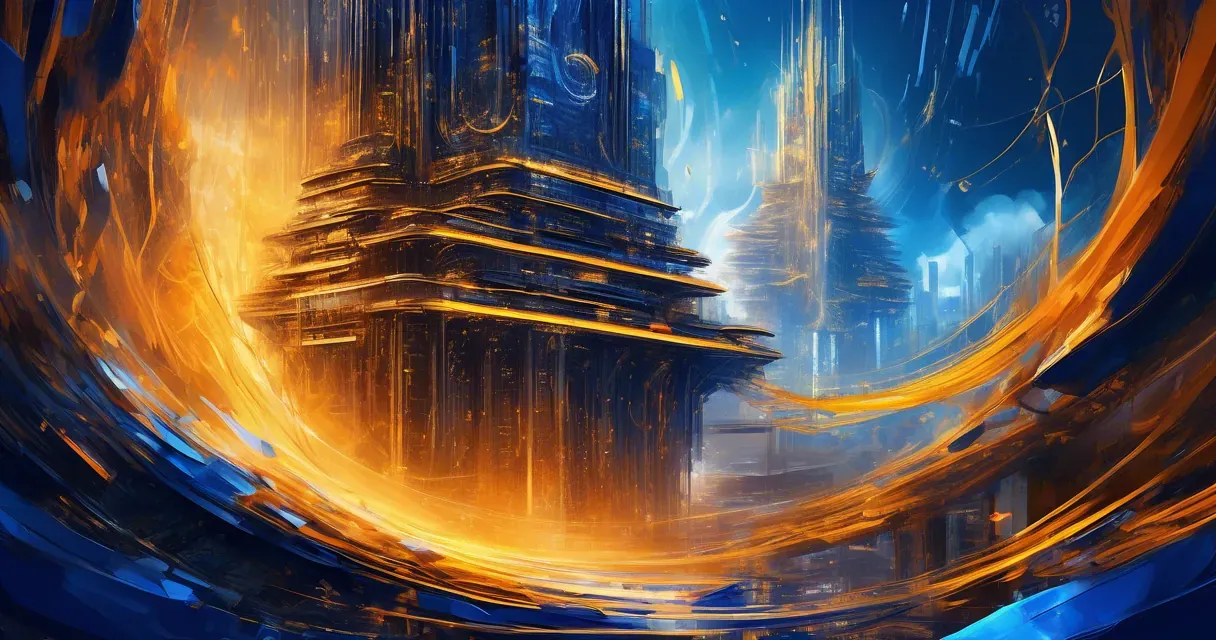
HoneypotNet Develops Defense Against Model Extraction Attacks
/ 4 min read
Quick take - HoneypotNet is a newly developed defense mechanism aimed at protecting machine learning models from model extraction attacks, demonstrating effective strategies and methodologies for enhancing cybersecurity in cloud-based ML applications.
Fast Facts
-
Introduction of HoneypotNet: A new defense mechanism aimed at protecting machine learning models from model extraction attacks, which involve adversaries replicating proprietary models.
-
Research Objectives: The study evaluated HoneypotNet’s effectiveness in cloud-based Machine Learning as a Service (MLaaS) platforms, using empirical methods like trigger generation and backdoor detection.
-
Key Findings: HoneypotNet employs strategies like Honeypot Layer Replacement to mislead attackers, and Reconstructive Neuron Pruning (RNP) to defend against neuron pruning attacks, with optimal trigger configurations enhancing protection.
-
Tools and Techniques: The research utilized adaptive defense mechanisms, KnockoffNets for generating substitute models, and Universal Adversarial Perturbation (UAP) to assess robustness against adversarial attacks.
-
Future Directions: The study suggests exploring Cognitive Distillation and collaboration with regulatory frameworks to improve model protection practices and enhance user awareness of model extraction risks.
In an era where machine learning (ML) is revolutionizing industries, the protection of these sophisticated models has become paramount. As organizations increasingly rely on ML to drive decision-making and automate processes, they inadvertently expose themselves to a growing range of cyber threats, particularly model extraction attacks. These attacks not only compromise the integrity of ML systems but also pose significant risks to sensitive data and intellectual property. To combat these challenges, researchers have developed innovative strategies, notably the HoneypotNet defense mechanism, which integrates principles from backdoor attacks with ownership verification techniques.
Cognitive Distillation (CD) emerges as a promising approach within this framework, focusing on minimizing the risk of information leakage while enhancing the robustness of ML models. By distilling knowledge from a model’s layers into a more compact form, CD helps limit what adversaries can extract during an attack. This method aligns closely with ongoing research to improve transferability across various model architectures, ensuring that defenses remain effective regardless of the specific configurations employed by attackers.
A pivotal aspect of HoneypotNet’s strategy is its ability to analyze trigger size and protection effect. Research highlights that smaller triggers are often less detectable yet can still yield significant insights into the underlying model, making them a focal point for future investigations. This interplay between trigger characteristics and model security underscores the need for continuous refinement of defensive techniques, particularly in cloud-based Machine Learning as a Service (MLaaS) platforms where vulnerabilities may be exploited at scale.
Tools like Backdoor Detection and Evasion play crucial roles in identifying potential threats posed by malicious entities seeking to manipulate model behavior. Similarly, techniques such as Universal Adversarial Perturbation (UAP) provide insights into how even small, seemingly innocuous changes can lead to substantial shifts in model outputs. By leveraging these methodologies, HoneypotNet aims to create a more resilient architecture against both direct attacks and indirect threats arising from nuanced manipulations.
Empirical validation remains essential in establishing HoneypotNet’s efficacy in real-world applications. The research indicates a strong correlation between robust defense mechanisms and adaptive frameworks capable of responding dynamically to emerging threats. For instance, Reconstructive Neuron Pruning (RNP) not only enhances model efficiency but also fortifies defenses against extraction tactics aimed at compromising operational integrity.
Looking ahead, there are compelling areas for further exploration within this research landscape. Collaborative efforts with regulatory frameworks could pave the way for standardizing security measures across industries reliant on machine learning technologies. Additionally, user education and awareness are critical components if organizations hope to foster a culture of security that extends beyond technical implementations. Educating stakeholders about the implications of model extraction attacks is vital in reinforcing the importance of adopting comprehensive protective measures.
As we move forward into an increasingly interconnected digital landscape, the implications of these findings are profound. The evolution of HoneypotNet represents just one facet of a broader movement toward enhanced model protection mechanisms that prioritize resilience in face of adversity. The integration of advanced techniques such as bi-level optimization (BLO) and shadow modeling further enriches the toolkit available to cybersecurity professionals. Ultimately, by acknowledging and addressing these vulnerabilities proactively, we can shape a future where machine learning models not only thrive but do so within secure environments resilient against exploitation.