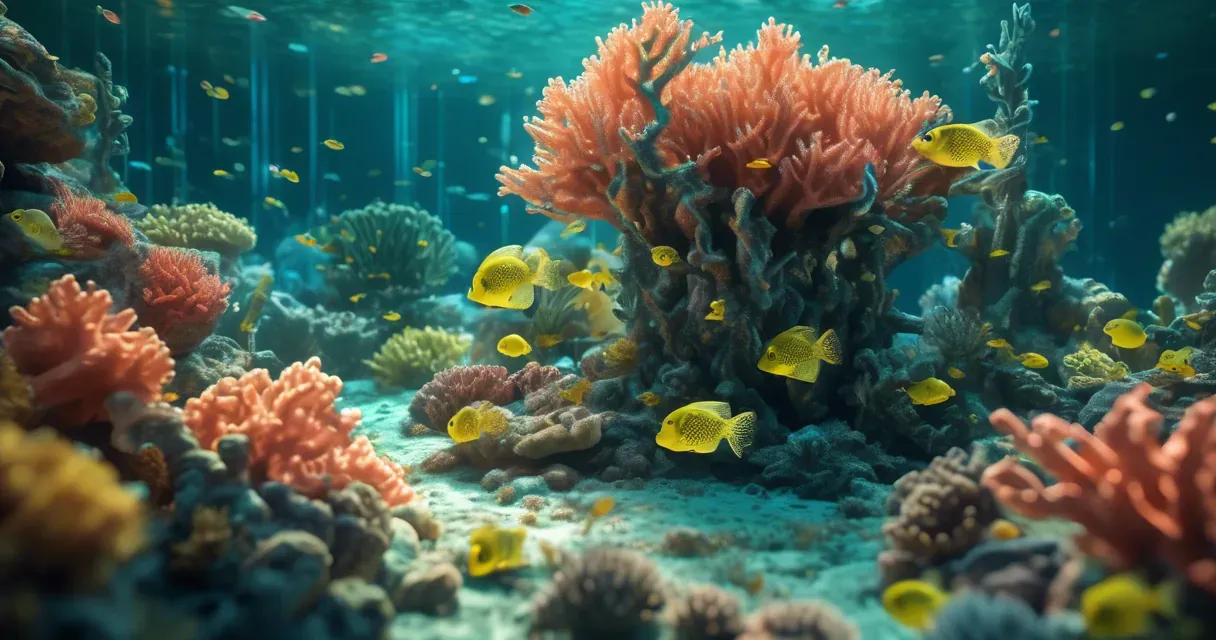
Power Side-Channel Attacks in Embedded Systems Security Explored
/ 4 min read
Quick take - The article discusses a comprehensive study on advancements in real-time intrusion detection systems (IDS) for enhancing cybersecurity, particularly in Internet of Things (IoT) environments, highlighting innovative methodologies, key findings, and future research directions to address evolving cyber threats.
Fast Facts
- The study focuses on enhancing real-time intrusion detection systems (IDS) for improved cybersecurity, particularly in Internet of Things (IoT) environments.
- Researchers developed a novel fingerprinting algorithm using wavelet transforms, which enhances signal processing and noise management in power side-channel attacks.
- Key methodologies included dynamic window matching, wavelet transform for control flow fingerprinting, and automated fuzzing tools for testing security protocols.
- The research highlights the limitations of machine learning in black-box scenarios and emphasizes the need for cross-architecture generalization in security measures.
- Future directions include developing tailored security protocols for IoT devices and exploring real-time fuzzing techniques and advanced neural networks to strengthen IDS effectiveness.
In the ever-evolving landscape of cybersecurity, where threats become increasingly sophisticated and pervasive, researchers are tirelessly seeking innovative solutions to bolster defenses against a myriad of attack vectors. With the proliferation of Internet of Things (IoT) devices, the necessity for enhanced security protocols has never been more pressing. A recent study dives deep into this realm, exploring methodologies such as wavelet transforms and dynamic window matching to improve intrusion detection systems (IDS) while addressing critical challenges faced in real-world applications.
At the forefront of this research is an investigation into FPGA-based security solutions which promises to revolutionize how we approach system vulnerabilities. By utilizing signal processing algorithms, particularly through techniques like high-pass filtering and noise management, researchers aim to refine the process of identifying anomalies in power consumption patterns—an essential component for detecting side-channel attacks. This work emphasizes the need for real-time application viability, ensuring that these advanced detection systems can operate effectively in dynamic environments.
The study employs an experimental setup that leverages tools like ChipWhisperer (CW) and oscilloscopes to gather data on power traces under various conditions. This meticulous collection of information serves as a foundation for implementing cross-device security profiling, allowing researchers to develop a fingerprinting algorithm capable of identifying unique operational characteristics across different devices. This aspect highlights a significant advancement: the cross-architecture generalization of security measures, enabling them to adapt across various hardware platforms seamlessly.
Yet, with these advancements come notable limitations and areas ripe for further exploration. The reliance on single execution analysis poses risks; variations in device performance can obscure detection capabilities. Additionally, while machine learning techniques like convolutional neural networks (CNNs) offer robust data processing potential, their effectiveness may wane in black-box scenarios where transparency is limited. Thus, there’s an urgent call for reevaluation of assumptions within existing security models to ensure they can withstand the rigors of practical application.
Among the key findings from this research is the realization that automated fuzzing tools for embedded systems could significantly enhance resilience against emerging threats. Such tools would allow for continuous testing and adaptation of security protocols, identifying weaknesses before they are exploited by malicious actors. The implications extend beyond mere detection; they encompass comprehensive strategies that integrate noise characterization and analysis to mitigate risks associated with power side-channel attacks.
As we look toward the future, the potential applications stemming from this research are vast. The integration of advanced signal-processing algorithms with traditional IDS can lead to a new era in cybersecurity where real-time threat detection becomes not just feasible but reliable. Moreover, there’s a growing necessity for improved security measures specifically tailored for IoT devices—an area that remains underrepresented in current literature yet is critical given the rapid adoption of smart technologies.
In conclusion, as organizations continue to grapple with cybersecurity challenges, leveraging wavelet-based fingerprinting methods alongside robust intrusion detection frameworks could provide a much-needed advantage. The blend of innovative signal processing techniques and machine learning approaches signifies a crucial step forward in developing more resilient systems capable of thwarting both existing and emerging threats. As researchers refine these methodologies and address their inherent limitations, we stand on the brink of a transformation in how we perceive and tackle cybersecurity challenges—a transformation that promises not only to protect our devices but also to safeguard our increasingly interconnected world.