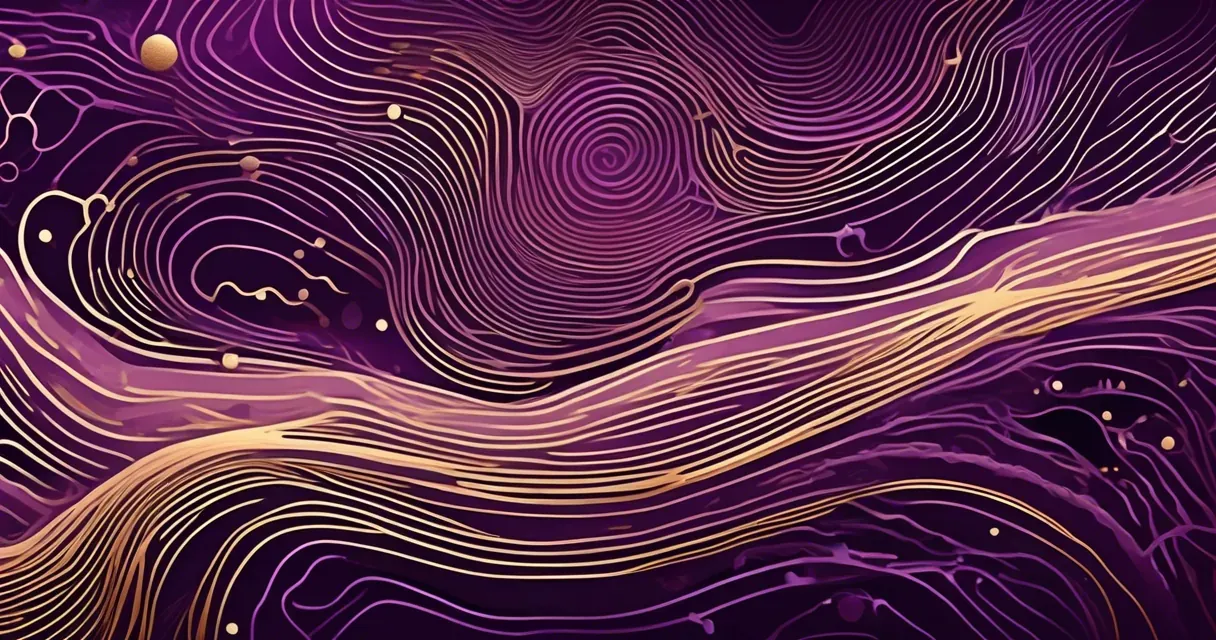
SecBench: New Benchmarking Dataset for Cybersecurity LLMs
/ 4 min read
Quick take - Recent research in cybersecurity highlights advancements in cross-language knowledge sharing, benchmarking of emerging technologies, and the development of specialized training programs, aiming to enhance global cooperation and improve the effectiveness of cybersecurity measures.
Fast Facts
-
Cross-Language Knowledge Sharing: Research highlights the importance of standardized terminologies and collaborative platforms to overcome language barriers in cybersecurity knowledge transfer among international experts.
-
Benchmarking Innovations: Introduction of SecBench, a specialized dataset for evaluating Large Language Models (LLMs) in cybersecurity, enabling realistic assessments of threat detection capabilities.
-
Automatic Evaluation Tools: Development of a natural language processing tool for automatically assessing short-answer questions (SAQs) to enhance cybersecurity training and provide immediate feedback to learners.
-
Specialized Training Programs: Emphasis on creating tailored cybersecurity training programs that address sector-specific challenges and incorporate real-world case studies for effective skill development.
-
Future Directions: Recommendations for expanding datasets, improving evaluation tools, and fostering international collaboration to enhance knowledge sharing and adapt to evolving cyber threats.
In the ever-evolving landscape of cybersecurity, where threats grow increasingly sophisticated and ubiquitous, the need for effective knowledge sharing across language barriers becomes paramount. Cross-language cybersecurity knowledge sharing stands at the forefront of this challenge, aiming to enhance global collaboration among cybersecurity professionals. Research in this domain is not just a theoretical exercise; it’s a practical approach to bridging gaps that can leave organizations vulnerable. By investigating methodologies that facilitate seamless communication and understanding of cybersecurity concepts across different languages, we open doors to a more unified defense against cyber threats.
The methodology employed in these studies often involves extensive analysis of existing knowledge-sharing frameworks, alongside innovative approaches that leverage advancements in technology. For instance, benchmarking and evaluating emerging cybersecurity technologies have become essential practices. Researchers focus on creating benchmarks that allow organizations to assess their readiness against current and anticipated threats. This includes evaluating tools designed for automated cybersecurity threat detection and response, which utilize machine learning algorithms to identify anomalies in network traffic or user behavior. Such tools are critical in preemptively thwarting attacks before they escalate into significant breaches.
One notable development within this research framework is the automatic evaluation of short-answer questions (SAQs), which serves as a tool for measuring knowledge retention among cybersecurity professionals. The strength of this method lies in its ability to provide immediate feedback and gauge understanding effectively, thereby ensuring that training programs are not just theoretical but grounded in practical application. Nonetheless, limitations exist; the effectiveness of SAQ evaluations can vary greatly depending on the complexity of the questions posed and the individual’s prior knowledge.
