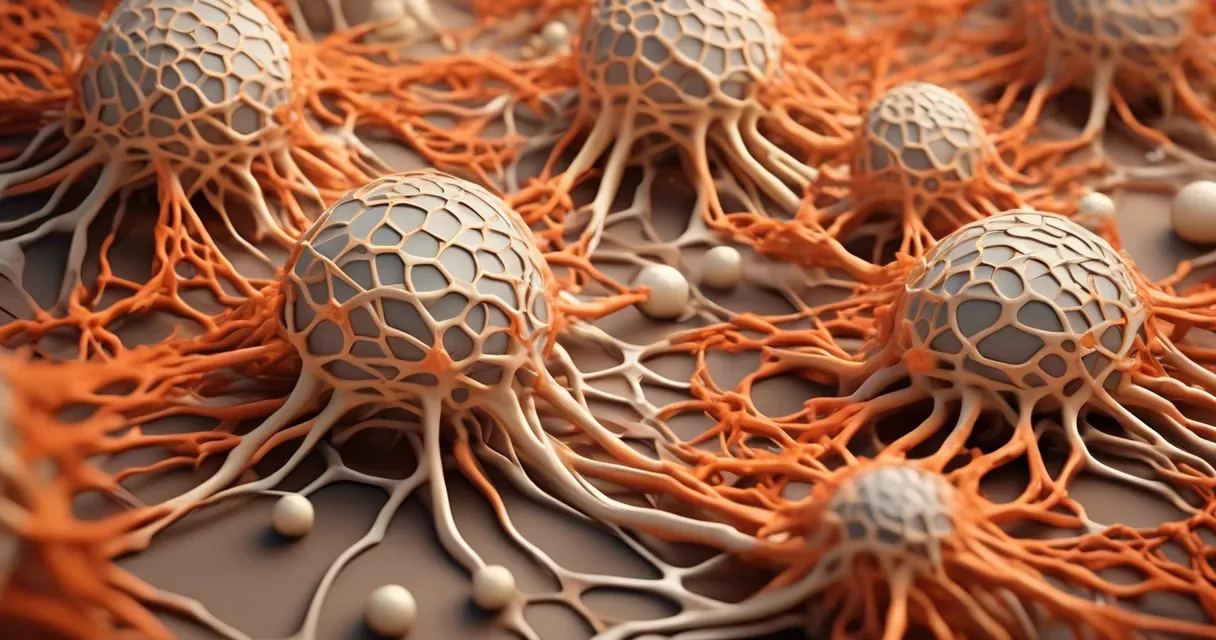
GraphDART Framework Enhances Threat Detection in Cybersecurity
/ 4 min read
Quick take - Researchers have developed the GraphDART framework, which combines graph learning techniques with real-time intrusion detection systems to enhance anomaly detection in cyber-physical-social systems, addressing current cybersecurity challenges and paving the way for future advancements in the field.
Fast Facts
- GraphDART Framework: A new framework combining graph learning and real-time intrusion detection systems to enhance anomaly detection in cyber-physical-social systems (CPSSs).
- Improved Anomaly Detection: Utilization of Graph Neural Networks (GNNs) and Graph Distillation Method (GCDM) led to better precision and recall rates in identifying advanced persistent threats (APTs).
- Scalability and Efficiency: The framework allows organizations to adapt their cybersecurity measures as they grow, addressing the dynamic nature of modern threats.
- Data Privacy Focus: Emphasizes the importance of balancing robust security with data privacy, highlighting ethical considerations in AI applications.
- Future Research Directions: Proposes further exploration of graph learning applications, automated incident response systems, and cross-domain security applications, particularly in IoT and smart cities.
In an era where cyber threats loom larger than ever, the quest for robust cybersecurity solutions has become imperative. The intricate dance between technological advancement and the rising sophistication of cyber adversaries necessitates a comprehensive approach that not only involves cutting-edge tools but also innovative methodologies. Recent research highlights several promising avenues in this domain, particularly focusing on anomaly detection techniques, graph learning for threat intelligence, and the integration of advanced frameworks like GraphDART to enhance real-time intrusion detection systems (IDS).
Anomaly detection is at the forefront of these advancements, especially within Internet of Things (IoT) networks. As more devices connect to the internet, they create a complex landscape where identifying irregular patterns becomes crucial for maintaining security. Research emphasizes improving accuracy and efficiency in detecting these anomalies, which can significantly reduce false positives that often plague traditional IDS. In this context, Graph Neural Networks (GNNs) have emerged as a powerful tool, allowing for nuanced analysis of interconnected data points. By leveraging GNNs, cybersecurity professionals can achieve an enhanced understanding of user behaviors and network dynamics.
Equally important is the role of graph-based threat intelligence sharing platforms. These systems enable organizations to share insights on emerging threats in real time, fostering a collaborative environment that enhances collective defense strategies. The challenge lies in ensuring data privacy while facilitating this exchange. Research has indicated that balancing transparency with confidentiality is crucial for maintaining trust among entities sharing sensitive information.
The Distribution Matching Method (GCDM) further complements these efforts by refining how threat data is interpreted and utilized across various platforms. It allows for better alignment of threat intelligence with actual attack vectors, ensuring that organizations can prioritize their defenses effectively. This methodology underscores the importance of adaptability in cybersecurity strategies—highlighting that static responses are insufficient against dynamic threats.
As research progresses, there is a growing emphasis on scalability in cybersecurity solutions. The ability to expand systems without sacrificing performance or security integrity is essential given the rapid growth of digital infrastructures. Future investigations into core-set selection methods provide pathways for optimizing resource allocation while maintaining high recall rates in threat detection frameworks. Such advancements will allow organizations to respond swiftly to evolving threats without overwhelming their security systems.
Looking ahead, the implications of these findings are profound. The intersection of anomaly detection with advanced graph learning techniques promises a future where cybersecurity measures are not only reactive but also predictive in nature. Automated incident response mechanisms could dramatically shorten breach response times, thereby minimizing potential damage. Additionally, as interdisciplinary approaches continue to flourish—drawing from artificial intelligence and data privacy considerations—the robustness of cybersecurity frameworks will likely improve exponentially.
In conclusion, as we navigate through increasingly complex cyber landscapes, the innovations stemming from recent research mark significant strides toward fortified cybersecurity postures. With ongoing exploration into graph-based methodologies and enhanced machine learning techniques, the future holds promise for creating resilient systems capable of thwarting even the most sophisticated cyber threats. As organizations embrace these tools and principles, they will not only safeguard their assets but also contribute to a broader movement toward secure digital ecosystems globally.