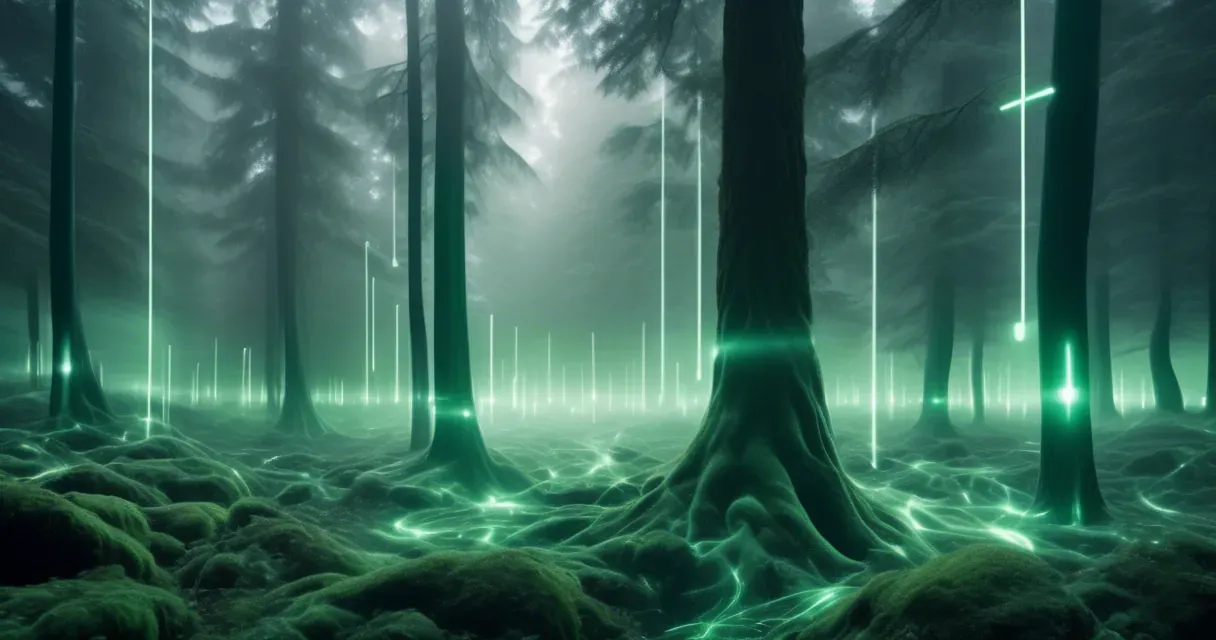
BARTPredict Enhances IoT Security with Machine Learning Techniques
/ 4 min read
Quick take - The research presented at CICIoT2023 introduces an advanced intrusion prediction framework for IoT networks that utilizes machine learning techniques to enhance cybersecurity by analyzing user behavior and network traffic patterns, while also emphasizing the importance of collaborative threat intelligence sharing and real-time threat detection.
Fast Facts
- The CICIoT2023 conference introduced a novel intrusion prediction framework for IoT networks, utilizing advanced machine learning techniques like BART and LLMs to enhance cybersecurity.
- The research focused on User Behavior Analytics (UBA) and Multi-Stage Attack Prediction, integrating edge computing to improve detection and response times to threats.
- Key findings revealed that UBA improved anomaly detection in user interactions, while the multi-stage model accurately forecasted various attack vectors, enhancing proactive defenses.
- The framework promotes collaborative threat intelligence sharing among IoT devices, leading to quicker dissemination of threat information and reduced latency for real-time threat detection.
- Future research is needed to address dataset diversity and explore the framework’s scalability across different IoT environments, aiming for improved prediction accuracy.
In an era where the Internet of Things (IoT) is not just a buzzword but a transformative force in various industries, the implications for cybersecurity have never been more pressing. With billions of devices interconnected, the potential for vulnerabilities increases exponentially. The recent CICIoT2023 IoT Attack Dataset has shed light on this critical issue, providing researchers with a robust framework to analyze user behavior and patterns within IoT environments. This dataset serves as a cornerstone for understanding how malicious actors exploit these systems and highlights the urgency for enhanced protective measures.
One of the significant advancements discussed in the research is User Behavior Analytics (UBA). UBA employs intricate algorithms to understand typical usage patterns and identify anomalies that may indicate security breaches. This approach leverages machine learning techniques, particularly Bidirectional and Auto-Regressive Transformers (BART), which are designed to understand context and predict user actions effectively. By analyzing data from multiple angles, BART enables a comprehensive view of user interactions, enhancing detection capabilities across IoT devices.
The landscape of intrusion detection is evolving with the introduction of multi-stage attack prediction methodologies. These frameworks allow for real-time analysis and proactive defense strategies, transforming how organizations respond to threats. The research emphasizes the importance of deploying these predictive models at Multi-Access Edge Computing (MEC) servers, optimizing response times and reducing latency in threat detection. With this strategic placement, organizations can achieve faster insights, thus fortifying their defenses against sophisticated attacks.
Beyond individual device security, the findings advocate for cross-domain security applications. By fostering collaborative threat intelligence sharing among organizations, stakeholders can pool resources and knowledge to combat emerging threats more effectively. This collective approach not only enhances situational awareness but also empowers entities to anticipate attacks before they materialize.
The integration of advanced machine learning techniques is pivotal in enhancing cybersecurity measures within IoT networks. Notably, the evaluation of predictions using BERT (Bidirectional Encoder Representations from Transformers) demonstrates how fine-tuning large language models (LLMs) can lead to improved next packet prediction accuracy. This level of foresight is crucial, as it allows systems to adaptively respond to potential threats based on learned behaviors rather than solely relying on predefined rules.
Despite these advancements, there are limitations that warrant further investigation. The reliance on packet parsing and pre-processing can introduce challenges in accurately identifying threats if not executed meticulously. Moreover, while the HuggingFace Transformers Library provides powerful tools for implementing these models, the complexity of IoT environments means that continuous refinement and adaptation will be necessary to maintain efficacy.
As we look ahead, the implications of this research extend beyond theoretical frameworks; they offer practical pathways for organizations striving to secure their IoT infrastructures. The convergence of AI-driven analytics with real-time data processing at edge nodes presents a promising frontier in cybersecurity. Future developments will likely focus on refining these models and exploring new avenues for collaboration across sectors, ultimately crafting a more resilient digital landscape against an ever-evolving threat landscape. As we embrace these innovations, it becomes clear that staying ahead of cyber adversaries requires an unwavering commitment to adaptation and vigilance in our security strategies.