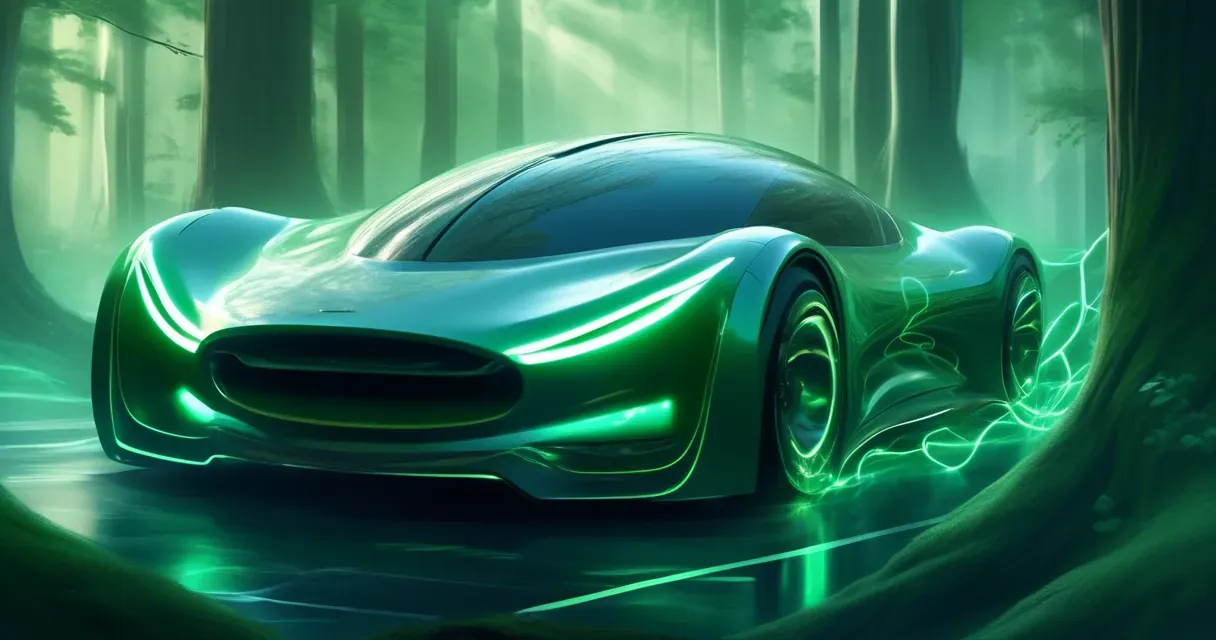
Autonomous Vehicles Vulnerable to Electromagnetic Signal Attacks
/ 4 min read
Quick take - Recent research by Guesmi et al. (2023) highlights the vulnerabilities of autonomous vehicles to Electromagnetic Signal Injection Attacks and emphasizes the need for robust detection mechanisms and multi-sensor fusion to enhance the security of AI perception systems.
Fast Facts
- Vulnerability to Attacks: Autonomous vehicles are susceptible to Electromagnetic Signal Injection Attacks (ESIA), which can manipulate sensor data and lead to catastrophic failures.
- Research Objectives: The study aimed to enhance the resilience of AI perception systems against ESIA and other adversarial threats, highlighting the need for robust detection mechanisms.
- Simulated Attack Dataset: A key outcome was the creation of a simulated attack dataset to evaluate AI model performance under ESIA conditions, aiding in the development of more secure AI models.
- Multi-Sensor Fusion: The research emphasized integrating data from various sensors (cameras, LiDAR, radar) to improve the security and reliability of autonomous systems.
- Future Directions: The study calls for stronger threat models, interdisciplinary collaboration, and the incorporation of cybersecurity into the design and implementation of autonomous vehicles.
In an era where autonomous vehicles are becoming more prevalent, the intersection of cybersecurity and artificial intelligence has never been more critical. Recent advancements have illuminated vulnerabilities within these systems, notably through the lens of Electromagnetic Signal Injection Attacks (ESIA). As researchers delve deeper into these threats, the implications stretch far beyond theoretical discussions; they weave into the very fabric of our daily interactions with technology, prompting a profound reconsideration of safety protocols and defensive strategies.
The research conducted by Guesmi et al. (2023) spotlights the importance of enhanced threat modeling for autonomous systems. This approach not only identifies potential vulnerabilities but also emphasizes the necessity for robust detection mechanisms to counteract emerging threats like ESIA. These attacks exploit weaknesses in AI perception systems, jeopardizing both safety and reliability. By understanding the mechanics behind such adversarial attacks, researchers can better prepare defenses that are as sophisticated as the threats themselves.
One of the standout contributions from this research is the development of a novel ESIA simulation method, which paves the way for a deeper understanding of how these attacks manifest in real-world scenarios. This methodology allows for systematic evaluations of AI model performance under duress, ultimately leading to robust AI models that better withstand malicious attempts to manipulate sensor data. Through this lens, multi-sensor fusion emerges as a critical technique, integrating data from various sources such as cameras, LiDAR, and radar to bolster security against sophisticated attacks.
Yet while these innovations are promising, limitations remain that necessitate further investigation. The need for real-time monitoring and response mechanisms cannot be overstated; as autonomous vehicles traverse unpredictable environments, their ability to respond dynamically to potential threats will determine their success or failure in practical applications. Furthermore, enhanced security protocols tailored specifically for autonomous vehicles are essential to safeguard against evolving attack vectors.
The research also highlights a significant area of focus: cross-disciplinary collaboration. By pooling knowledge across fields—cybersecurity experts working alongside automotive engineers—the industry can establish a more resilient framework for addressing vulnerabilities posed by ESIA. This collaborative spirit could lead to breakthroughs in policy and regulatory implications, ensuring that standards evolve in tandem with technological advancements.
In terms of practical tools discussed, frameworks like Mask R-CNN and Grad-CAM serve crucial roles in enhancing detection capabilities within smart surveillance systems. These techniques help visualize areas of focus in neural networks, offering insights into potential weaknesses that adversaries might exploit. As we continue to refine these models, real-time adjustments based on simulated attack datasets will become increasingly vital.
Looking ahead, the future implications of this research are compelling. As autonomous vehicles integrate more deeply into urban infrastructures, their resilience against adversarial attacks will be paramount. Ensuring that AI perception systems are fortified against ESIA not only enhances individual vehicle safety but also contributes to broader societal trust in autonomous technologies. Researchers and practitioners must collectively prioritize these developments to create safer technological environments that can withstand the complexities of modern threats.
As we stand at this crossroads between innovation and vulnerability, it becomes clear: safeguarding the future of autonomous vehicles requires an unwavering commitment to research and collaboration across disciplines. Only then can we ensure a secure path forward in our journey towards a fully autonomous world.