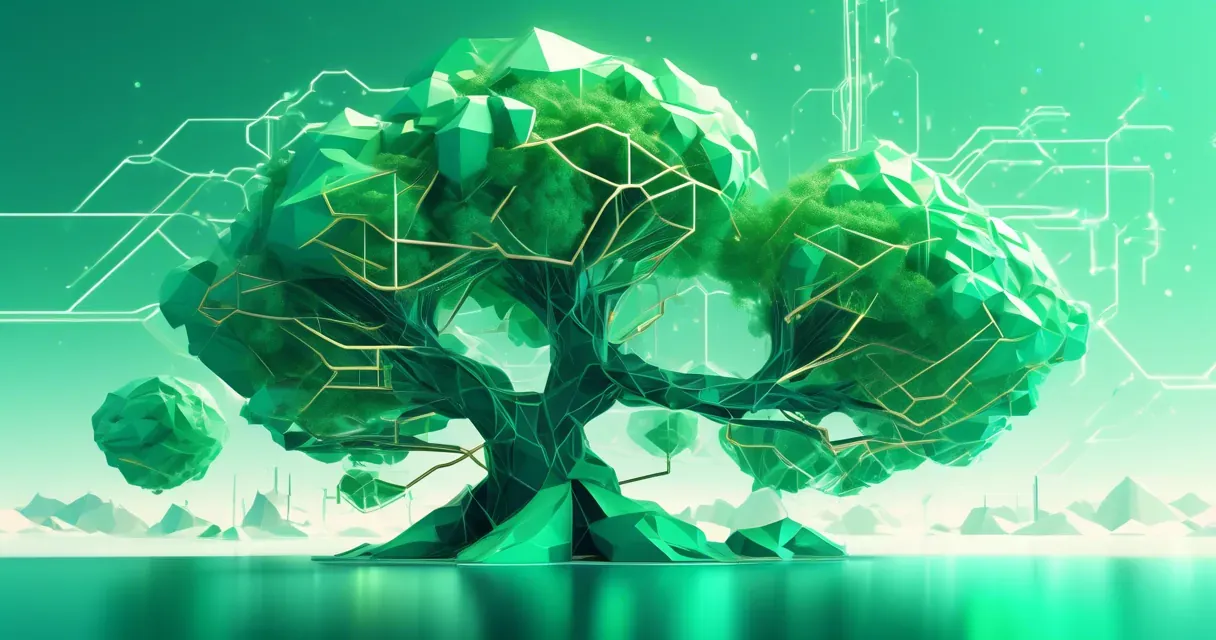
Quantum Learning Framework Enhances Cybersecurity and Data Security
/ 4 min read
Quick take - Researchers have developed a Quantum Label Encoding Framework that enhances data security and machine learning outcomes against eavesdropping threats, demonstrating the potential of quantum technologies in improving cybersecurity measures and intrusion detection systems.
Fast Facts
- Quantum Label Encoding Framework: Developed to enhance data security and improve machine learning outcomes against eavesdropping threats.
- Robustness Against Eavesdropping: Demonstrated that quantum protocols can mitigate risks from eavesdroppers, ensuring secure federated learning.
- Integration of CNNs: Successfully applied Convolutional Neural Networks to improve anomaly detection in intrusion detection systems.
- Novel Learning Probability Evaluation: Introduced a new method for optimizing learning outcomes in quantum environments.
- Future Directions: Calls for further research on quantum secure communication protocols and enhancing federated learning against advanced attack strategies.
In the ever-evolving landscape of cybersecurity, where threats grow more sophisticated by the day, researchers are turning to the frontiers of quantum computing to bolster defenses against malicious actors. A recent exploration into Quantum Label Encoding has unveiled a framework that not only enhances data security but also presents intriguing possibilities for machine learning applications in intrusion detection and anomaly identification. The integration of quantum principles into cybersecurity aims to redefine how we approach data integrity and secure communications, particularly in a world increasingly susceptible to eavesdropping.
At the core of this research is the Quantum Label Encoding Framework, which revolutionizes traditional methods by allowing for more robust encoding of information. This advancement is particularly crucial in the context of Convolutional Neural Networks (CNNs), which have long been employed in various machine learning tasks, including image recognition and data classification. By implementing quantum-enhanced methodologies within CNN architectures, the research demonstrates a notable increase in both learning efficiency and accuracy when detecting anomalies in network security.
The implications extend beyond mere performance enhancements. The study delves into the Probably-Approximately-Correct (PAC) Learning Framework, establishing conditions under which authorized learners can achieve guaranteed superior outcomes compared to potential eavesdroppers. This focus on Eavesdropping Impact Assessment sheds light on how quantum protocols can protect sensitive data during transmission, ensuring that even if an attack occurs, the integrity of the learning process remains intact.
One standout feature of this research is its proposal for Quantum Secure Machine Learning Frameworks that integrate secure federated learning models. This approach allows multiple parties to collaboratively train machine learning models without compromising their individual datasets, thus paving the way for collective intelligence while maintaining strict privacy controls. The concept of a Collective Attack Strategy (𝒮E(𝒫)) further underscores the need for novel defensive tactics against coordinated cyber threats; it highlights the importance of understanding attacker behavior in a probabilistic manner.
Yet, while these advancements present promising pathways toward strengthening cybersecurity frameworks, they are not without limitations. The current Quantum Data Transmission Protocol (𝒫) requires thorough assessment to evaluate its practical applicability across varying real-world scenarios. Furthermore, there remains a pressing need for theoretical proofs and conditions that underpin these new methodologies—ensuring that they can stand up against future challenges posed by adversaries equipped with equally advanced technologies.
As researchers continue to validate these frameworks through rigorous testing and application in real-world contexts, there is a clear trajectory towards developing quantum-resistant algorithms capable of withstanding emerging threats. Innovations in quantum machine learning may soon become pivotal in threat intelligence, empowering organizations to not only detect intrusions with greater precision but also respond proactively to potential breaches.
Looking ahead, the intersection of quantum computing and cybersecurity presents an exhilarating frontier filled with opportunities and challenges alike. As this field matures, it will undoubtedly influence the next generation of cyber defense mechanisms—leveraging quantum principles not just as a theoretical exercise but as essential tools in safeguarding our digital environments against an increasingly hostile landscape. With every breakthrough, we inch closer to realizing a future where security measures are as advanced as the threats they seek to neutralize.