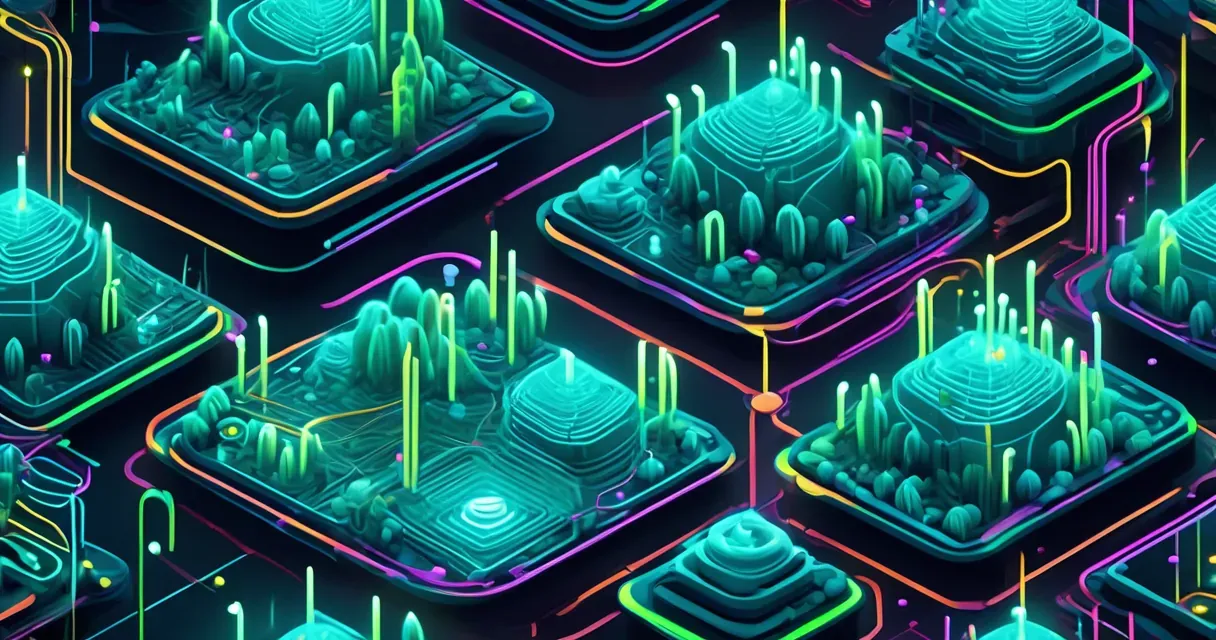
Decentralized Anomaly Detection Advances in Smart Grids
/ 4 min read
Quick take - Recent research highlights the application of Decentralized Federated Learning (DFL) to improve anomaly detection in smart grids, addressing cybersecurity vulnerabilities and suggesting potential advancements in various sectors through enhanced collaboration and real-time data processing.
Fast Facts
- Innovative Approach: The study explores Decentralized Federated Learning (DFL) to improve anomaly detection in smart grids, addressing cybersecurity vulnerabilities in Industrial Control Systems (ICS).
- Methodology: Utilized a Transformer Autoencoder (TAE) for anomaly detection, along with gradient quantization for communication efficiency, and techniques like PCA and KNN for data integrity.
- Key Findings: DFL combined with gossip-based protocols enhances anomaly detection and reduces computational overhead, improving resilience against cyberattacks in power systems.
- Broader Implications: The research highlights DFL’s potential in enhancing cybersecurity across various sectors, including healthcare IoT and urban infrastructure, through real-time data processing.
- Future Directions: Acknowledges limitations in scalability and suggests further exploration of cross-domain applications and enhanced collaboration frameworks to strengthen cybersecurity efforts.
In an age where smart cities are becoming a reality, the integration of advanced technologies into urban infrastructure is transforming our lives. Yet, with this transformation comes an imperative to safeguard these interconnected systems against increasingly sophisticated cyber threats. Enter Decentralized Federated Learning (DFL)—a cutting-edge approach that holds promise for real-time anomaly detection in critical infrastructures like power systems. As researchers delve deeper into this innovative framework, they unveil a treasure trove of methodologies and findings that not only enhance cybersecurity but also reshape our understanding of data privacy and collaboration.
At the heart of this research lies the Transformer-Autoencoder (TAE), a sophisticated mechanism designed for anomaly detection that leverages the strengths of deep learning architectures. By employing feature selection and dimensionality reduction techniques such as Principal Component Analysis (PCA) and K-Nearest Neighbor (KNN) imputation, the TAE efficiently identifies abnormalities in data streams while preserving essential information. The use of normalization further ensures that input features are standardized, promoting better model performance. These methodologies are particularly pertinent in environments like smart grids, where vast amounts of data from various sources must be processed in real-time to detect potential security breaches.
One standout aspect of this research is the gossip-based decentralized federated learning, which introduces a collaborative framework allowing multiple stakeholders to share insights without revealing sensitive data. This approach not only enhances resilience against cyberattacks but also employs a novel sign-based gradient quantization scheme to optimize communication efficiency within DFL frameworks. By minimizing the computational overhead typically associated with centralized models, DFL emerges as a frontrunner for tackling the unique challenges posed by industrial control systems (ICS) security.
The implications of these findings extend beyond technical improvements; they herald a paradigm shift in how we conceptualize cybersecurity in interconnected environments. For instance, the ability to implement robust anomaly detection systems can significantly enhance the operational integrity of smart grids while addressing privacy concerns inherent in traditional centralized systems. Furthermore, the integration of edge computing allows for localized processing, further bolstering security measures.
While the research showcases numerous strengths—such as cross-organizational collaboration and innovative protocol designs—it does not shy away from acknowledging limitations. There remains a need for more extensive testing under diverse operational conditions to validate the robustness of these methodologies. Additionally, questions about scalability and real-world applicability linger, urging further investigation into how DFL can be seamlessly integrated across various sectors including healthcare IoT security.
As we look toward future directions, the potential applications for decentralized federated learning are expansive. From enhancing smart city infrastructures to developing resilient healthcare systems capable of defending against cyber threats, the possibilities seem limitless. Researchers are encouraged to explore cross-domain applications that leverage DFL’s capacity for secure data sharing and improved anomaly detection mechanisms.
In summary, as we stand on the brink of an interconnected future, it is crucial to harness technologies like decentralized federated learning to safeguard our digital landscape. By combining innovation with collaborative approaches, we can pave the way for smarter, safer cities that not only prioritize efficiency but also uphold the highest standards of cybersecurity. The road ahead is filled with opportunities for advancement; with continued research and collaboration, we may soon see these ideas transform into practical solutions that redefine resilience in our increasingly complex world.