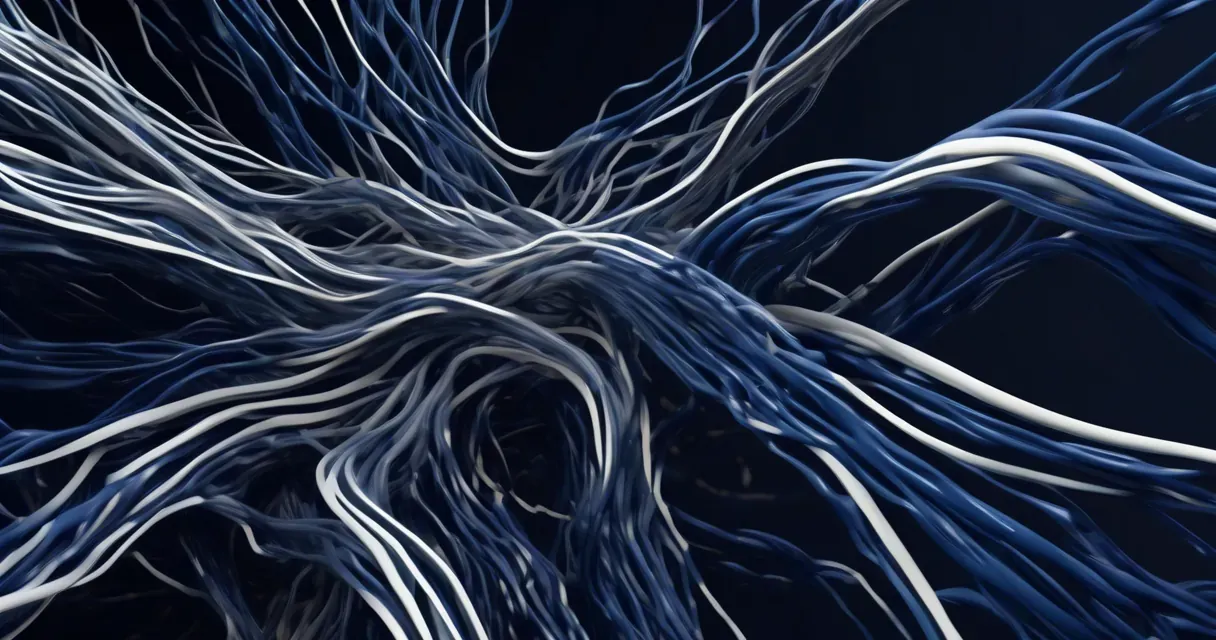
Advancements in Encrypted Traffic Classification with MH-Net
/ 4 min read
Quick take - The study introduces MH-Net, a novel model designed to improve the classification of encrypted network traffic, enhancing the detection of malicious activities and paving the way for future innovations in real-time traffic monitoring and anomaly detection in cybersecurity.
Fast Facts
-
Introduction of MH-Net: A novel model designed to enhance the classification of encrypted network traffic, addressing challenges in cybersecurity and enabling real-time monitoring and anomaly detection.
-
Advanced Classification Techniques: Utilizes multi-task training and heterogeneous traffic graph representation for improved flow-level and packet-level traffic classification through byte correlation analysis.
-
Key Findings: Demonstrated enhanced detection of malicious activities, reduced false positives, and robust performance through experimental validation and contrastive learning integration.
-
Supporting Tools and Frameworks: Incorporates tools like EBSNN for byte analysis, Securitas for real-time monitoring, and adaptive learning systems for continuous improvement.
-
Future Implications: Promises advancements in anomaly detection and IoT security frameworks, with potential for integration into existing cybersecurity tools for a comprehensive defense strategy.
In the ever-evolving landscape of cybersecurity, where threats grow increasingly sophisticated and adaptive, the need for advanced traffic classification techniques has never been more pressing. Traditional methods often struggle to keep pace with the demands posed by encrypted traffic, leaving organizations vulnerable to undetected malicious activities. Enter MH-Net, a cutting-edge model designed to revolutionize how we classify and monitor network traffic. By leveraging innovative methodologies such as byte correlation analysis and multi-task training, MH-Net stands at the forefront of enhancing anomaly detection capabilities and reducing false positives in real-time traffic monitoring.
The research behind MH-Net highlights its unique strengths. Contrastive learning integration plays a pivotal role, allowing the model to differentiate between benign and malicious activities with greater precision. This capability is particularly crucial in an era where attackers frequently employ encryption to hide their malicious intents. Additionally, the model’s architecture incorporates a multi-view traffic graph construction, which not only captures complex relationships between different traffic flows but also adapts to heterogeneous data sources—a necessity for modern networks that encompass a variety of devices and protocols.
While the findings are promising, they also reveal limitations that warrant further exploration. For instance, while MH-Net excels at classifying encrypted traffic, the integration with threat intelligence platforms could amplify its effectiveness by providing context-rich insights into emerging threats. Moreover, there exists a compelling opportunity to expand its application within IoT security frameworks, given the unique challenges presented by interconnected devices that often operate under constrained resources.
Another area ripe for investigation is the potential development of enhanced anomaly detection systems built on the foundation of MH-Net. The ability to detect deviations from established patterns is paramount in preempting cyber attacks before they escalate. The research suggests that future iterations could incorporate elements from Extended Byte Segment Neural Networks (EBSNN), which would further refine detection accuracy and adaptability to evolving threats.
