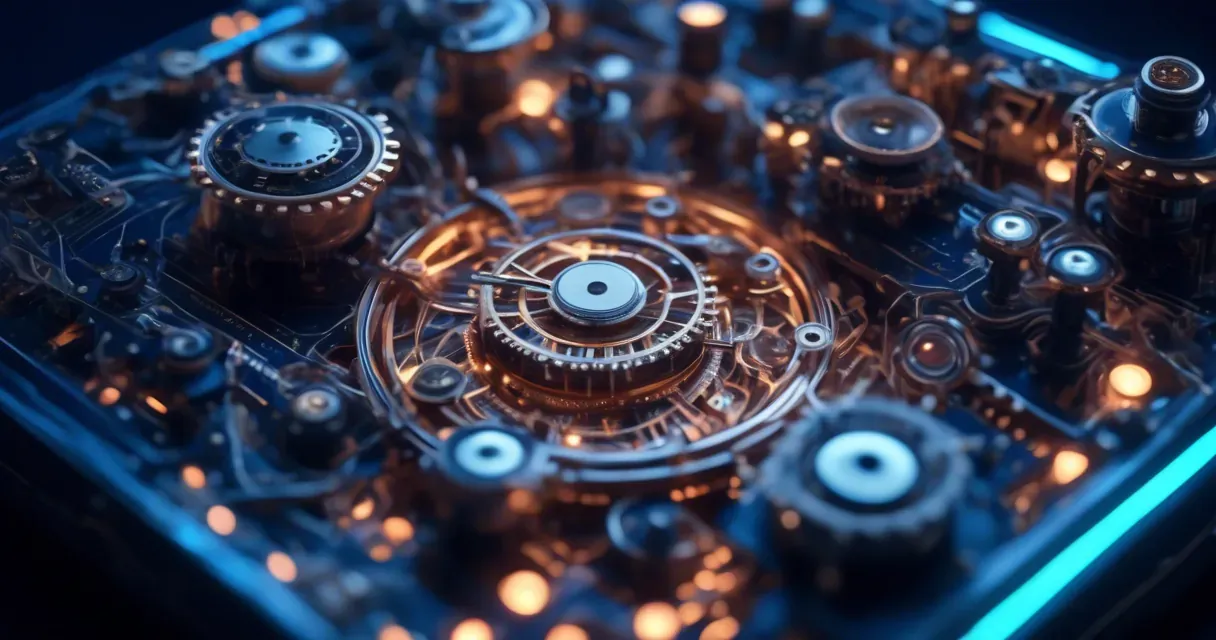
Study Introduces Method for Predicting IoT Vulnerability Fix Times
/ 3 min read
Quick take - Recent research has developed a predictive framework for estimating the time required to fix vulnerabilities in Internet of Things (IoT) systems, utilizing advanced statistical models to enhance cybersecurity and risk management strategies.
Fast Facts
- New research introduces a framework for predicting vulnerability fix times in IoT systems, addressing critical cybersecurity challenges.
- The study utilized the Accelerated Failure Time (AFT) Model and XGBoost Ensemble Regression Model for data analysis, emphasizing data quality and feature engineering.
- Findings indicate that the XGBoost model significantly outperforms traditional methods, enhancing predictive accuracy for vulnerability resolution.
- The research promotes improved patch management strategies and data-driven security policies, aiding organizations in risk assessment and incident response.
- Future research directions include refining predictive models through enhanced feature engineering and exploring cross-domain vulnerability predictions.
In an era increasingly dominated by the Internet of Things (IoT), the ramifications of unpatched vulnerabilities could be catastrophic. As billions of devices connect to the internet, the potential attack surface expands exponentially, making the security of these systems paramount. A recent study has shed light on a groundbreaking approach to predicting how long it will take to fix vulnerabilities within IoT systems. By emphasizing the importance of data quality and model selection, this research introduces methodologies that could significantly enhance cybersecurity strategies.
At the heart of the research is the use of an XGBoost ensemble regression model, a powerful tool for predicting outcomes based on complex datasets. This model excels in feature selection and evaluation techniques, enabling researchers to sift through extensive data on IoT vulnerabilities effectively. The integration of an Accelerated Failure Time (AFT) model further refines predictions, providing insights into not just whether a vulnerability will be patched, but when. This dual approach allows cybersecurity professionals to prioritize vulnerabilities based on their likelihood and timing of resolution, an essential factor in managing risk.
The study also proposes enhanced patch management strategies that can lead to more informed decision-making during IoT deployments. With comprehensive databases detailing IoT vulnerabilities, decision-makers can leverage real-time threat intelligence platforms to monitor vulnerabilities actively. Such proactive measures are crucial as they enable organizations to respond swiftly, rather than reactively, mitigating potential damage before it occurs.
Furthermore, the research emphasizes vendor accountability and compliance monitoring, which are vital in ensuring that manufacturers adhere to security standards. By implementing robust frameworks that hold vendors responsible for timely patches, organizations can create a more secure ecosystem. In conjunction with advanced risk assessment models, companies are better equipped to navigate the complexities of vulnerability management.
The implications extend beyond immediate fixes; with data-driven security policies grounded in reliable predictions, businesses can redesign their incident response planning. Enhanced feature engineering and data enrichment techniques allow for a nuanced understanding of vulnerabilities, equipping teams with the knowledge needed to tackle threats efficiently.
While the findings are promising, limitations exist. The reliance on a comprehensive IoT vulnerabilities database raises concerns about data accuracy and completeness—an area ripe for further investigation. Additionally, integration with automated patch management systems remains a challenge that needs addressing to maximize efficacy.
As we look ahead, the pathways outlined by this research open doors to numerous applications in cybersecurity. Future investigations could refine these predictive models even further, potentially incorporating machine learning advancements that adapt to emerging threats in real-time. The landscape of IoT security is evolving rapidly; thus, ongoing innovation in vulnerability prediction methodologies will be crucial in staying one step ahead of cybercriminals. Ultimately, as these predictive frameworks gain traction, they herald a future where organizations can not only anticipate but also preemptively address vulnerabilities before they become significant threats.