Meanwhile, data labeling plays a crucial role in enhancing the quality of datasets used for training models in cybersecurity contexts. The construction of robust datasets is fundamental for developing specialized cybersecurity training programs tailored to address specific gaps within organizations. These programs can significantly improve knowledge retention and operational readiness among staff, thus contributing to a more resilient cybersecurity posture.
At the heart of this research is SecBench, a benchmarking dataset specifically designed for assessing Large Language Models (LLMs) within cybersecurity realms. Its significance cannot be overstated; by providing standardized benchmarks, SecBench facilitates a structured evaluation process that addresses existing shortcomings in current methodologies. It empowers institutions to make informed decisions about integrating AI-driven solutions into their security frameworks while also highlighting potential areas for improvement.
Yet, as with any emerging technology, there are inherent limitations. The reliance on automated systems raises concerns regarding false positives and negatives—issues that can lead to misallocation of resources or missed threats. Furthermore, as LLMs continue to evolve, so too must our benchmarks; staying ahead requires continuous adaptation and refinement.
Looking ahead, the future of cybersecurity will undoubtedly hinge on our ability to foster collaboration across linguistic divides while simultaneously leveraging cutting-edge technologies like LLMs and advanced data analytics. As researchers and practitioners delve deeper into the interconnected realms of technology and human knowledge, we may find ourselves better equipped to counteract the multifaceted nature of cyber threats. The journey towards achieving comprehensive cybersecurity resilience is ongoing, yet with each breakthrough in knowledge sharing and technological evaluation, we inch closer to safeguarding our digital landscapes against an unpredictable future.
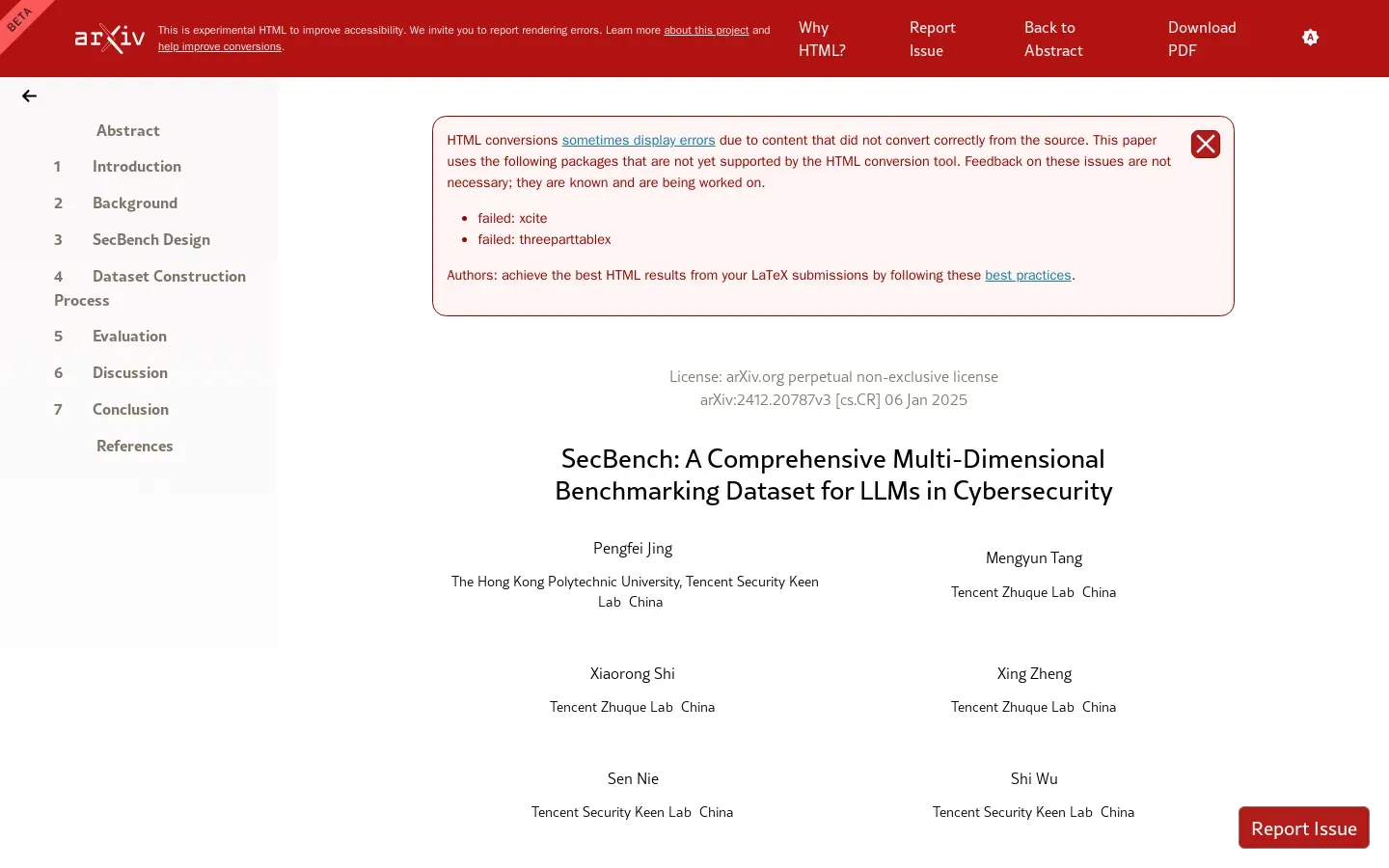