As organizations increasingly turn to cloud services and remote work solutions, the need for robust VPN and encrypted traffic analysis becomes evident. The research advocates for a framework that can seamlessly integrate these analyses into existing infrastructure without sacrificing performance or user privacy. This balance is crucial; effective solutions must ensure that user data remains protected while still enabling organizations to monitor for malicious behavior.
Another significant implication of this research lies in its potential impact on privacy-preserving solutions. As data breaches become more prevalent, users demand transparency and security in their online interactions. By developing models like MH-Net that prioritize both classification accuracy and user privacy, cybersecurity practitioners can build trust with consumers while reinforcing defenses against cyber threats.
The experimental validation of MH-Net demonstrates its practical applicability across various cybersecurity scenarios. With a focus on real-time monitoring and enhanced detection of malicious activities, it offers a viable pathway toward securing networks against increasingly sophisticated attacks. Yet, as promising as these advancements are, they beckon further inquiry into their long-term resilience against emerging attack vectors.
Looking forward, the journey toward comprehensive cybersecurity solutions is far from over. As researchers continue to refine models like MH-Net and explore their integration into broader security frameworks, the implications for future innovations are vast. By prioritizing collaboration across disciplines—combining machine learning with threat intelligence—the industry stands poised to develop more effective strategies that not only defend against current threats but also anticipate future challenges in this dynamic digital landscape.
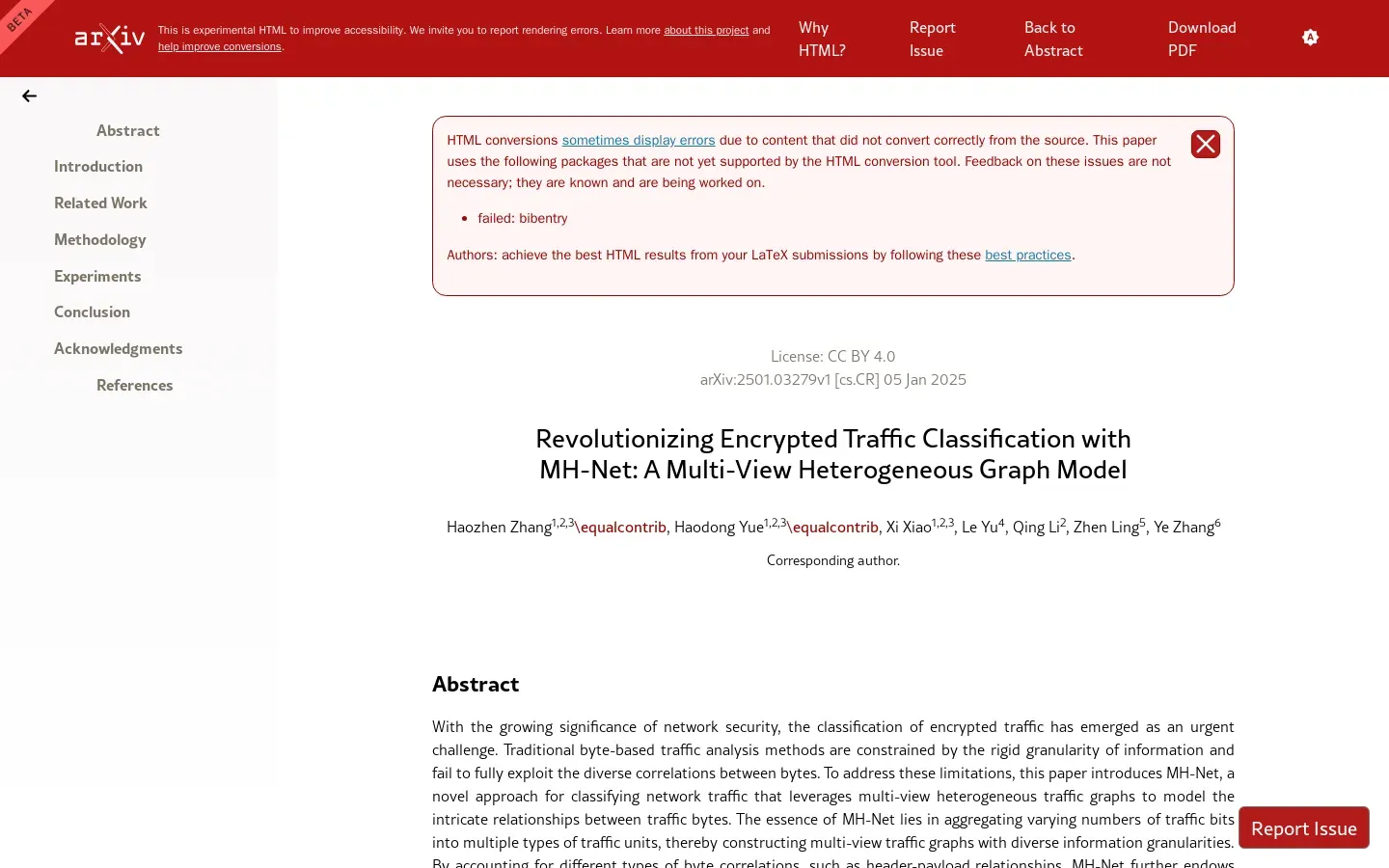