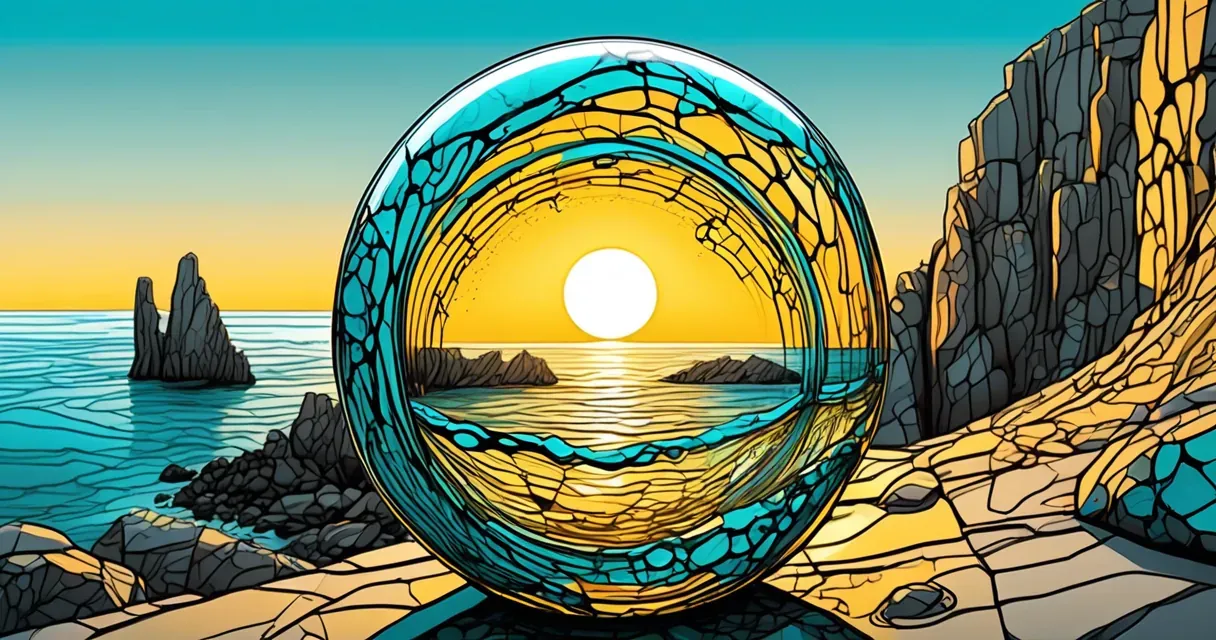
Vulnerabilities of Diffusion Models in AI Explored
/ 4 min read
Quick take - Recent research on the MMP-Attack has revealed significant vulnerabilities in multi-modal AI systems, particularly those using diffusion models for text-to-image generation, highlighting the need for enhanced security measures and robust defense strategies in AI applications.
Fast Facts
-
MMP-Attack Development: A novel adversarial technique, MMP-Attack, was created to exploit vulnerabilities in text-to-image diffusion models, demonstrating superior manipulation capabilities.
-
Adversarial Prompt Detection: The research focused on identifying effective adversarial prompts and optimizing them through advanced gradient-based techniques.
-
Importance of Adversarial Training: The study emphasized adversarial training as a crucial defense mechanism to enhance the robustness of AI models against attacks.
-
Implications for AI Deployment: Findings highlighted the need for secure AI content generation in sensitive applications to prevent exploitation by malicious actors.
-
Future Research Directions: The study calls for increased awareness of multi-modal vulnerabilities, development of robustness metrics, and exploration of effective defense strategies against adversarial attacks.
In the rapidly evolving landscape of artificial intelligence, the intersection of cybersecurity and multi-modal AI systems presents both immense potential and significant challenges. The emergence of diffusion models—sophisticated frameworks that generate images from textual descriptions—has revolutionized creative processes, yet simultaneously raised concerns about their vulnerabilities to adversarial attacks. As researchers delve into the intricacies of these models, the concept of MMP-Attack has come to the forefront, designed to exploit weaknesses in text-to-image generation by manipulating inputs to achieve desired visual outputs. This raises crucial questions about how we can safeguard against such threats while harnessing the capabilities of these advanced technologies.
The foundational goal of recent studies is to better understand the cross-model transferability of attacks, revealing that adversarial prompts can affect various diffusion models differently. By employing techniques like t-SNE visualization, researchers are able to map and visualize multi-modal feature spaces, offering insights into how models process information and where they are susceptible. Such visualizations not only enhance our understanding but also serve as a critical tool for identifying vulnerabilities. The systematic approach taken in this research involves developing robust metrics for evaluating model performance against adversarial inputs and establishing a framework for effective adversarial training aimed at bolstering model robustness.
Among the strengths of this research is its focus on ethical and responsible AI use, advocating for a balance between innovation and security. The findings highlight the need for secure AI content generation practices, especially in sensitive applications where manipulation could have dire consequences. By emphasizing ethical considerations alongside technological advancements, researchers aim to ensure that multi-modal systems serve their intended purposes without falling prey to nefarious exploits.
In exploring the capabilities of CLIP (Contrastive Language-Image Pretraining) models in conjunction with diffusion frameworks, the research underscores a pivotal shift towards understanding how language and imagery interact within AI systems. This interaction is crucial for developing more resilient models capable of withstanding adversarial assaults like those posed by MMP-Attack. The study evaluates the effectiveness of this attack against existing defenses, demonstrating its superior manipulation capability through comprehensive experiments across various text-to-image models.
Despite its promising findings, the research does acknowledge limitations—particularly in generalizability across all types of diffusion models and the need for further investigation into multi-modal threats. As artificial intelligence continues to permeate various sectors, understanding these limitations becomes vital for developing effective defense mechanisms. Future directions may include increased awareness of multi-modal vulnerabilities, fostering collaborative efforts in multi-modal threat intelligence, and enhancing methodologies that support robust AI development.
The implications of deploying these advanced models in sensitive contexts cannot be overstated; as organizations increasingly rely on AI-generated content, ensuring their integrity becomes paramount. The roadmap paved by current research guides future inquiries into enhancing security measures while also considering the ethical ramifications of AI deployment.
Looking ahead, as technology advances and becomes more integrated into daily life, it will be essential for researchers and practitioners alike to remain vigilant about potential exploits in AI systems. By focusing on robust defense mechanisms and responsible AI usage, stakeholders can not only mitigate risks but also ensure that innovations continue to drive progress rather than compromise it. The journey towards secure multi-modal AI systems is just beginning, but with continued collaboration and rigorous investigation, we can forge a path that embraces innovation while safeguarding against emerging threats.