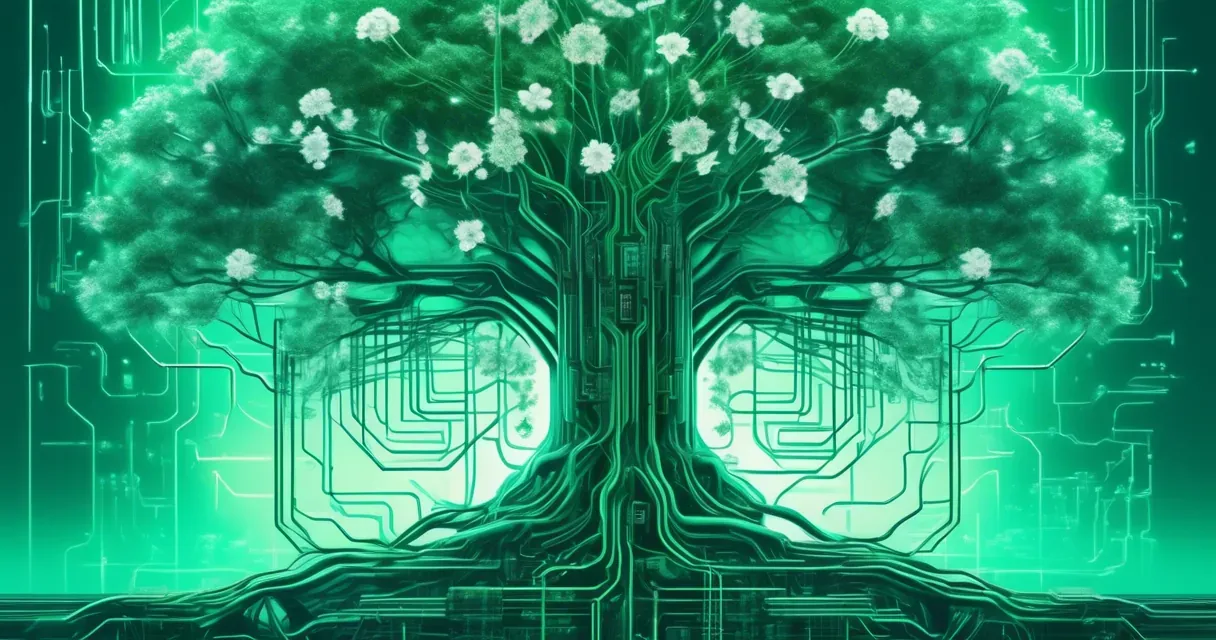
Innovative AI Solutions for Cybersecurity in CPS
/ 4 min read
Quick take - Recent research on enhancing cybersecurity in cyber-physical systems focuses on advanced methodologies and adaptive strategies to improve resilience against cyber threats, emphasizing the importance of anomaly detection, data privacy, and risk management.
Fast Facts
- Recent research focuses on enhancing cybersecurity in cyber-physical systems (CPS) through advanced methodologies and adaptive strategies to improve detection, data privacy, and risk management.
- Key findings include the effectiveness of adaptive cybersecurity frameworks for Industrial IoT, emphasizing context-aware security solutions and batch federated aggregation methods for privacy and collaborative learning.
- Innovative tools discussed include Kitsune for real-time anomaly detection, CANSHEILD for autonomous vehicle security, and bio-inspired deep neural networks for improved anomaly detection.
- The research highlights the need for further investigation into optimizing anomaly detection, refining risk assessment methods, and exploring cross-domain attack detection strategies.
- Future directions emphasize developing adaptive security mechanisms and fostering collaboration between cybersecurity and physical security to create a comprehensive defense strategy for CPS.
In an era where our physical and digital worlds are increasingly intertwined, the security of cyber-physical systems (CPS) has never been more crucial. As cities evolve into smart metropolises and industries adopt advanced technologies, vulnerabilities emerge that hackers eagerly exploit. To combat these threats, researchers are turning to innovative methodologies that leverage explainable artificial intelligence (AI) for effective cybersecurity decision-making. This approach focuses on enhancing detection capabilities, safeguarding data privacy, and refining risk management in CPS.
Adaptive learning algorithms stand at the forefront of this evolution. These algorithms allow systems to self-improve over time by learning from new data patterns, thus enhancing their ability to detect anomalies—an essential feature for maintaining security in environments like smart manufacturing and autonomous vehicles. For instance, the integration of federated learning in smart cities exemplifies how collaborative AI can be utilized to train models across decentralized networks without compromising sensitive data. By allowing individual devices to learn locally while contributing to a global model, federated learning not only bolsters anomaly detection but also preserves privacy—a critical aspect when dealing with personal or proprietary information.
The research highlights several key tools and frameworks that play pivotal roles in enhancing CPS security. Kitsune, an anomaly detection framework, utilizes machine learning techniques to identify unusual patterns indicative of potential breaches. Meanwhile, Batch Federated Aggregation serves as a vital method for improving the robustness of federated learning models by aggregating insights from multiple participants, further reinforcing defenses against cyber threats. Together, these technologies lay the groundwork for creating adaptive cybersecurity frameworks tailored specifically for industrial IoT environments.
