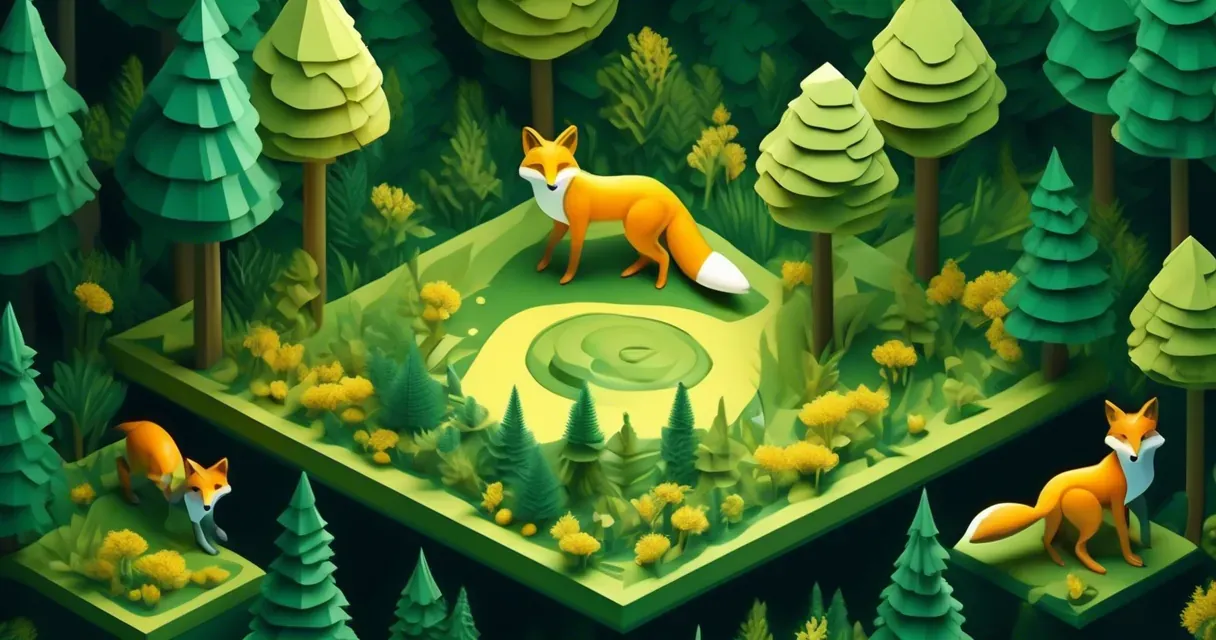
Backdoor Attacks Identified in Android Deep Learning Models
/ 3 min read
Quick take - Recent research has explored the vulnerabilities of deep learning systems to backdoor attacks, particularly in mobile and safety-critical applications, while developing detection tools and strategies to enhance cybersecurity.
Fast Facts
- Backdoor Attack Vulnerabilities: Research highlights significant risks of backdoor attacks in deep learning systems, especially in mobile applications and safety-critical environments.
- Detection and Mitigation Tools: Development of advanced frameworks like DeepInspect and BARWM aims to identify and counteract backdoor threats effectively.
- User Awareness Initiatives: Emphasis on user-centric security programs to educate developers and users about backdoor attack risks and defenses.
- Cross-Domain Strategies: Exploration of mitigation strategies across different application domains to enhance the effectiveness of defenses against backdoor attacks.
- Future Directions: Recommendations include integrating detection mechanisms into AI deployment pipelines and enhancing security frameworks specifically for mobile applications.
In an era where artificial intelligence (AI) and machine learning (ML) are rapidly reshaping industries, cybersecurity remains a pivotal concern. The rise of sophisticated backdoor attacks in deep learning systems poses significant threats not just to individual organizations but also to the integrity of AI applications globally. As researchers delve deeper into the mechanics of these attacks, a clearer picture emerges—one that underscores both the vulnerabilities and the avenues for enhanced defenses within this evolving landscape.
Automated model auditing tools have surfaced as a vital development in the fight against backdoor attacks. These tools serve as digital watchdogs, meticulously scrutinizing AI models for potential vulnerabilities introduced during training phases. Among them, DeepInspect shines by offering a robust framework for model extraction and analysis, while Sentinet focuses on safeguarding mobile applications against rising risks. The Neural Cleanse technique further fortifies defenses by inspecting models for hidden trojans, ensuring that malicious backdoors remain undetected. Yet, despite these advancements, there’s a glaring need for enhanced model protection mechanisms and user-centric security awareness programs that can preemptively address potential breaches.
The implications of research surrounding backdoor attacks using DNN-based steganography reveal profound insights for cybersecurity strategies. This method not only exploits existing vulnerabilities but also raises questions about the integrity of AI in safety-critical systems. As we explore methods like BARWM, which targets real-world models, it becomes clear that there’s both urgency and opportunity in developing robust detection frameworks specifically tailored for federated learning environments. These models must integrate backdoor detection within deployment pipelines to mitigate risks systematically.
But challenges persist. The limitations identified in current methodologies highlight areas requiring further investigation, particularly concerning restoration and defense strategies against trojan backdoors. Developing comprehensive approaches that inspect and identify these hidden threats remains paramount. Additionally, cross-domain research into backdoor attack mitigation strategies could yield critical insights into how different sectors can share knowledge and tools to fortify their defenses.
As organizations increasingly recognize the importance of user education, implementing effective training programs for developers is essential. Programs that emphasize awareness and understanding of backdoor mechanisms can empower teams to create more secure AI applications from the ground up. Coupled with practical initiatives like Tabor—a framework designed to enhance mobile security—these educational efforts can cultivate a proactive security culture.
Looking ahead, the future of cybersecurity in an AI-driven world lies in leveraging these findings to build resilient infrastructures capable of thwarting complex cyber threats. By focusing on multi-faceted approaches that blend technical solutions with organizational practices, the industry can foster a safer environment for deploying innovative technologies. As researchers continue to unravel the intricacies of deep learning vulnerabilities, the hope is that the next wave of solutions will not only safeguard our current systems but also pave the way for more secure AI applications in the years to come.