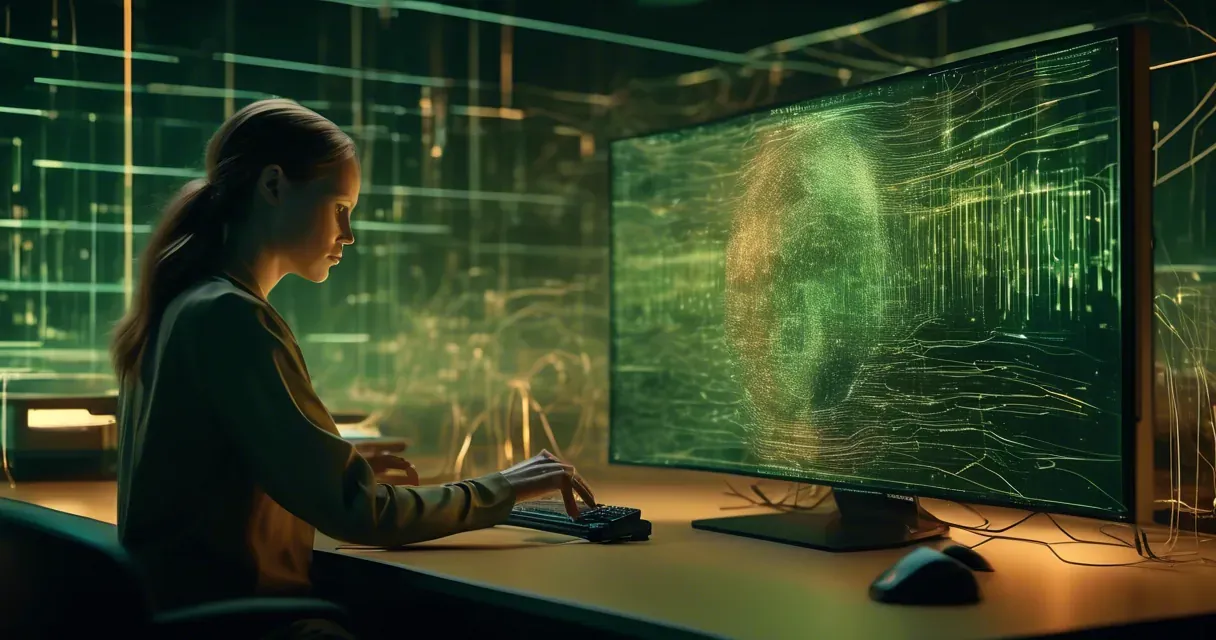
Study Examines Machine Learning for Malware Detection on Windows
/ 4 min read
Quick take - A study by Marzieh Esnaashari and Nima Moradi explores the effectiveness of advanced machine learning techniques, particularly XGBoost, in enhancing malware detection through robust model development and feature engineering, while emphasizing the need for adaptive learning systems to address evolving cyber threats.
Fast Facts
- Researchers Marzieh Esnaashari and Nima Moradi explored advanced machine learning techniques to enhance malware detection, focusing on feature engineering and innovative methodologies.
- The study integrated machine learning algorithms with threat intelligence platforms, utilizing methods like Support Vector Machines, Random Forests, and XGBoost for effective malware identification.
- Key findings indicated that gradient boosting methods, especially XGBoost, outperformed others in malware detection, highlighting the importance of model tuning and feature selection.
- The research advocates for adaptive learning systems and real-time detection to improve organizational defenses against evolving cyber threats.
- Future directions include exploring deep learning techniques, addressing dataset imbalance, and assessing model adaptability across different operating systems.
In the ever-evolving landscape of cybersecurity, the fight against malware has taken on new dimensions, fueled by advancements in machine learning and data analysis. As cyber threats grow in sophistication, traditional detection methods often fall short, necessitating innovative approaches. Recent research conducted by Marzieh Esnaashari and Nima Moradi sheds light on the pivotal role that feature engineering and advanced machine learning algorithms play in enhancing malware detection capabilities. This study provides a comprehensive view of the methodologies employed to develop robust detection models while also highlighting areas for future exploration.
At the heart of this research is a meticulous focus on feature engineering—an essential process that involves identifying and analyzing key indicators that signal malware infections. By applying innovative feature analysis techniques, the researchers aim to refine detection methodologies, ultimately leading to improved accuracy and efficiency in identifying malicious software. This is particularly crucial given the varied nature of malware, which can manifest differently across platforms and environments. The study’s findings underscore the necessity of integrating insights from threat intelligence platforms, allowing for a more proactive stance against emerging threats.
The exploration of various machine learning algorithms, including Support Vector Machines (SVM), Random Forests, and XGBoost (eXtreme Gradient Boosting), reveals their effectiveness in tackling malware challenges. Notably, gradient boosting methods have shown exceptional performance, emphasizing the significance of careful model tuning and judicious feature selection. This aligns with the broader trend in cybersecurity where organizations strive to balance accuracy with computational efficiency—a critical factor when deploying real-time malware detection systems.
An intriguing aspect of this research is its emphasis on cross-platform malware detection. As devices become increasingly interconnected, the ability to detect malware across different operating systems and environments is paramount. The study advocates for further investigation into how these models can be adapted to various enterprise settings, thereby enhancing their generalizability and effectiveness. This adaptability is not just about recognizing known threats but also about developing systems capable of identifying novel or previously unseen malware variants.
While the findings are promising, they also highlight limitations that warrant attention. For instance, there remains a need for comparative studies between traditional machine learning approaches and emerging deep learning techniques. Such investigations could unveil new insights into performance metrics, especially regarding the adaptability of detection systems to evolving cyber threats. Furthermore, addressing dataset imbalances through synthetic data generation or advanced sampling techniques could bolster the robustness of these models.
Looking ahead, it becomes clear that real-time malware detection systems must evolve alongside cybercriminal tactics. Implementing these advanced machine learning models in production environments will be crucial for assessing their performance under practical conditions—focusing on latency, accuracy, and adaptability. As organizations increasingly rely on automated solutions for cybersecurity, understanding user behavior through analytics may offer an additional layer of defense against sophisticated attacks.
In conclusion, as we navigate the complexities of modern cybersecurity landscapes, continuous improvement in malware detection methodologies will be vital. The integration of advanced machine learning techniques promises to enhance our defensive capabilities significantly. Yet, it is imperative that researchers and practitioners remain vigilant, adapting strategies as new threats emerge and technologies evolve—ensuring that our defenses are always one step ahead in this ongoing digital battleground.