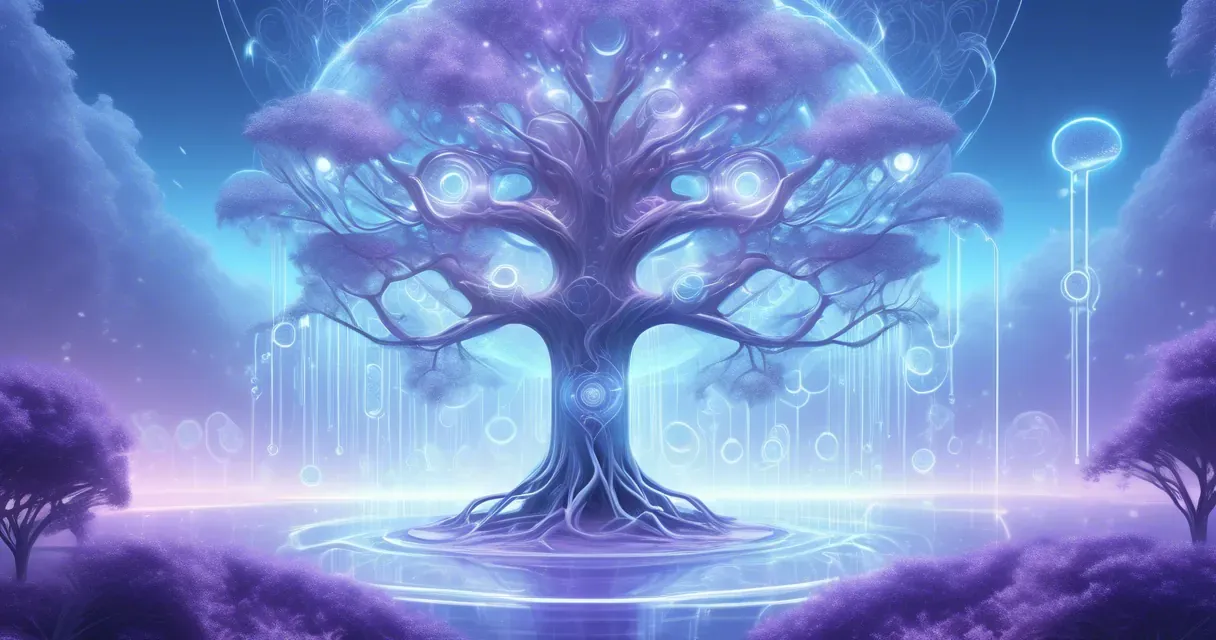
CySecBench Dataset Aims to Enhance Cybersecurity Evaluations
/ 4 min read
Quick take - Researchers have developed innovative jailbreaking methodologies to assess and enhance the security of large language models (LLMs) in cybersecurity, revealing vulnerabilities and proposing future directions for improved safety protocols.
Fast Facts
- Researchers developed innovative jailbreaking methodologies to enhance cybersecurity by investigating vulnerabilities in large language models (LLMs).
- The study utilized the CySecBench dataset to evaluate LLM vulnerabilities and employed generative pre-trained transformers (GPT) for initial jailbreaking techniques.
- Key advancements included prompt obfuscation to evade safety filters and multi-model refinement to improve response generation under security constraints.
- The research emphasizes the need for ethical AI use and the development of tailored security protocols, highlighting the importance of understanding model vulnerabilities.
- Future directions include creating domain-specific datasets and refining jailbreaking methodologies to strengthen AI resilience against threats across various sectors.
In an era dominated by rapid technological advancements, the intermingling of artificial intelligence and cybersecurity has engendered both opportunities and challenges. With the rise of Generative Pre-trained Transformers (GPT) and similar language models (LLMs), organizations face a dual-edged sword: while these tools can streamline operations and enhance user experiences, they also present new vulnerabilities that adversaries are keen to exploit. Recent research highlights significant strides in jailbreaking methodologies, illustrating how attackers refine their techniques to bypass safety filters embedded within LLMs. As we delve deeper into this evolving landscape, it becomes crucial to understand the strengths and limitations of current methodologies and explore future directions for bolstering cybersecurity defenses.
The study’s foundation rests on the CySecBench Dataset, a pioneering effort designed to assess LLM vulnerabilities effectively. By generating domain-specific datasets tailored for industries with unique security challenges, researchers can better scrutinize how LLMs respond to varied prompts. This approach not only enhances our understanding of model vulnerabilities but also underscores the necessity for customized evaluation frameworks. The dataset’s development marks a crucial step toward ensuring that LLMs can withstand sophisticated attack vectors, particularly in sectors where data integrity is paramount.
As attackers continuously innovate, the advancements in jailbreaking techniques warrant attention. The research outlines two notable enhancements: prompt obfuscation and multi-model refinement. Prompt obfuscation aims to disguise malicious inputs, making it increasingly difficult for LLMs’ safety mechanisms to detect threats. On the other hand, the multi-model refinement process involves using various model architectures to identify weaknesses across different systems, ultimately improving overall resilience against exploitation.
A key finding from this investigation is the pressing need for automated evaluation processes that leverage threat intelligence integration. By automating the assessment of LLMs against evolving threats, organizations can stay ahead of potential breaches. This approach not only streamlines evaluations but also provides insights into emerging vulnerabilities that might otherwise go unnoticed.
Nevertheless, the research acknowledges several limitations and areas for further exploration. While advancements in jailbreaking methodologies are commendable, they highlight an ongoing cat-and-mouse game between developers and attackers. There is a call for the development of enhanced security protocols specifically designed for LLMs, which could mitigate risks associated with prompt manipulation and other vulnerabilities. Additionally, educational tools for cybersecurity training must evolve to include real-world scenarios involving AI-driven threats, preparing professionals to address these challenges head-on.
Looking ahead, the implications for ethical AI use cannot be overstated. As organizations increasingly rely on AI-driven solutions, ensuring their safety becomes paramount. The study advocates for cross-domain applications of dataset generation methodologies to foster collaboration among industries facing similar security threats. Enhanced evaluation metrics will also play a critical role in assessing model vulnerability more comprehensively.
In conclusion, while the advancements in jailbreaking methodologies signal a growing sophistication among cyber adversaries, they also illuminate pathways for robust defense mechanisms. The intersection of AI and cybersecurity necessitates ongoing research and innovation; as we refine our approaches to threat detection and mitigation, the future holds promise for building resilient systems capable of withstanding even the most advanced attacks. The journey toward securing our digital landscapes continues, emphasizing the importance of vigilance and adaptability in this ever-evolving field.