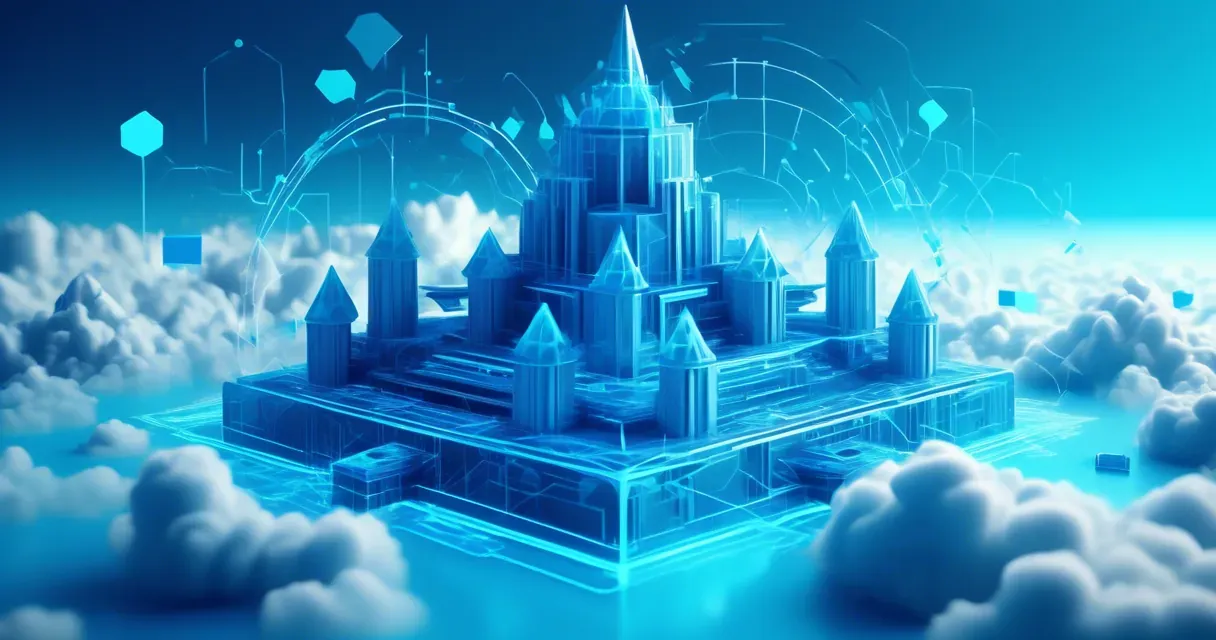
Innovative Framework Enhances Cloud Security Measures
/ 4 min read
Quick take - The research by Avradip Mandal and Meghna Sengupta proposes a comprehensive framework for enhancing data privacy in cloud environments through advanced security protocols, including federated learning and zero-trust architecture, while addressing the challenges of data management and compliance in various sectors.
Fast Facts
- Research Focus: Mandal and Sengupta propose a framework to enhance data privacy in cloud environments through advanced security protocols, federated learning, and zero-trust architecture.
- Methodology: The study introduces a novel tokenization algorithm for creating a secure data privacy vault, ensuring compliance with emerging security standards like IND-CATA and IND-SIA.
- Key Findings: Development of a zero-trust architecture compatible with cross-cloud solutions, emphasizing scalability, cost-effectiveness, and practical application in sensitive sectors like finance and healthcare.
- Implications: The framework significantly reduces risks of data breaches and unauthorized access, enhancing the resilience of cloud-based systems.
- Future Directions: Recommendations include refining access control mechanisms with AI and machine learning, and adapting the framework for various industries to meet specific regulatory needs.
In the ever-evolving landscape of cybersecurity, the need for robust data protection mechanisms has never been more pressing. As businesses increasingly rely on cloud-based solutions for their operations, the implications of sensitive data breaches loom larger than ever. The paper “Secure Vault Scheme in the Cloud Operating Model” by Avradip Mandal and Meghna Sengupta proposes groundbreaking advancements in secure data management practices that promise to reshape how organizations approach cloud security, particularly for analytics and machine learning applications. At the heart of this research lies an innovative tokenization algorithm designed to enhance data privacy while ensuring compliance with regulatory frameworks.
The integration of federated learning into this model represents a significant leap forward. By allowing multiple parties to collaboratively learn from shared data without directly accessing it, federated learning safeguards sensitive information while still enabling powerful analytics. This unique approach not only enhances data privacy but also reinforces the viability of cloud environments as secure repositories for sensitive information. Furthermore, the development of a formal framework is crucial, establishing a standardized methodology for implementing these security measures across varied platforms.
A key aspect of the proposed architecture is its compatibility with Zero-Trust Frameworks. In an era where threats can originate from both external and internal sources, Zero-Trust principles emphasize continuous verification and least privilege access. By aligning the secure vault scheme with these principles, organizations can significantly reduce their attack surface while enhancing overall security posture. This model also accommodates cross-cloud and multi-cloud security solutions, ensuring that diverse systems can work together seamlessly without compromising data integrity or confidentiality.
One promising avenue for future exploration is the implementation of sophisticated access control mechanisms utilizing tokenization schemes. This would allow organizations to create fine-grained access policies tailored to user roles, contextual requirements, and behavioral analytics. Such advancements could revolutionize how businesses manage access to sensitive data, enabling them to respond dynamically to evolving threats and user behaviors.
The research also delves into critical security notions such as IND-CATA (indistinguishability under chosen access and tampering attacks) and IND-SIA (indistinguishability under chosen input attacks). These concepts provide a theoretical foundation for assessing the robustness of the proposed tokenization algorithm against potential vulnerabilities. Additionally, scalability remains a pivotal concern; as organizations expand their cloud operations, they require cost-effective solutions that do not sacrifice security.
Despite these strengths, the study recognizes certain limitations that warrant further investigation. The reliance on a Random Oracle Model (ROM) raises questions about practical applicability—while theoretically sound, its real-world effectiveness must be evaluated through rigorous testing and analysis. Moreover, enhancing access control mechanisms will require ongoing refinement to adapt to emerging threats in a constantly shifting digital landscape.
The promise of deterministic tokenization for large language model training presents another exciting area for future research. As machine learning becomes more pervasive, ensuring that training datasets remain secure while providing valuable insights will be crucial for maintaining trust in AI-driven applications. Likewise, addressing compliance issues within regulatory frameworks will be vital as governments worldwide tighten their grip on data privacy laws.
As we look ahead, the implications of these findings are profound. The integration of advanced security frameworks within cloud operating models signals a transformative shift towards more resilient data management practices. Organizations willing to embrace these changes will not only enhance their defense against cyber threats but also foster greater trust among stakeholders who demand accountability in how their data is handled. In this rapidly changing environment, those who prioritize innovative security solutions will undoubtedly lead the way into a safer digital future.