As we delve deeper into these advancements, it becomes evident that context-aware security solutions are paramount. These solutions dynamically assess security needs based on the context in which they operate, ensuring that protective measures are both relevant and effective. The development of hybrid approaches—combining traditional methods with cutting-edge machine learning techniques—enables organizations to better predict risks and manage vulnerabilities across their operations.
While significant progress has been made, challenges remain. The research identifies areas requiring further investigation, particularly regarding risk assessment and criticality analysis within CPS. Understanding asset criticality is essential for prioritizing protection measures effectively. Moreover, researchers emphasize the need for enhanced anomaly detection techniques powered by deep learning to counteract sophisticated cyber attacks that target complex systems.
Collaboration between cybersecurity and physical security sectors cannot be overlooked. As we witness an increasing convergence of these domains, strategies must evolve to ensure comprehensive protection against potential threats. The integration of machine learning for real-time anomaly detection serves as a promising solution, harnessing advanced algorithms to provide timely alerts against breaches before they escalate into crises.
Looking ahead, the findings underscore a future where the resilience of cyber-physical systems hinges on leveraging advanced technologies alongside adaptive strategies. As interconnected devices proliferate and cyber threats evolve in complexity, embracing a proactive stance on risk management will be essential for safeguarding not just critical infrastructure but also everyday life in our increasingly digital world. By addressing existing limitations and fostering innovation in cybersecurity methodologies, we can better prepare ourselves for the challenges that lie ahead in this dynamic landscape.
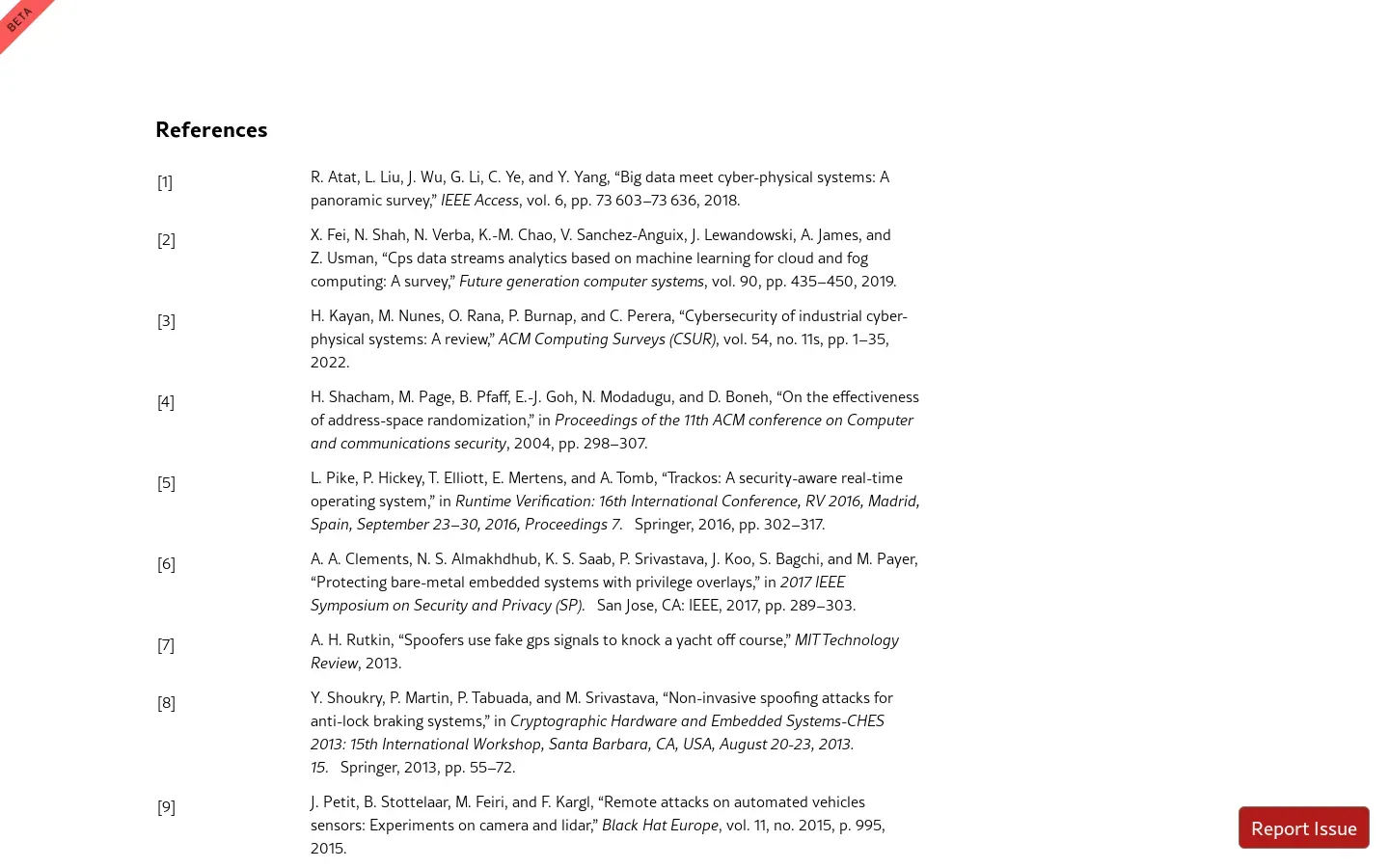