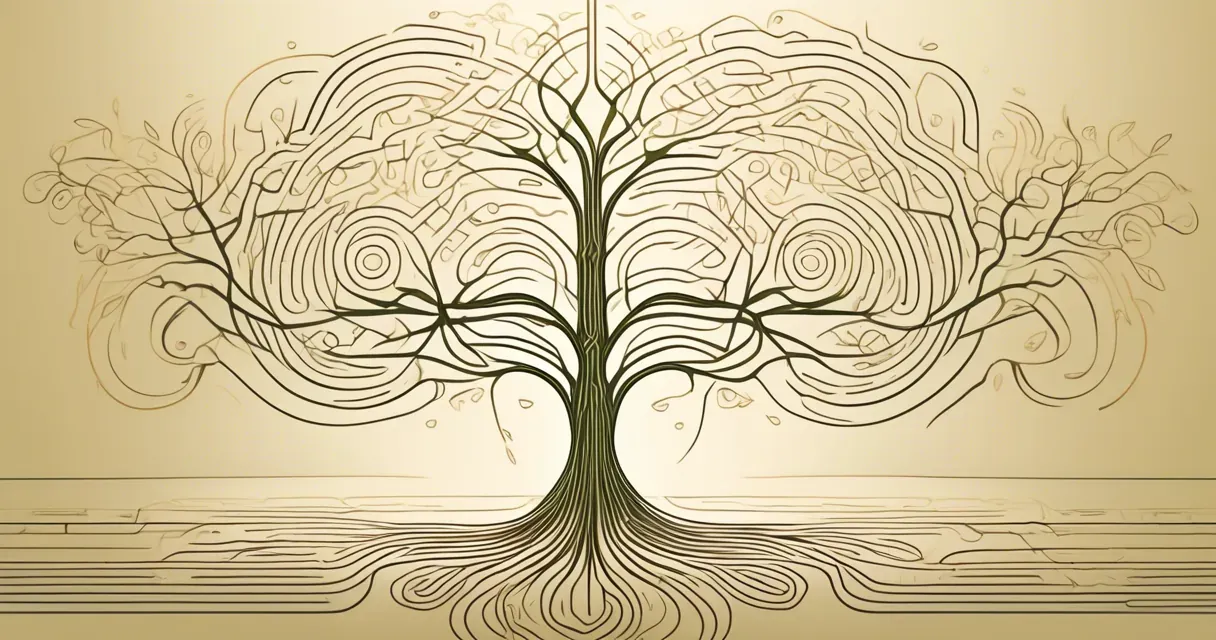
Research Highlights Risks in General-Purpose AI Deployment
/ 4 min read
Quick take - Researchers, led by Mario Fritz, are advancing the understanding and mitigation of vulnerabilities in general-purpose artificial intelligence models through innovative frameworks, including Dynamic Testing and Data-Instruction Separation, to enhance cybersecurity measures and address potential threats.
Fast Facts
- Researchers, led by Mario Fritz, focus on vulnerabilities in general-purpose AI models, aiming to enhance vulnerability awareness and develop mitigation strategies.
- Key findings include the formalization of Data-Instruction Separation to prevent indirect prompt injection attacks and the introduction of a taxonomy for categorizing these threats.
- The study emphasizes the importance of dynamic threat detection and proactive security measures, utilizing AI-driven code security auditing tools.
- Methodologies like Dynamic Testing and Auto Red Teaming are employed to simulate real-world attacks and uncover vulnerabilities in AI systems.
- Future directions include refining Auto Red Teaming mechanisms and exploring advanced multi-agent system security frameworks to adapt to evolving threats.
In the rapidly evolving landscape of cybersecurity, where threats are becoming increasingly sophisticated and pervasive, researchers are incessantly exploring innovative solutions to bolster defenses. A recent study led by Mario Fritz and colleagues sheds light on critical advancements in vulnerability awareness and mitigation strategies, particularly in the context of general-purpose AI models. As organizations increasingly adopt these tools for various applications, understanding the vulnerabilities inherent in their deployment becomes paramount. The study emphasizes enhanced vulnerability awareness, underscoring the urgent need for improved security frameworks that can seamlessly integrate into existing infrastructures.
One of the standout contributions of this research is the introduction of Dynamic Testing and Auto Red Teaming methodologies. By simulating real-world attacks in a controlled environment, these techniques allow organizations to identify potential vulnerabilities before malicious actors can exploit them. This proactive approach not only highlights weaknesses but also aids in developing robust mitigation strategies tailored to specific threats. The study introduces an Indirect Prompt Injection Threat Taxonomy, which categorizes potential attack vectors against AI systems. This taxonomy serves as a vital resource for cybersecurity professionals, helping them understand and anticipate the myriad ways in which attackers may attempt to compromise AI-driven applications.
A significant theme throughout the findings is the necessity of Data-Instruction Separation (DIS) as a foundational requirement for secure AI deployment. By isolating data from instructions, organizations can significantly reduce the risk of exploitation through injection attacks. The formalization of DIS protocols lays out clear guidelines that enhance security measures while ensuring operational efficiency. Such separation not only minimizes vulnerabilities but also promotes a more disciplined approach to coding practices within AI development, fostering a culture of security-first thinking.
In tandem with these strategies, the research advocates for the adoption of AI-Driven Code Security Auditing Tools. These tools leverage machine learning algorithms to analyze codebases for potential vulnerabilities, offering developers insights that manual reviews often miss. While promising, it’s crucial to acknowledge the limitations of these tools; they must be regularly updated to stay relevant in the face of evolving threats. The journey towards achieving comprehensive security measures involves ongoing investigation and refinement of these technologies.
The implications of these findings extend into addressing the broader landscape shaped by an information ecosystem shift driven by advancements in AI. As organizations adapt to this new reality, dynamic threat detection and response systems become vital components in safeguarding digital assets. These systems provide real-time analysis and alerts, enabling swift responses to emerging threats—a necessity given today’s fast-paced cyber environment.
Looking ahead, the research identifies several future directions for enhancing cybersecurity frameworks surrounding general-purpose AI models. Enhanced Data-Instruction Separation Protocols will likely gain traction as organizations strive to fortify their defenses against increasingly complex attack vectors. Additionally, implementing Auto Red Teaming mechanisms can serve as a game-changer in continuous security assessments by automating the penetration testing process, thus allowing teams to focus on remediation rather than discovery.
Moreover, exploring Multi-Agent System Security Frameworks could pave the way for collaborative defense strategies where multiple agents work together to detect and neutralize threats dynamically. These innovations not only reflect a commitment to advancing cybersecurity practices but also highlight an understanding that resilience requires adaptability and foresight.
As we navigate this intricate web of technology and security challenges, integrating these findings into practical applications will be essential for ensuring that AI continues to empower rather than endanger organizations. The future will hinge on our ability to remain one step ahead—anticipating threats while fostering a culture that prioritizes security at every level of development and deployment.