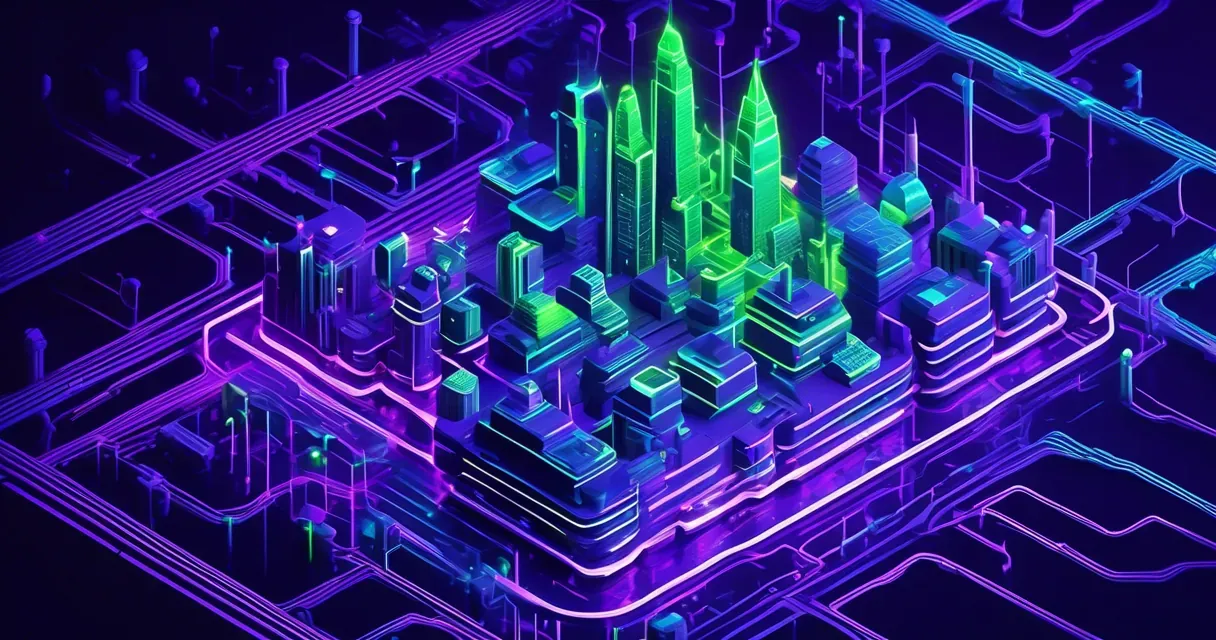
LENS-XAI Framework Advances Intrusion Detection in Cybersecurity
/ 4 min read
Quick take - The LENS-XAI framework is a newly developed approach aimed at improving anomaly detection in cybersecurity, particularly in resource-constrained environments, by utilizing advanced machine learning techniques and enhancing explainability to foster trust in AI systems.
Fast Facts
- The LENS-XAI framework aims to improve anomaly detection in cybersecurity, particularly in resource-constrained environments, using advanced machine learning techniques like Variational Autoencoders (VAEs).
- Key features include enhanced explainability through variable attribution methods, which help stakeholders understand AI decision-making processes, fostering trust in intrusion detection systems (IDS).
- The framework demonstrates improved anomaly detection capabilities and integrates federated learning for real-time threat intelligence sharing while maintaining data privacy.
- Despite its strengths, challenges remain in scalability and efficiency, with future research needed in unsupervised learning and knowledge distillation techniques.
- The ongoing development of LENS-XAI is crucial for enhancing cybersecurity resilience against evolving threats, with potential applications in autonomous systems and cross-domain security.
In the ever-evolving landscape of cybersecurity, organizations are increasingly pressed to fortify their defenses against a barrage of sophisticated threats. As traditional intrusion detection systems (IDS) struggle to keep pace with these dynamic adversities, innovative frameworks like the Lightweight Explainable Network Security framework (LENS-XAI) emerge as beacons of hope. By harnessing advanced machine learning techniques, particularly variational autoencoders (VAEs), LENS-XAI offers enhanced anomaly detection capabilities that promise to redefine the standards of cybersecurity.
The research surrounding LENS-XAI highlights its strengths in several key areas. At the forefront is its ability to operate effectively in resource-constrained environments. With computational efficiency at its core, LENS-XAI is designed to tackle the challenges posed by limited resources without sacrificing performance. This capability not only broadens its applicability but also paves the way for real-time threat intelligence sharing—an essential feature in today’s fast-paced digital environment.
Yet, as promising as LENS-XAI appears, it is not without limitations. One significant hurdle lies in its scalability and efficiency when faced with increasingly complex datasets. The reliance on unsupervised and semi-supervised learning techniques necessitates further investigation; future explorations could delve into how these models can better adapt when labeled data is scarce. Additionally, while LENS-XAI excels at identifying anomalies, its ability to provide clear, actionable insights remains a critical area for development. Real-time explainability optimization must evolve hand-in-hand with detection capabilities to foster trust among stakeholders and ensure effective decision-making.
A notable strength of the LENS-XAI framework stems from its integration with federated learning and edge computing, which enhances user privacy while maintaining robust security protocols. This integration not only addresses privacy concerns but also enables dynamic adaptation to an evolving threat landscape—a crucial requirement as cyber attackers continuously refine their tactics. The promise of variable attribution-based explainability further accentuates this framework’s potential; by elucidating why certain decisions are made, organizations can cultivate a deeper trust in automated systems.
The methodology employed in developing LENS-XAI is equally commendable. It emphasizes standardization through Z-score normalization—a technique vital for balancing gradient updates during model training and improving convergence rates across various algorithms. Such meticulous attention to data preprocessing and feature engineering underscores a commitment to quality that is paramount in cybersecurity applications.
As we explore future directions for the LENS-XAI framework, several avenues beckon further inquiry. The potential for cross-domain security applications is vast; integrating LENS-XAI into sectors such as autonomous systems or IoT devices could amplify its impact on safeguarding critical infrastructure against emerging cyber threats. Furthermore, incorporating lightweight knowledge distillation techniques may allow for even more streamlined operations without compromising performance integrity.
Looking ahead, the ramifications of advancing frameworks like LENS-XAI are profound. As organizations navigate an increasingly interconnected world fraught with cyber risks, the need for scalable, interpretable, and efficient solutions will only grow. By pursuing these investigative paths and refining current methodologies, LENS-XAI—and similar frameworks—could play a pivotal role in reshaping cybersecurity practices, ultimately enhancing resilience against the intricate tapestry of modern cyber threats.