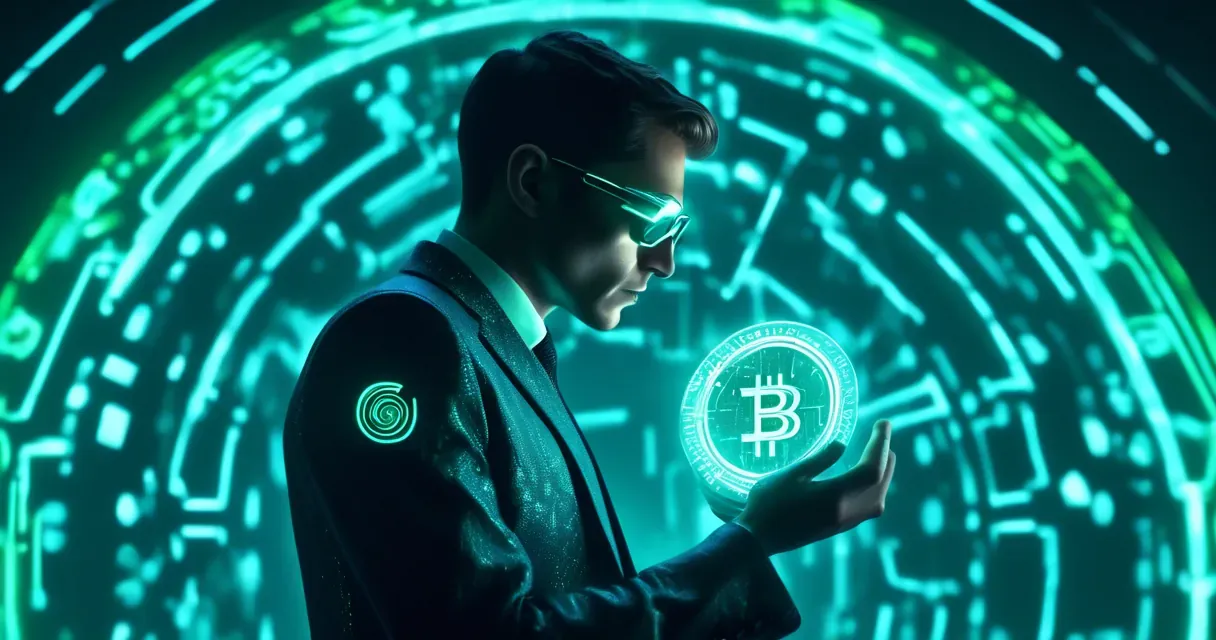
Innovative Methods for Detecting Financial Bots on Ethereum
/ 4 min read
Quick take - Recent research has advanced bot detection in the Ethereum ecosystem through machine learning techniques, establishing a framework for real-time monitoring and regulatory compliance while highlighting the need for user protection and future developments in cybersecurity.
Fast Facts
- Objective: The research aimed to develop a framework for detecting financial bots in the Ethereum ecosystem, focusing on bot behavior, classification, and creating a ground-truth dataset.
- Methodologies: Utilized machine learning techniques like Random Forest and Gaussian Mixture Model (GMM) for classification, along with UMAP for dimensionality reduction and improved data visualization.
- Key Findings: Established a taxonomy of financial bots, demonstrated real-time detection capabilities, and highlighted the integration of detection frameworks with regulatory compliance tools.
- Implications: The research enhances user protection and regulatory oversight, contributing to a safer trading environment by improving understanding and monitoring of bot activities.
- Future Directions: Suggested development of user education programs, further investigation into clustering techniques, and expansion of bot detection frameworks to other blockchain platforms.
In today’s rapidly evolving digital landscape, the proliferation of automated systems, particularly financial bots operating within Distributed Ledger Technologies (DLTs), poses significant challenges for cybersecurity professionals. As these bots infiltrate platforms like Ethereum and manipulate market dynamics, the need for sophisticated detection mechanisms becomes paramount. The latest research into this arena not only sheds light on the intricacies of bot behavior but also offers a comprehensive framework for understanding and mitigating their impact.
At the heart of this investigation lies the classification of bots, a cornerstone for effective monitoring and regulation. By developing a taxonomy of financial bots, researchers have established a foundational framework that categorizes various types, enabling cybersecurity experts to identify and target specific threats. This classification is bolstered by the creation of a ground-truth dataset, which serves as an essential reference point for future studies and enhances the accuracy of detection methodologies.
To tackle these challenges, the research introduces several cutting-edge tools and techniques aimed at enhancing bot detection in real-time trading platforms. Among them is the Gaussian Mixture Model (GMM), utilized for its ability to discern patterns within complex datasets. This statistical model excels in identifying anomalies indicative of bot activity, allowing for proactive measures to be implemented before significant damage occurs. Coupled with machine learning model deployment strategies, these frameworks provide robust solutions that adapt to evolving threats in real time.
Another pivotal tool discussed is dimensionality reduction with UMAP, which enhances clustering performance by simplifying data while retaining essential features. This technique proves invaluable in analyzing vast amounts of trading data, ensuring that relevant bot behaviors are accurately captured and classified. By integrating such advanced analytical methods, researchers can better understand bot interactions within the Ethereum ecosystem.
The study also emphasizes the importance of integration with regulatory compliance tools. As financial markets grow increasingly scrutinized under regulatory frameworks, aligning bot detection mechanisms with these compliance tools ensures that organizations remain vigilant against illicit activities. This integration fosters transparency and accountability, crucial components in building trust within digital finance.
Despite these advancements, there remain limitations that necessitate further investigation. Current methodologies often struggle with real-time monitoring and response systems, leaving gaps in immediate threat detection capabilities. Additionally, while feature engineering has significantly progressed, continuous enhancement in this area is vital to keep pace with sophisticated bot techniques.
Looking ahead, the implications of these findings extend beyond mere detection; they pave the way for enhanced user protection mechanisms and comprehensive education programs aimed at raising awareness about cyber threats. As financial systems become more intertwined with technology, educating users on potential risks associated with bots will play a critical role in fostering a more secure digital environment.
In summary, as researchers delve deeper into the complexities of bot activity within DLTs like Ethereum, they lay the groundwork for innovative applications of machine learning-based detection methods in cybersecurity. The integration of advanced frameworks combined with regulatory compliance will not only enhance security measures but also prepare organizations for an increasingly automated future where vigilance is paramount. The continued evolution of this field promises to transform how we approach cybersecurity challenges in finance and beyond.