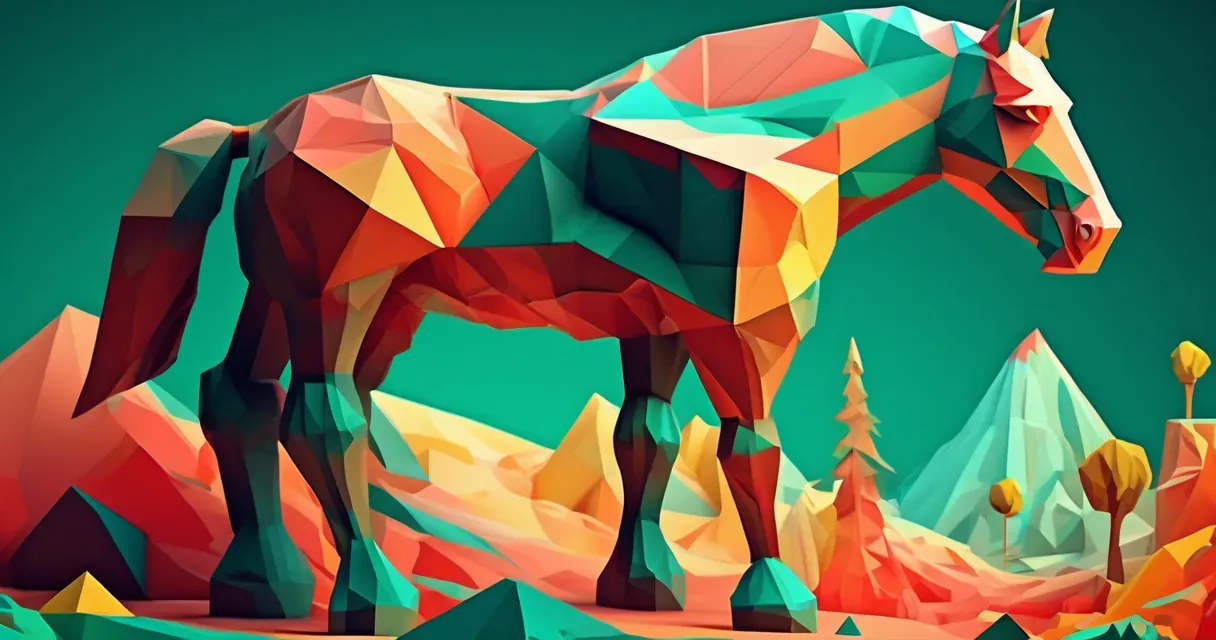
TrojanDec Framework Enhances Detection of Trojan Inputs
/ 4 min read
Quick take - Recent research has introduced the TrojanDec framework, which aims to enhance cybersecurity by providing a defense mechanism against trojan attacks in self-supervised learning and reinforcement learning contexts, demonstrating improved accuracy in downstream classifiers and the potential for real-time threat detection integration.
Fast Facts
- TrojanDec Framework: A new defense mechanism developed to combat trojan attacks in self-supervised learning and reinforcement learning contexts, enhancing the robustness of machine learning models.
- Methodology: Utilizes advanced techniques like the Denoising Diffusion Null-Space Model (DDNM) for image restoration, along with Mask Set Generation and K-means Clustering for improved detection.
- Key Findings: The framework significantly boosts the accuracy of downstream classifiers and reduces the attack success rate on trojaned images, with effective image restoration being crucial for detection accuracy.
- Practical Implications: Highlights the need for robust cybersecurity measures in AI-driven environments and suggests integration with real-time threat detection systems for immediate response to cyber threats.
- Future Directions: Emphasizes the importance of optimizing TrojanDec for low-latency applications and exploring domain-specific trojan attacks, alongside user education to strengthen overall cybersecurity defenses.
In a world where the digital landscape is increasingly fraught with threats, cybersecurity has become an imperative field of research and implementation. With the rise of artificial intelligence and machine learning, traditional security measures have struggled to keep pace, particularly in defending against sophisticated threats like trojan attacks. The TrojanDec framework emerges as a pivotal advancement in this ongoing battle, specifically designed to bolster self-supervised learning models against such insidious incursions. By integrating cutting-edge methodologies and tools such as Denoising Diffusion Null-Space Models (DDNM) and adversarial training, researchers are laying the groundwork for robust defenses that can adapt to the evolving nature of cyber threats.
Real-time Monitoring and Response Systems play a crucial role in this framework, enabling immediate detection and mitigation of potential risks. The ability to respond promptly is not just a luxury; it’s a necessity in an era where delays could result in significant data breaches or system compromises. Building on this capability, the research also emphasizes the importance of evaluation and performance metrics to assess the effectiveness of these systems continually. By analyzing accuracy (ACC) and attack success rates (ASR), stakeholders can ensure that their defenses are not only effective but also resilient under varied conditions.
While the advancements presented by TrojanDec are promising, there remain limitations that call for further investigation. A core area of focus is mask generation and sampling, which plays a vital role in enhancing detection mechanisms. Additionally, the integration of cross-domain transfer learning can help improve the response capabilities of these systems across different operational contexts. This adaptability is essential, especially as attackers become more sophisticated in their tactics.
The implications for cybersecurity are profound. The TrojanDec framework offers insights not only into practical defense mechanisms but also into theoretical paradigms that underpin these technologies. For instance, integrating federated learning can enhance collaborative defense strategies among various entities without compromising sensitive data. This approach reinforces collective security while addressing individual privacy concerns—a balancing act that is increasingly vital in today’s data-driven world.
As researchers continue to explore the strengths of TrojanDec, they highlight its potential applications beyond reinforcement learning environments. Incorporating self-supervised learning (SSL) techniques allows for enhanced encoder pre-training, effectively improving model robustness against adversarial attacks. Furthermore, implementing collaborative defense mechanisms could foster a community-oriented approach to cybersecurity, where knowledge sharing leads to stronger collective defenses.
Looking ahead, several future directions merit attention. Optimizing TrojanDec for low-latency environments will be critical as industries demand real-time responses without sacrificing performance quality. Exploring domain-specific adaptations will ensure that defenses remain relevant across varied applications—be it healthcare, finance, or critical infrastructure.
Moreover, user education and awareness programs cannot be overlooked. As organizations implement advanced frameworks like TrojanDec, equipping users with knowledge about potential threats and best practices will amplify these technological advancements’ effectiveness.
In conclusion, as we navigate an increasingly AI-driven world fraught with cyber threats, the findings from research on frameworks like TrojanDec serve as both a beacon of hope and a call to action. The future of cybersecurity lies not only in developing innovative technologies but also in fostering an ecosystem where collaboration and education empower all stakeholders to defend against emerging threats effectively. Embracing this holistic approach could very well define how we safeguard our digital futures.