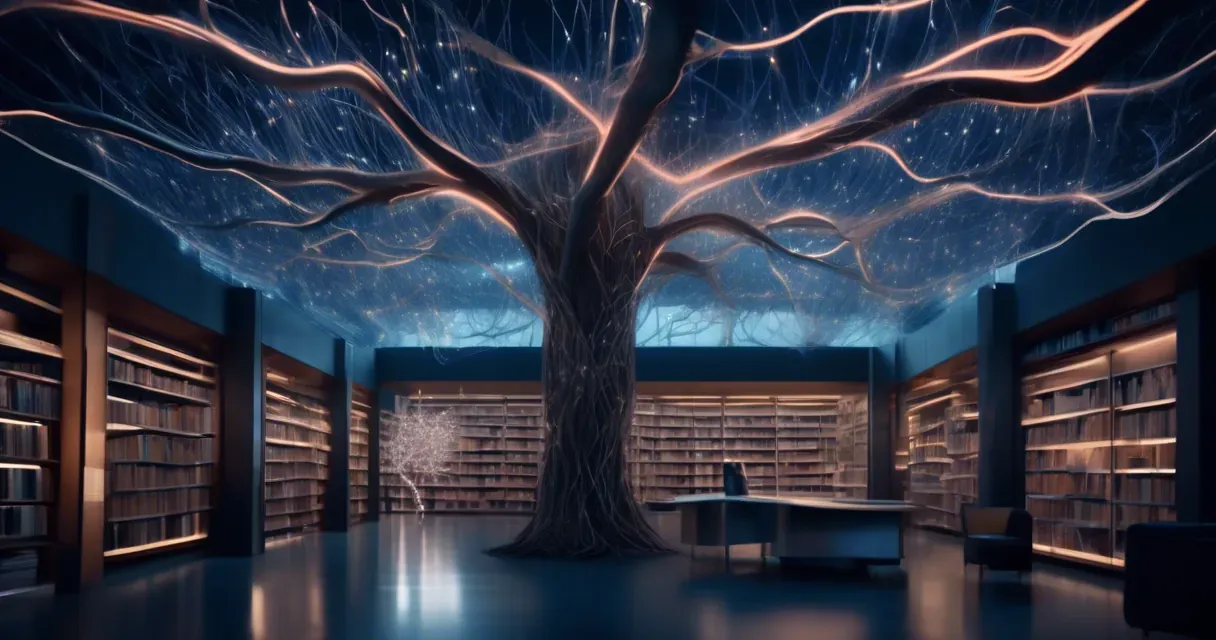
Strategies to Enhance Security of Large Language Models
/ 4 min read
Quick take - Recent research has focused on developing adaptive defense mechanisms to enhance the safety and robustness of large language models against adversarial attacks, particularly jailbreak attempts, while maintaining their performance on benign tasks.
Fast Facts
- Research focuses on enhancing the safety and robustness of large language models (LLMs) against adversarial prompts, particularly jailbreak attacks.
- Adaptive defense mechanisms were developed using Layer-specific Editing (LED) and Reinforcement Learning from Human Feedback (RLHF) to improve model security while maintaining performance.
- Key findings indicate that LED effectively mitigates jailbreak attacks and that cross-model defense strategies can enhance overall security.
- The study emphasizes the importance of user education and awareness in understanding and mitigating risks associated with LLMs.
- Future research should refine adaptive mechanisms and promote interdisciplinary collaboration among cybersecurity experts, AI researchers, and policymakers for improved LLM security.
In an era where artificial intelligence is rapidly reshaping industries and everyday life, the security of large language models (LLMs) becomes paramount. The proliferation of these models has ushered in both advancements and vulnerabilities, particularly concerning adversarial prompts that can manipulate their outputs. As we delve into this pressing issue, recent research shines a light on innovative strategies aimed at fortifying LLMs against such threats. This multifaceted approach not only aims to bolster defenses but also seeks to retain the efficacy of these models in benign tasks—a delicate balance that underpins the ongoing evolution of cybersecurity practices.
Layer-specific Editing (LED) emerges as a pivotal technique in this landscape, enabling targeted modifications within specific layers of LLMs to mitigate risks associated with jailbreak attacks. This method allows for nuanced alterations without compromising the overall functionality of the model. By strategically adjusting responses at various levels, researchers can enhance the robustness of LLMs while preserving their performance across standard applications. This approach demonstrates a keen understanding that effective cybersecurity measures must integrate seamlessly with operational capabilities.
Another significant advancement is Reinforcement Learning from Human Feedback (RLHF), which harnesses real-time human input to fine-tune model responses. This feedback loop not only improves accuracy but also enhances the model’s ability to discern between constructive and harmful prompts. The implications are clear: by prioritizing human oversight in the learning process, LLMs become increasingly resilient against manipulative tactics employed by malicious actors.
A vital component of this research is the development of adaptive defense mechanisms designed to evolve alongside emerging threats. These mechanisms adjust dynamically based on threat intelligence, ensuring that LLMs remain fortified against novel forms of attack. This adaptability is crucial in a landscape where cyber threats are perpetually evolving; static defenses can quickly become outdated. Coupled with integration into multi-layered security frameworks, these adaptive solutions promise a more holistic defense strategy.
The role of adversarial prompt generation cannot be overlooked. Understanding how adversaries formulate their attacks equips researchers and practitioners with the tools necessary to preemptively guard against them. By simulating potential attack vectors, teams can refine their defensive strategies and better prepare LLMs for real-world scenarios where manipulation is a constant risk.
Moreover, user education and awareness programs play an indispensable role in this ecosystem. Equipping users with knowledge about potential vulnerabilities fosters a culture of vigilance that extends beyond technical defenses. By promoting awareness around cybersecurity best practices, organizations can empower individuals to become active participants in safeguarding LLMs.
In reflecting on these findings, it becomes evident that the implications extend far beyond mere technical defenses. The interplay between cybersecurity practices, model design, and interdisciplinary collaboration highlights a critical need for cohesive efforts across various domains. As researchers explore these avenues further, they pave the way towards not just enhancing security but ensuring the responsible use of LLMs across diverse applications.
While there are limitations inherent in each strategy—such as the potential for overfitting during layer-specific edits or challenges inherent in obtaining consistent human feedback—the collective potential of these approaches offers a promising outlook for future developments. Looking ahead, as we continue to confront an increasingly complex cyber threat landscape, the integration of robust defense mechanisms into LLM architecture will be essential for fostering trust and reliability in AI-driven solutions. The journey toward secure LLMs has only just begun, but with concerted efforts across disciplines, there lies immense potential for creating safer digital environments that leverage the power of artificial intelligence responsibly.