AI (XAI) for real-time intrusion detection systems (IDS) using the XReason tool, improving model interpretability in cybersecurity.
- The study integrated advanced algorithms like LightGBM and SAT Solvers to provide immediate feedback on AI decisions during cyber threats.
- User-centric explainability interfaces were developed to make AI insights accessible, fostering trust between human operators and AI systems.
- The research offers a robust framework for understanding AI decision-making in cybersecurity, with implications for other industries like finance and healthcare.
- Future work will focus on expanding model support, improving adversarial sample handling, and refining explainability interfaces for diverse user needs.
In an age where cyber threats evolve at lightning speed, the quest for enhanced security measures relies heavily on artificial intelligence (AI) and machine learning (ML). As organizations increasingly turn to these technologies to bolster their defenses, the need for explainability in AI systems becomes paramount. The complexity of models like LightGBM and the intricacies of adversarial training necessitate a robust framework for interpreting their decisions. This is where the research surrounding the XReason tool for Explainable Artificial Intelligence (XAI) comes into play, offering insights that could redefine our approach to cybersecurity.
At its core, XReason aims to illuminate the black-box nature of AI systems, making it easier for cybersecurity professionals to understand how decisions are made in real-time. By integrating with real-time Intrusion Detection Systems (IDS), XReason not only enhances model transparency but also provides crucial insights into threat detection processes. This integration allows practitioners to respond more effectively to potential breaches by understanding the rationale behind alerts generated by AI systems.
An essential aspect of this research involves model support expansion, which broadens the types of models that can benefit from explainability features. By accommodating various frameworks, including those used in adversarial sample generation and detection, XReason paves the way for cross-domain applications of XAI. Cybersecurity experts can leverage this flexibility to tailor solutions that cater to specific operational needs while maintaining rigorous interpretability standards.
The study also delves into adversarial sample handling and defense mechanisms, highlighting a dual focus: improving detection and mitigation strategies against adversarial attacks. The findings suggest that implementing formal guarantees for model interpretability can significantly strengthen defenses by ensuring that AI systems remain trustworthy, even when faced with sophisticated manipulation techniques.
Another promising avenue discussed in the research is the development of user-centric explainability interfaces. These interfaces prioritize user experience, enabling cybersecurity professionals to interact with AI systems intuitively. By fostering a deeper understanding of algorithmic outputs through accessible visualizations and explanations, these tools can empower users to make informed decisions quickly during critical incidents.
Yet, as with any advancing field, limitations persist. Areas requiring further investigation include refining how models handle adversarial samples and evaluating their effectiveness across diverse environments. The ongoing evolution of cyber threats demands continuous improvement in these areas to ensure that AI systems do not just react but also adapt intelligently over time.
The strengths of this research lie not only in its practical applications but also in its forward-thinking approach. By pursuing enhancements and adjustments to tools like XReason, researchers are poised to contribute significantly to the cybersecurity landscape. The implications extend beyond immediate threat detection; they touch on broader applications within AI systems across various sectors, emphasizing the necessity for transparency and interpretability in all critical applications.
As we look ahead, fostering a culture of trustworthiness in AI will be indispensable. The exploration of explainable frameworks like XReason signifies a vital step toward that objective, promising a future where cybersecurity measures are not only effective but also comprehensible to those who deploy them. Engaging with these advancements will ultimately shape how organizations navigate the complex interplay between technology and security, ensuring resilience in an increasingly perilous digital world.